Conditional preference nets for user and item cold start problems in music recommendation
2017 IEEE INTERNATIONAL CONFERENCE ON MULTIMEDIA AND EXPO (ICME)(2017)
摘要
A great amount of data is usually needed for a recommender system to learn the associations between users and items. However, in practical applications, new users and new items emerge everyday, and the system has to react to them promptly. The ability to recommend proper items to new users affects the users' first impression and accordingly the retention rate, whereas recommending new items to proper users contributes to the freshness of the recommendation. In this paper, we propose a deep learning model called the conditional preference nets (CPN) to deal with both new users and items under the same model framework. CPN employs an introductory user survey to learn about new users, and content features automatically extracted from items for the item side. Through a new idea called the preference vectors and an existing content embedding technique, the same model can capitalize the observed associations between known (i.e. old) users and items, thereby benefiting from the cumulative knowledge of user behavior gained over time. We validate the superiority of CPN over prior arts using the Million Song Dataset. We also demonstrate how CPN allows a user to pick either genres, artists, or the combination of them in the introductory survey.
更多查看译文
关键词
Content-based recommendation,cold start problem,introductory survey,convolutional neural network
AI 理解论文
溯源树
样例
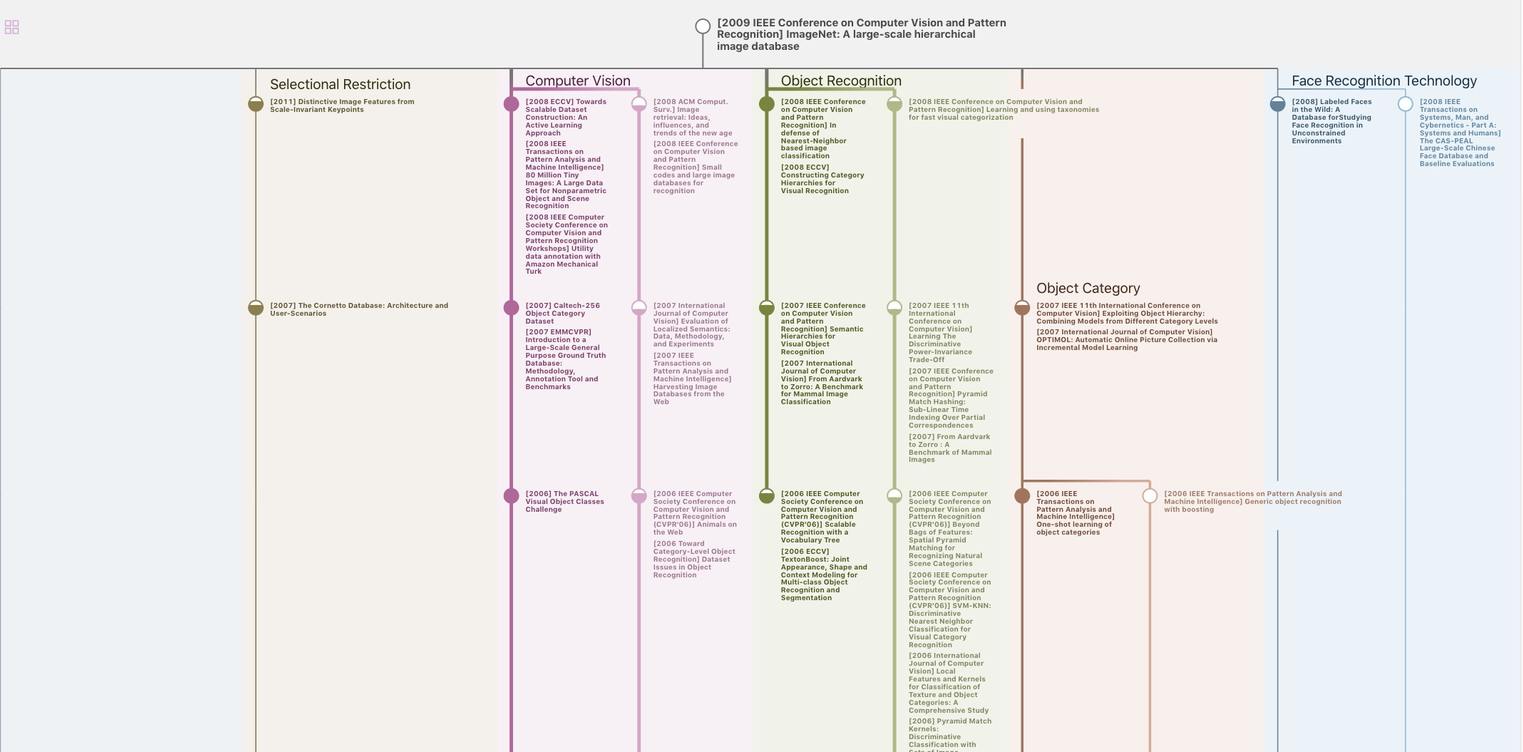
生成溯源树,研究论文发展脉络
Chat Paper
正在生成论文摘要