Near-Orthogonality Regularization In Kernel Methods
CONFERENCE ON UNCERTAINTY IN ARTIFICIAL INTELLIGENCE (UAI2017)(2017)
摘要
Kernel methods perform nonlinear learning in high-dimensional reproducing kernel Hilbert spaces (RKHSs). Even though their large model-capacity leads to high representational power, it also incurs substantial risk of over-fitting. To alleviate this problem, we propose a new regularization approach, near-orthogonality regularization, which encourages the RKHS functions to be close to being orthogonal. This effectively imposes a structural constraint over the function space, which reduces model complexity and can improve generalization performance. Besides, encouraging orthogonality reduces the redundancy among functions, which hence can reduce model size without compromising modeling power and better capture infrequent patterns in the data. Here, we define a family of orthogonality-promoting regularizers by encouraging the Gram matrix of the RKHS functions to be close to an identity matrix where the closeness is measured by Bregman matrix divergences. We apply these regularizers to two kernel methods, and develop an efficient ADMM-based algorithm to solve the regularized optimization problems. We analyze how near-orthogonality affects the generalization performance of kernel methods. Our results suggest that the closer the functions are to being orthogonal, the smaller the generalization error is. Experiments demonstrate the efficacy of near-orthogonality regularization in kernel methods.
更多查看译文
AI 理解论文
溯源树
样例
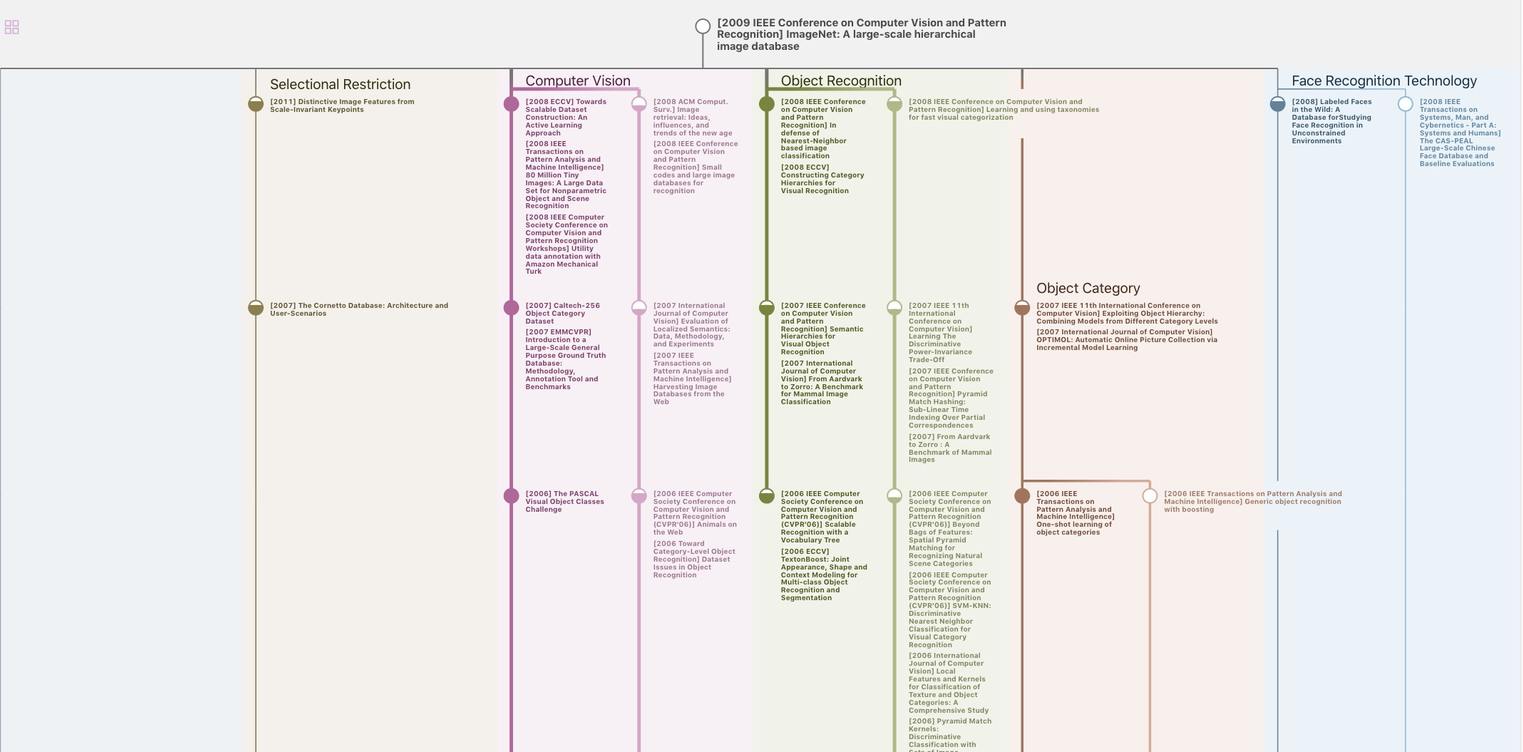
生成溯源树,研究论文发展脉络
Chat Paper
正在生成论文摘要