A fast algorithm for nonsmooth penalized clustering.
Neurocomputing(2018)
摘要
As a novel framework of clustering analysis, penalized clustering is able to learn the number of clusters automatically, and therefore has aroused widespread interest recently. To address the computational difficulties arising from the nonsmoothness of the penalty, a simple iterative algorithm based on smoothing trust region (STR) can be used. However, since STR only needs first-order information of the model, it might exhibit slow convergence rate sometimes. To accelerate STR and further improve the efficiency of penalized clustering, we propose a nonmonotone smoothing trust region (NSTR) algorithm, in which nonmonotone technique and the Barzilai and Borwein (BB) method are utilized together. We also prove that the new algorithm is globally convergent and estimate its worst case computational complexity. Experimental results on both simulated and real-life data sets validate the effectiveness and efficiency of the proposed method.
更多查看译文
关键词
BB method,Nonmonotone technique,Smoothing trust region,Nonsmooth penalized clustering
AI 理解论文
溯源树
样例
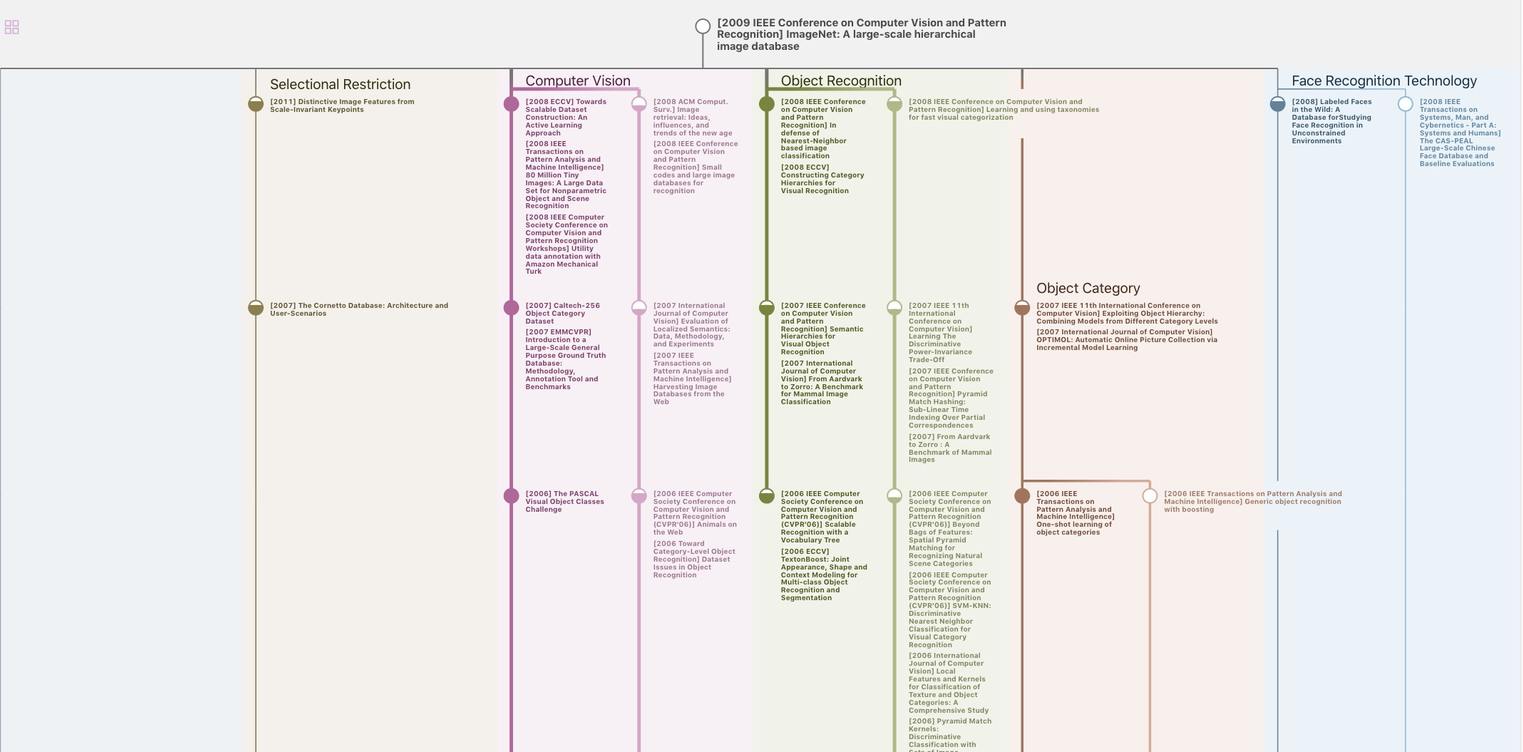
生成溯源树,研究论文发展脉络
Chat Paper
正在生成论文摘要