Learning Convolutional Ranking-Score Function By Query Preference Regularization
INTELLIGENT DATA ENGINEERING AND AUTOMATED LEARNING - IDEAL 2017(2017)
摘要
Ranking score plays an important role in the system of content-based retrieval. Given a query, the database items are ranked according to the ranking scores in a descending order, and the top-ranked items are returned as retrieval results. In this paper, we propose a new ranking scoring function based on the convolutional neural network (CNN). The ranking scoring function has a structure of CNN, and its parameters are adjusted to both queries and query preferences. The learning process guarantees that the ranking score of the query itself is large, and also the ranking scores of the positives (database items which the query wants to link) are larger than those of the negatives (database items which the query wants to avoid). Moreover, we also impose that the neighboring database items have similar ranking scores. An optimization problem is formulated and solved by Estimation-Maximization method. Experiments over the benchmark data sets show the advantage over the existing learning-to-rank methods.
更多查看译文
关键词
Convolutional neural network, Content-based retrieval, Ranking score, Estimation-maximization method
AI 理解论文
溯源树
样例
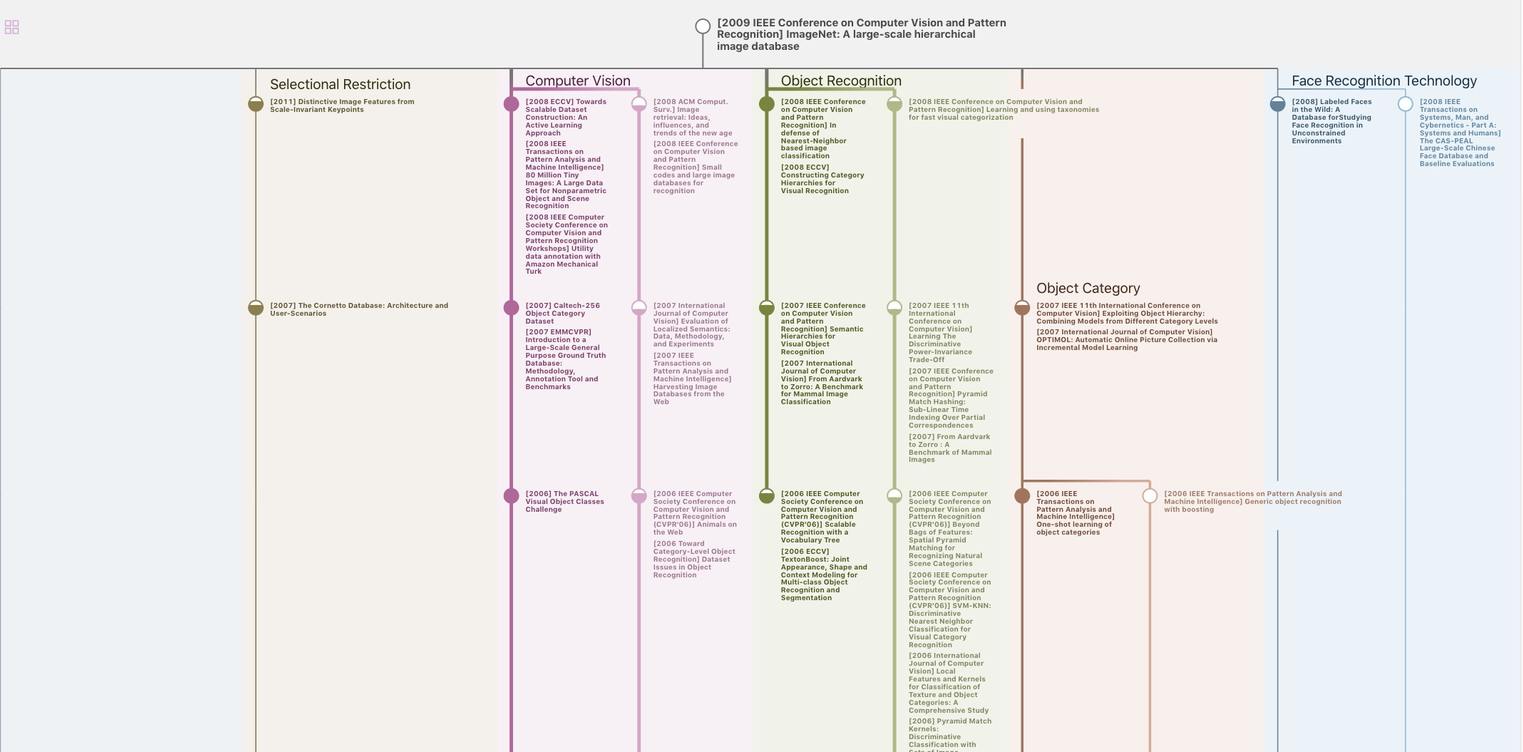
生成溯源树,研究论文发展脉络
Chat Paper
正在生成论文摘要