Atlas-based global and local RF segmentation of head and neck organs on multimodal MRI images
Proceedings of the 10th International Symposium on Image and Signal Processing and Analysis(2017)
摘要
Organ segmentation in the head and neck region is very challenging due to the large variability of the shape and size of organs among patients. Accurate and consistent segmentation of the organ-at-risk (OAR) regions is important in radiation treatment planning. This paper presents a fully automated atlas- and learning-based method for segmenting three OARs (trachea, spinal cord, parotid glands) in multimodal head-and-neck MRI images. The proposed method consists of three main parts. First, a probabilistic atlas is generated. Then, a Random Forest classifier that incorporates the atlas as well as various image features of the multimodal images is applied globally and locally in order to handle local variations. The method was trained and tested on 30 multimodal MRI examinations including T2w, T1w and T1w fat saturated images. Manually defined contours were used as reference. The presented results show good correlation with the reference using DICE similarity measurements. Based on these preliminary results the proposed method can be adapted to other organs of the head-and-neck region.
更多查看译文
关键词
organ-at-risk regions,radiation treatment planning,spinal cord,parotid glands,multimodal head,Random Forest classifier,T1w fat saturated images,atlas-based global RF segmentation,atlas-based local RF segmentation,T2w fat saturated images,head-and-neck region,multimodal MRI image features,learning-based method,DICE similarity measurements
AI 理解论文
溯源树
样例
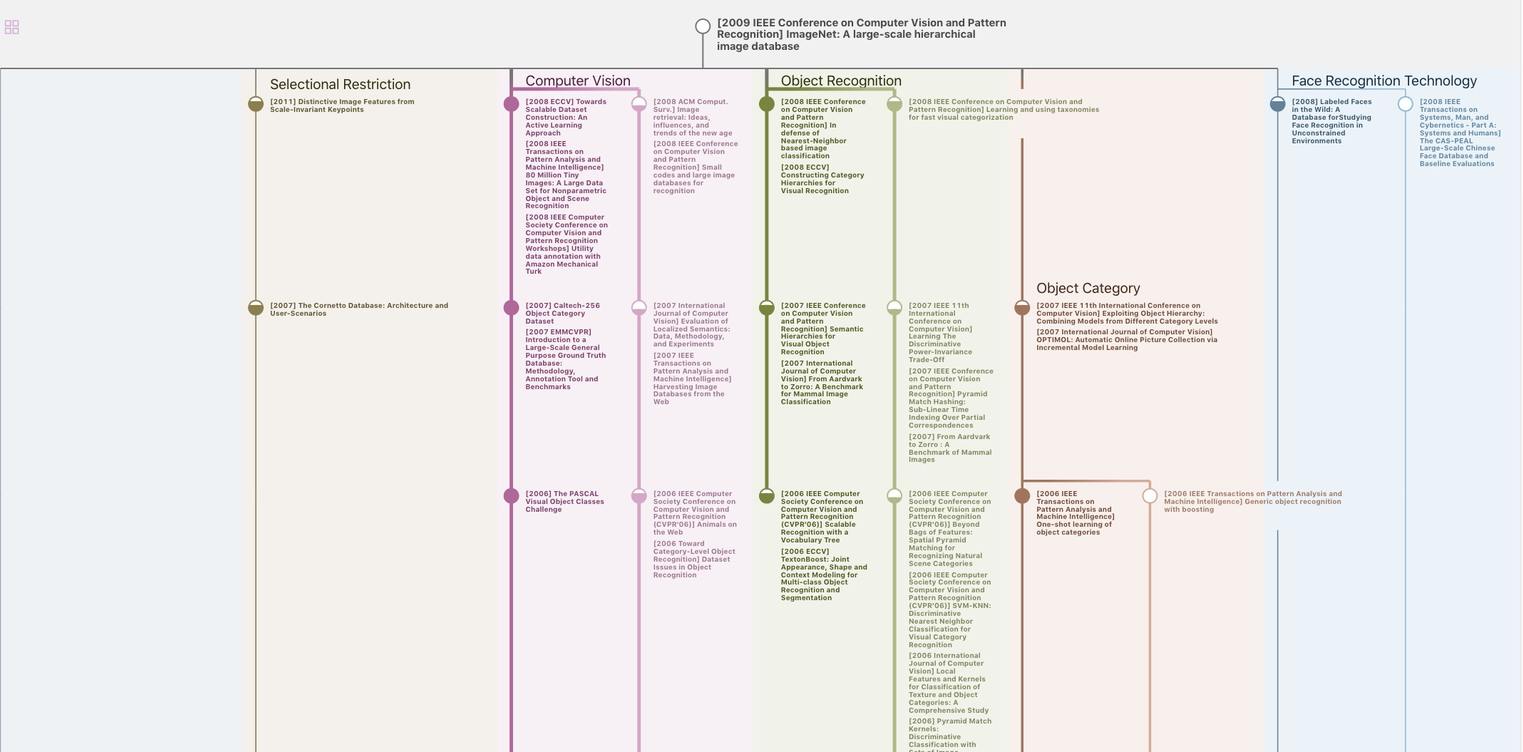
生成溯源树,研究论文发展脉络
Chat Paper
正在生成论文摘要