Regularizing Cnn Via Feature Augmentation
NEURAL INFORMATION PROCESSING (ICONIP 2017), PT II(2017)
摘要
Very deep convolutional neural network has a strong representation power and becomes the dominant model to tackle very complex image classification problems. Due to the huge number of parameters, overfitting is always a primary problem in training a network without enough data. Data augmentation at input layer is a commonly used regularization method to make the trained model generalize better. In this paper, we propose that feature augmentation at intermediate layers can be also used to regularize the network. We implement a modified residual network by adding augmentation layers and train the model on CIFAR10. Experimental results demonstrate our method can successfully regularize the model. It significantly decreases the cross-entropy loss on test set although the training loss is higher than the original network. The final recognition accuracy on test set is also improved. In comparison with Dropout, our method can cooperate better with batch normalization to produce performance gain.
更多查看译文
关键词
Deep learning,CNN,Overfitting,Model regularization
AI 理解论文
溯源树
样例
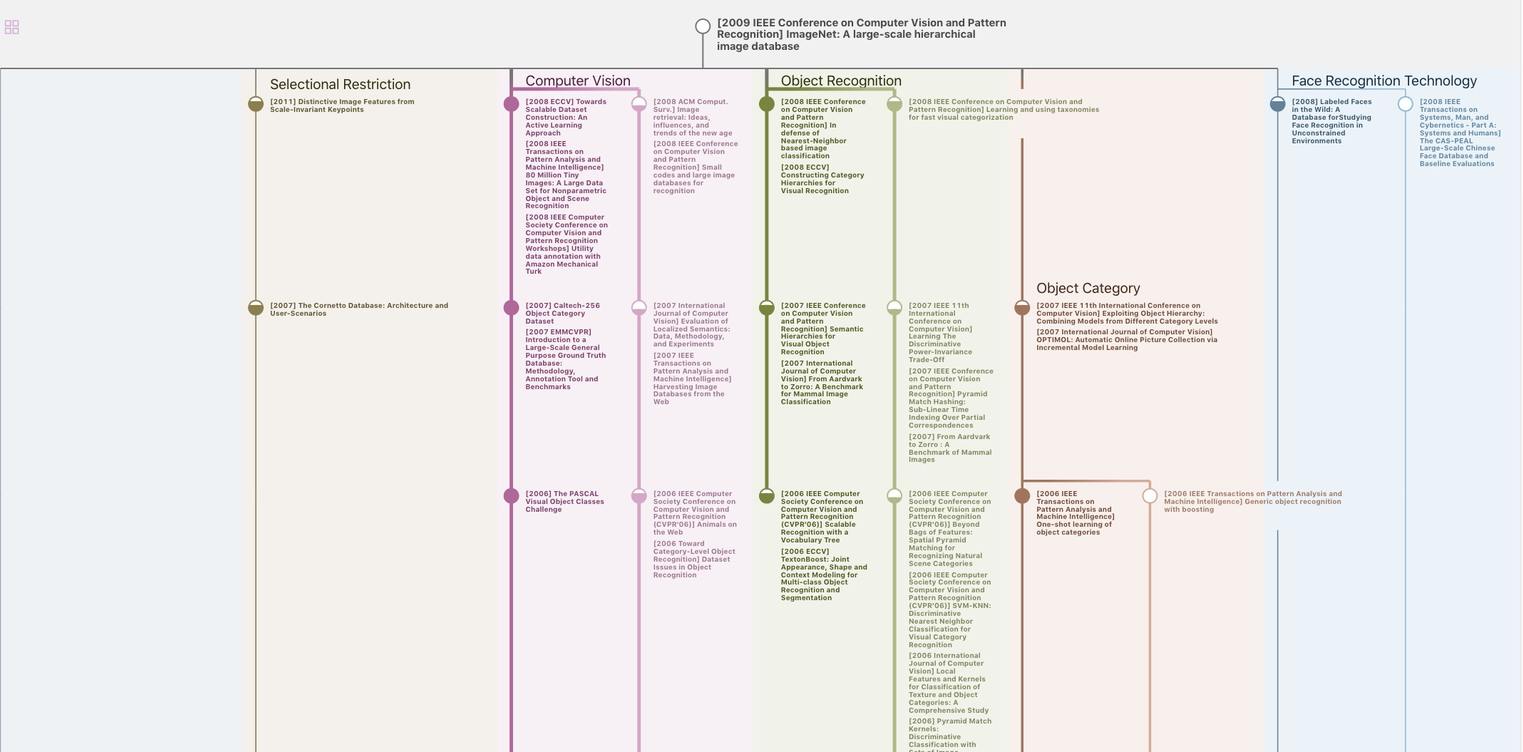
生成溯源树,研究论文发展脉络
Chat Paper
正在生成论文摘要