Unsupervised Feature Selection via Local Structure Learning and Self-Representation
2017 IEEE International Conference on Big Knowledge (ICBK)(2017)
摘要
At present, most of the manifold learning methods use the kNN criterion to build the neighborhood graph. This kind of method results in two disadvantage: 1) These methods ignore the adverse effects of noise and redundancy, 2) The neighborhood graph is a fixed graph, which makes bad effects to the adaptability of the model. In order to handle these problems, this paper proposes a new unsupervised feature selection algorithm based on feature self-representation. Firstly, the similarity matrix of the data is catched according to the similarity between the samples, and the transformation matrix of the data is dynamically adjusted by the similarity matrix until it reaches the best state. Then, the low rank representation is applied to the transformation matrix to consider the global structure of the data. After that, the self-representation of the feature is used to counteract the adverse effect of the outliers on the manifold learning. At last, combining sparse learning to conduct feature selection. The experimental results on real datasets showed that the proposed method outperformed for state-of-the-art methods.
更多查看译文
关键词
feature selection,structure learning,feature self-representation
AI 理解论文
溯源树
样例
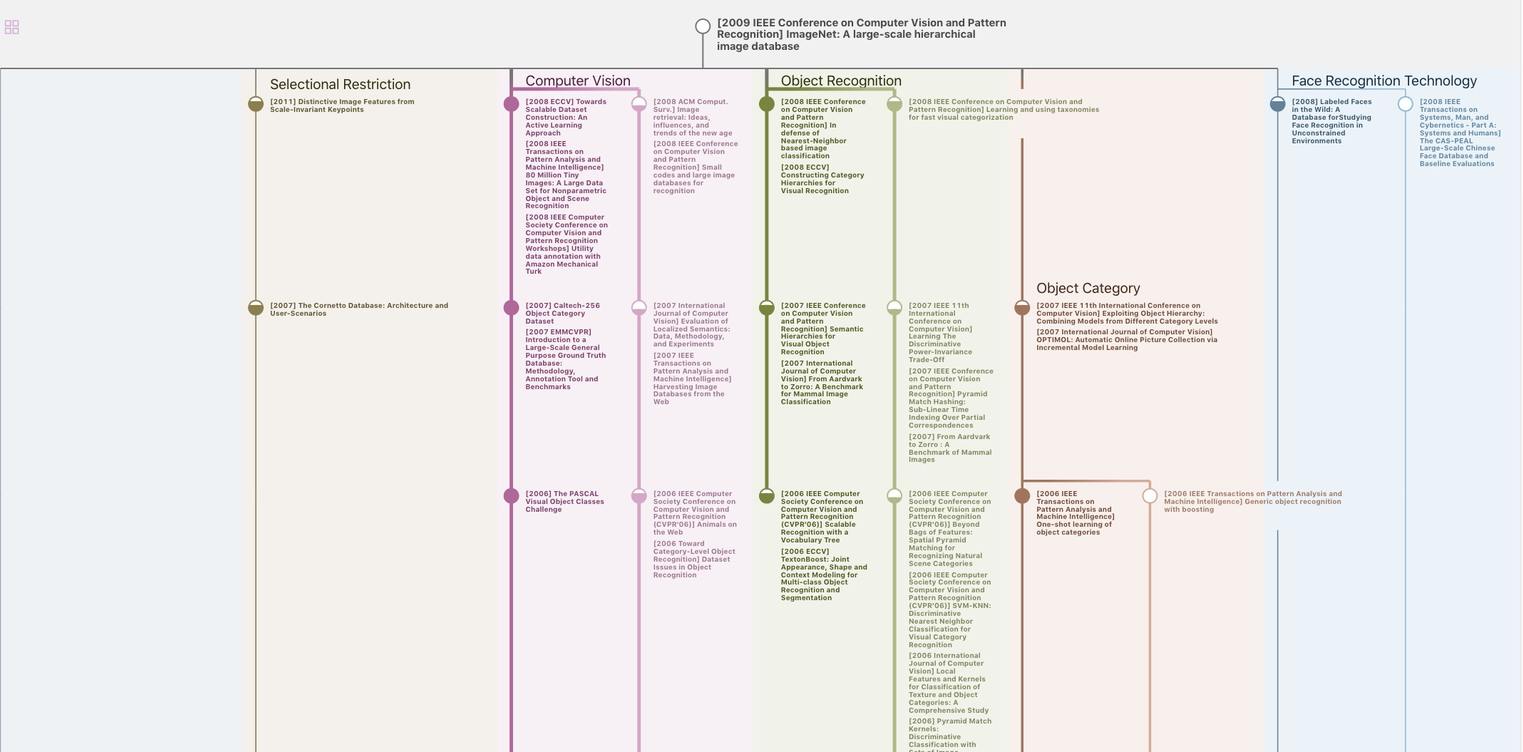
生成溯源树,研究论文发展脉络
Chat Paper
正在生成论文摘要