Tinydl: Just-In-Time Deep Learning Solution For Constrained Embedded Systems
2017 IEEE INTERNATIONAL SYMPOSIUM ON CIRCUITS AND SYSTEMS (ISCAS)(2017)
摘要
This work proposes TinyDL, an automated end-to-end framework that aims to integrate the state-of-the-art Deep Learning (DL) models into embedded systems. TinyDL enables efficient training and execution of DL models as data is collected over time while adhering to the underlying physical resources and constraints. The constraints can be characterized in terms of memory bandwidth, energy resources (e.g., battery life), and/or real-time requirement. TinyDL takes advantage of platform profiling to abstract the physical characteristics of the target embedded device. We introduce a platform-aware signal transformation methodology to enable DL training and execution within the confine of the available resources. Our approach balances the trade-off between data movements and computations to improve the performance of costly iterative DL training/execution. Proof-of-concept implementation on NVIDIA Jetson TK1 embedded platform demonstrates up to two orders of magnitude energy improvement over the previous DL solutions, none of which had been amenable to constrained devices.
更多查看译文
关键词
TinyDL,just-in-time deep learning solution,constrained embedded systems,automated end-to-end framework,platform-aware signal transformation methodology
AI 理解论文
溯源树
样例
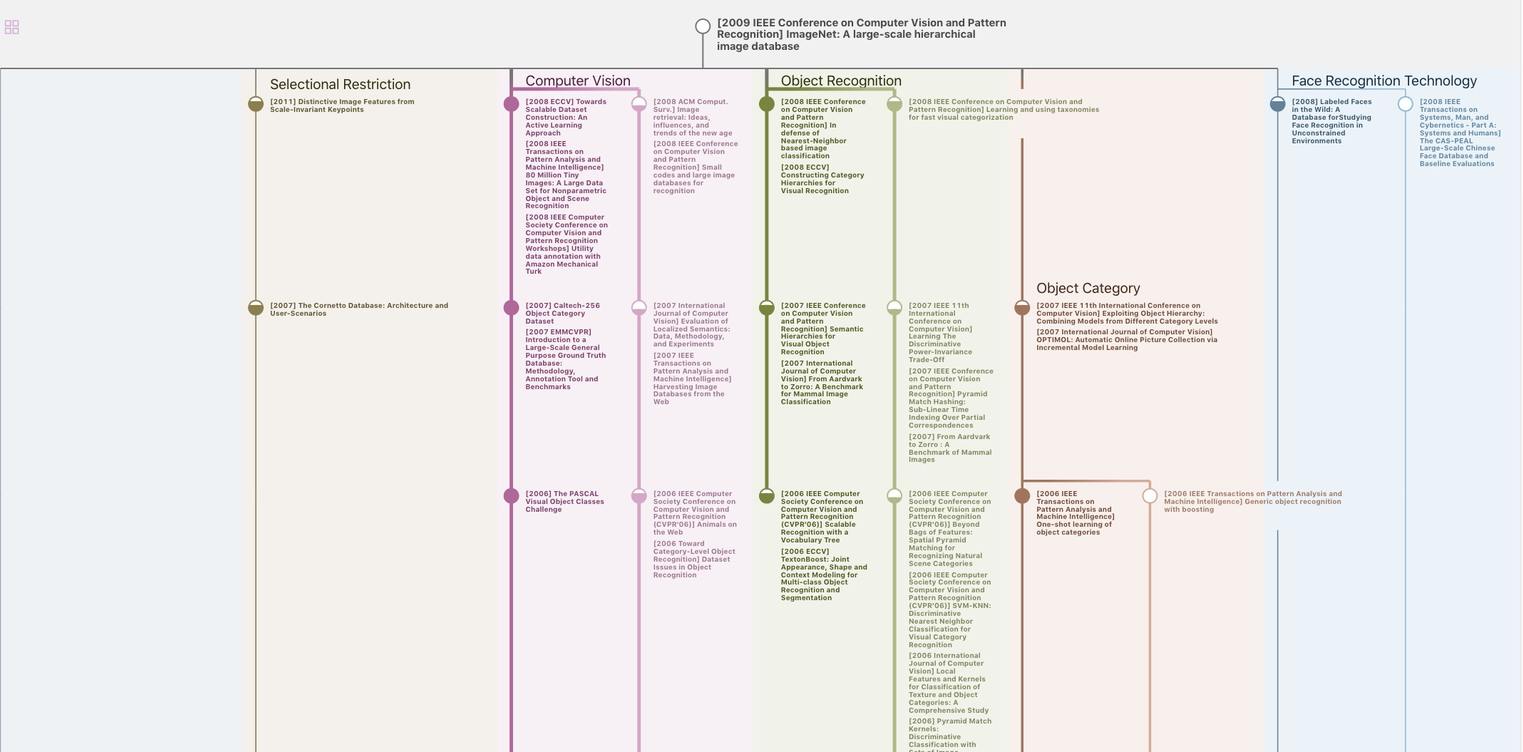
生成溯源树,研究论文发展脉络
Chat Paper
正在生成论文摘要