De-biased dart ensemble model for personalized recommendation
2017 IEEE INTERNATIONAL CONFERENCE ON MULTIMEDIA AND EXPO (ICME)(2017)
摘要
Personalized recommendation aims to use the historical behavior of users to recommend new items that are likely to be of interest to them. Due to a tiny improvement of it can lead to a huge profits, lots of giant e-commerce companies, such as Amazon, Alibaba and eBay, have put their great effort on this field. In this paper, we formulate the problem of personalized recommendation as a tree based regression task and present a de-biased DART ensemble model. To generate compact personalized profile of user, a decision tree based sparse encoding approach is first proposed to refine those raw behaviors, like clicks, views, and purchases from user-item interaction. Considering the problem of class imbalance in training the predictive models, resampling based de-biased DART model is utilized to form multiple weak predictors for recommendation. Thus, based on these learnt weak predictors, a stronger predictor can be built. Experimental results on real commercial dataset demonstrate the effectiveness of the proposed personalized recommendation model.
更多查看译文
关键词
Personalized feature engineering,Personalized recommendation,Decision tree,Ensemble learning
AI 理解论文
溯源树
样例
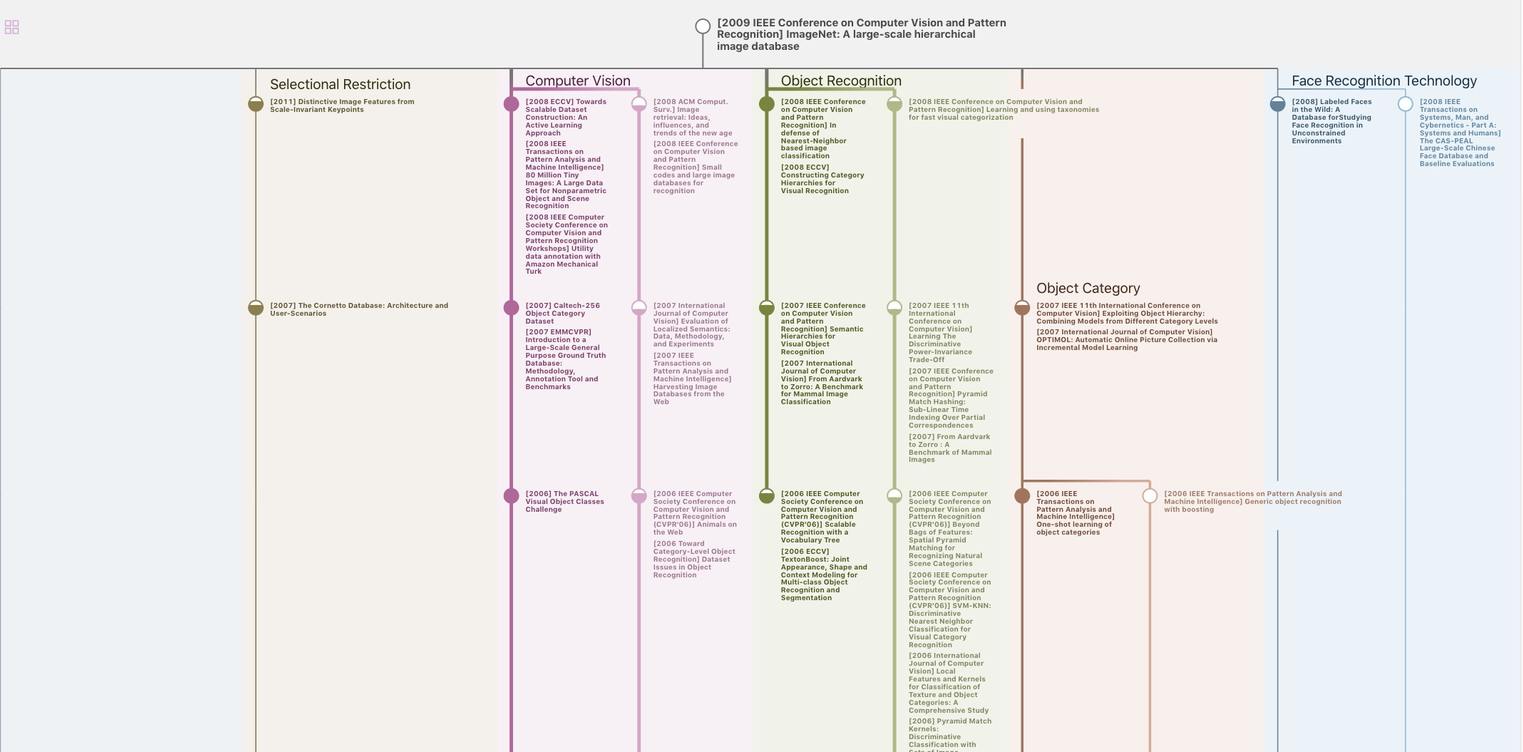
生成溯源树,研究论文发展脉络
Chat Paper
正在生成论文摘要