Super Learning For Anomaly Detection In Cellular Networks
2017 IEEE 13TH INTERNATIONAL CONFERENCE ON WIRELESS AND MOBILE COMPUTING, NETWORKING AND COMMUNICATIONS (WIMOB)(2017)
摘要
The ever-growing population of smartphones connected to mobile networks is changing the cellular traffic ecosystem. The traffic volumes and patterns generated by smartphone apps pose complex challenges to cellular network operators, particularly in terms of detection and diagnosis of network anomalies caused by specific apps. The high-dimensionality of network data provided by current network monitoring systems opens the door to the application of machine learning approaches to improve the detection and classification of network anomalies, but this higher dimensionality comes with an extra data processing overhead. In addition, critical network monitoring applications such as the detection of anomalies require fast mechanisms for on-line analysis of thousands of events per second, as well as efficient techniques for off-line analysis of massive historical data. In this paper we explore the application of big data analytics and big data platforms to the automatic detection of anomalies in mobile networks. We consider ensemble, multi-combined machine learning models to enhance anomaly detection, following a particularly promising model known as Super Learning. Super-learning is an ensemble learning approach which performs asymptotically as well as the best possible weighted combination of multiple learning algorithms, providing a very powerful detection approach. We implement and test different super-learning models on top of Big-DAMA, a big data analytics framework for network monitoring. We test the proposed solution on the detection of traffic anomalies in operational cellular networks, and compare it to other traditional ensemble learning approaches such as bagging and boosting. Results indicate that our approach outperforms traditional ones.
更多查看译文
关键词
Big-Data, Network Traffic Monitoring and Analysis, Machine Learning, Ensemble Learning, Super Learning, Service Anomalies
AI 理解论文
溯源树
样例
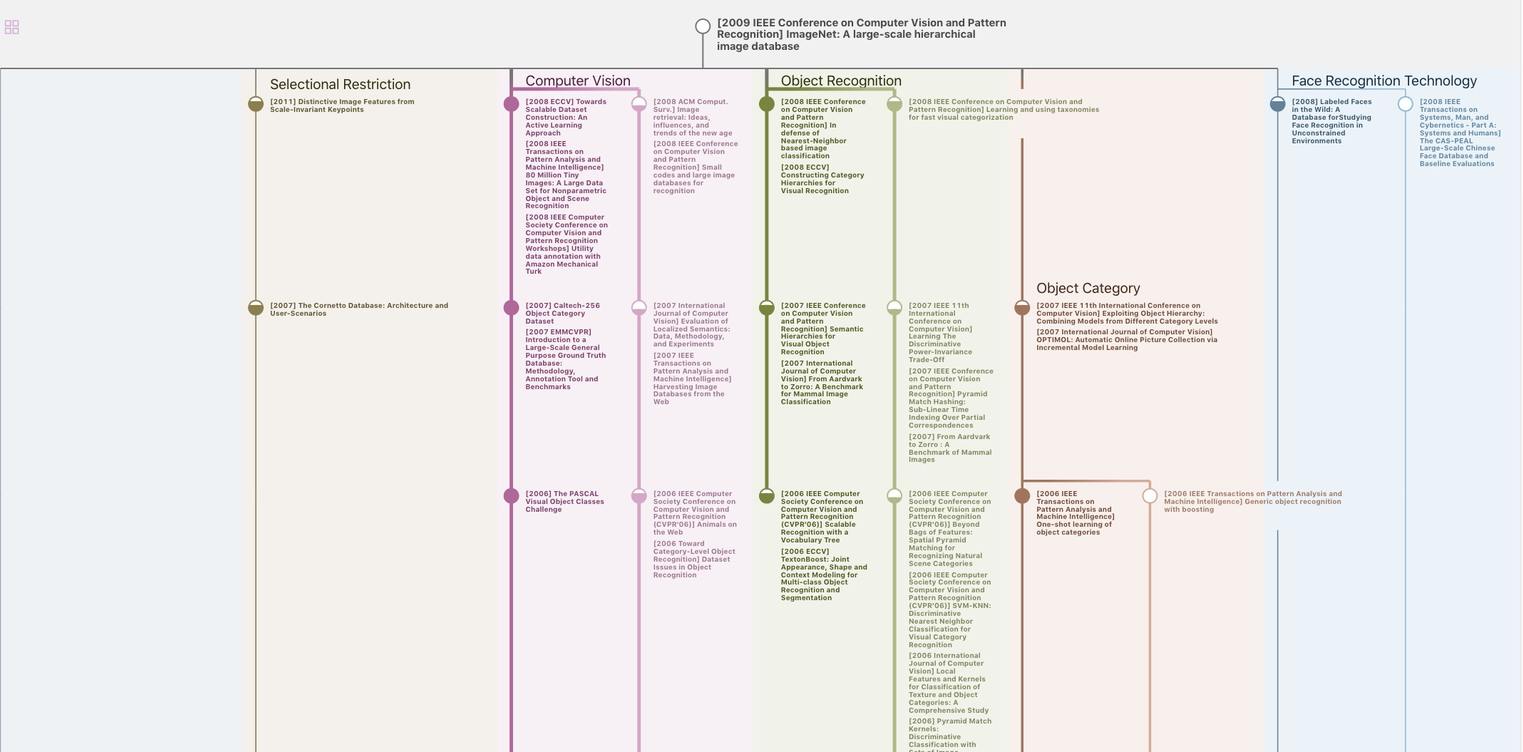
生成溯源树,研究论文发展脉络
Chat Paper
正在生成论文摘要