ReForeSt: Random Forests in Apache Spark
ARTIFICIAL NEURAL NETWORKS AND MACHINE LEARNING, PT II(2017)
摘要
Random Forests (RF) of tree classifiers are a popular ensemble method for classification. RF are usually preferred with respect to other classification techniques because of their limited hyperparameter sensitivity, high numerical robustness, native capacity of dealing with numerical and categorical features, and effectiveness in many real world classification problems. In this work we present ReForeSt, a Random Forests Apache Spark implementation which is easier to tune, faster, and less memory consuming with respect to MLlib, the de facto standard Apache Spark machine learning library. We perform an extensive comparison between ReForeSt and MLlib by taking advantage of the Google Cloud Platform (https://cloud.google.com). In particular, we test ReForeSt and MLlib with different library settings, on different real world datasets, and with a different number of machines equipped with different number of cores. Results confirm that ReForeSt outperforms MLlib in all the above mentioned aspects. ReForeSt is made publicly available via GitHub (https://github.com/alessandrolulli/reforest).
更多查看译文
关键词
Random Forests,Apache Spark,Open source software
AI 理解论文
溯源树
样例
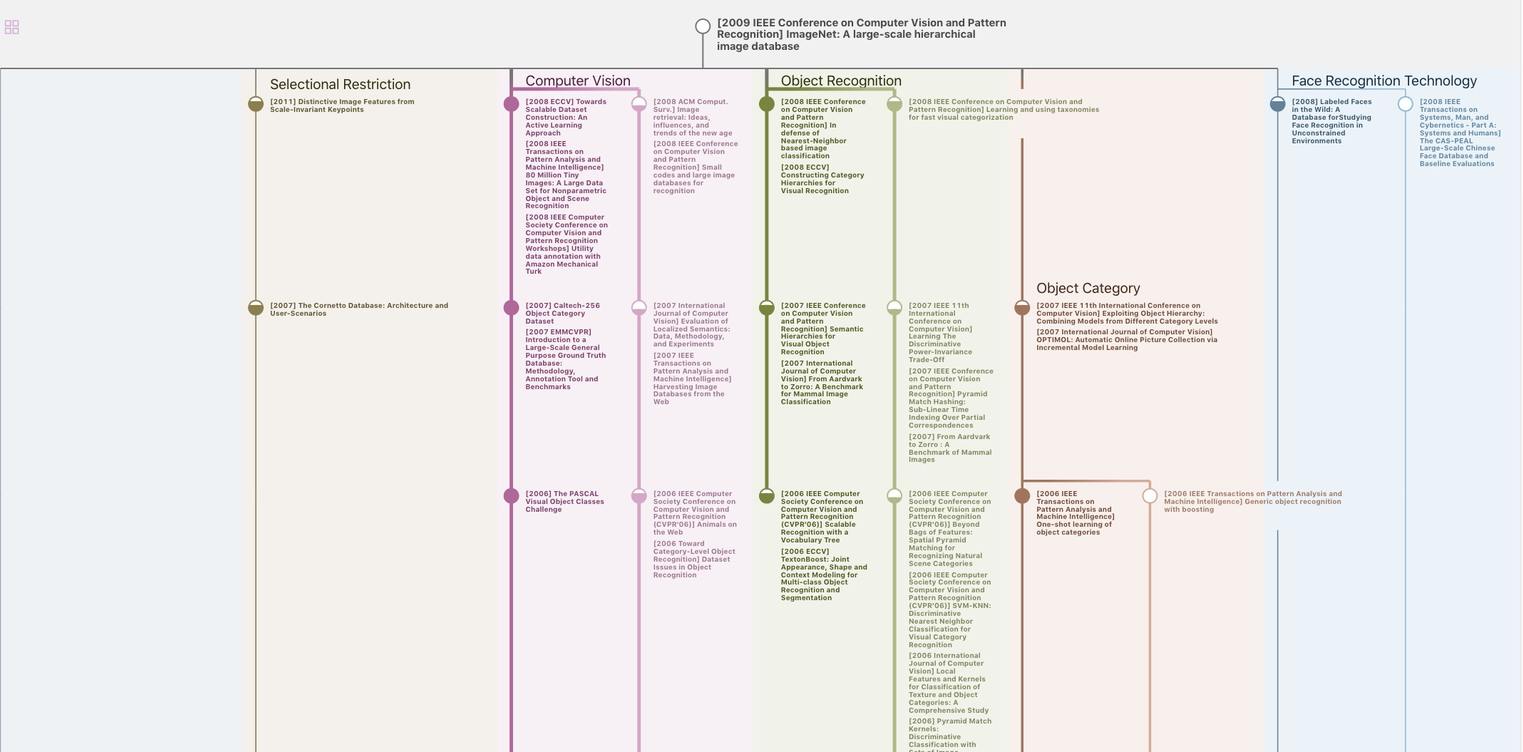
生成溯源树,研究论文发展脉络
Chat Paper
正在生成论文摘要