Combining deep learning and language modeling for segmentation-free OCR from raw pixels
2017 1st International Workshop on Arabic Script Analysis and Recognition (ASAR)(2017)
摘要
We present a simple yet effective LSTM-based approach for recognizing machine-print text from raw pixels. We use a fully-connected feed-forward neural network for feature extraction over a sliding window, the output of which is directly fed into a stacked bi-directional LSTM. We train the network using the CTC objective function and use a WFST language model during recognition. Experimental results show that this simple system outperforms extensively tuned state-of-the-art HMM models on the DARPA Arabic Machine Print corpus.
更多查看译文
关键词
DARPA Arabic Machine Print corpus,deep learning,language modeling,segmentation-free OCR,raw pixels,machine-print text,feed-forward neural network,feature extraction,sliding window,stacked bi-directional LSTM,CTC objective function,WFST language model,simple system,HMM models,LSTM-based approach
AI 理解论文
溯源树
样例
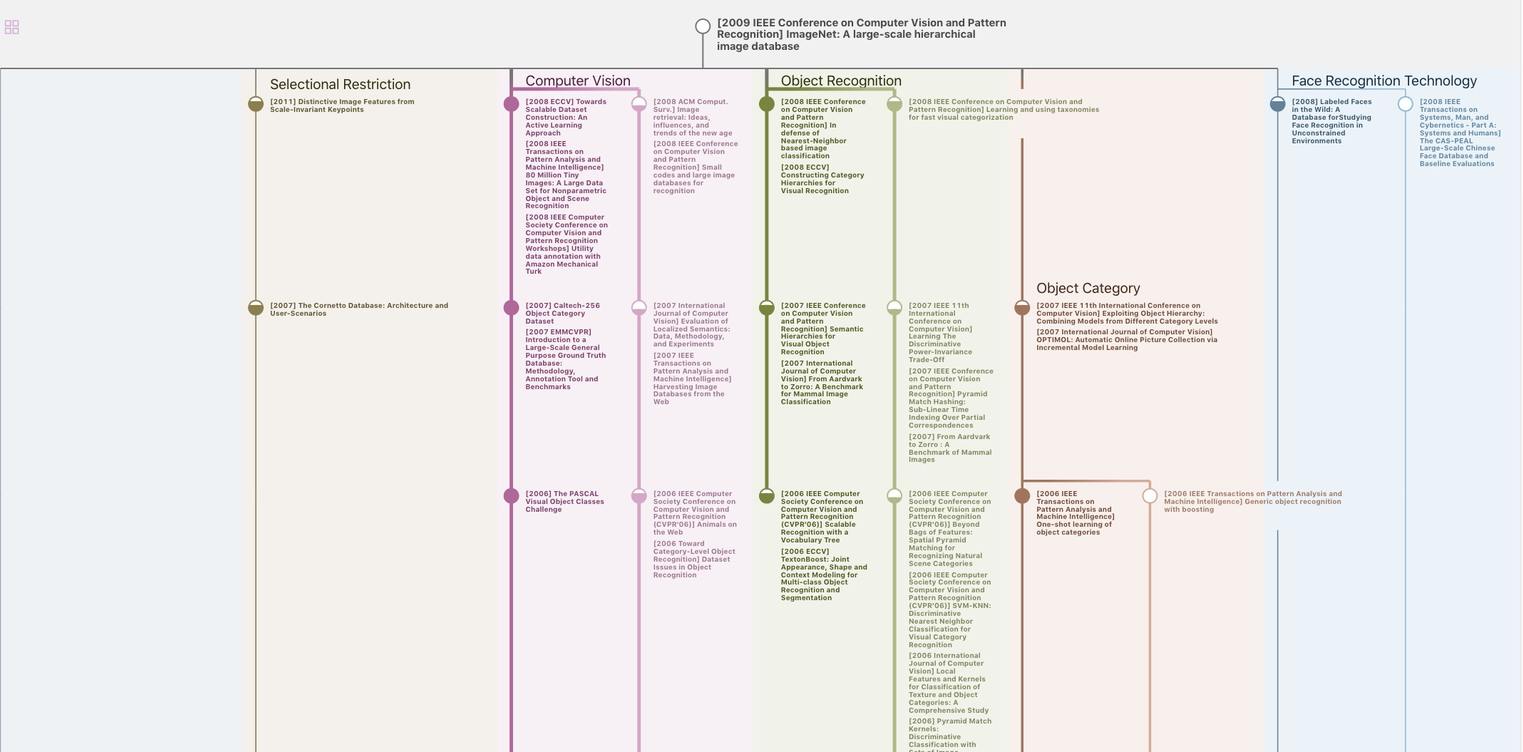
生成溯源树,研究论文发展脉络
Chat Paper
正在生成论文摘要