Population-Shrinkage of Covariance to Estimate Better Brain Functional Connectivity.
MICCAI(2017)
摘要
Brain functional connectivity, obtained from functional Magnetic Resonance Imaging at rest (r-fMRI), reflects inter-subject variations in behavior and characterizes neuropathologies. It is captured by the covariance matrix between time series of remote brain regions. With noisy and short time series as in r-fMRI, covariance estimation calls for penalization, and shrinkage approaches are popular. Here we introduce a new covariance estimator based on a non-isotropic shrinkage that integrates prior knowledge of the covariance distribution over a large population. The estimator performs shrinkage tailored to the Riemannian geometry of symmetric positive definite matrices, coupled with a probabilistic modeling of the subject and population covariance distributions. Experiments on a large-scale dataset show that such estimators resolve better intra- and inter-subject functional connectivities compared existing covariance estimates. We also demonstrate that the estimator improves the relationship across subjects between their functional-connectivity measures and their behavioral assessments.
更多查看译文
AI 理解论文
溯源树
样例
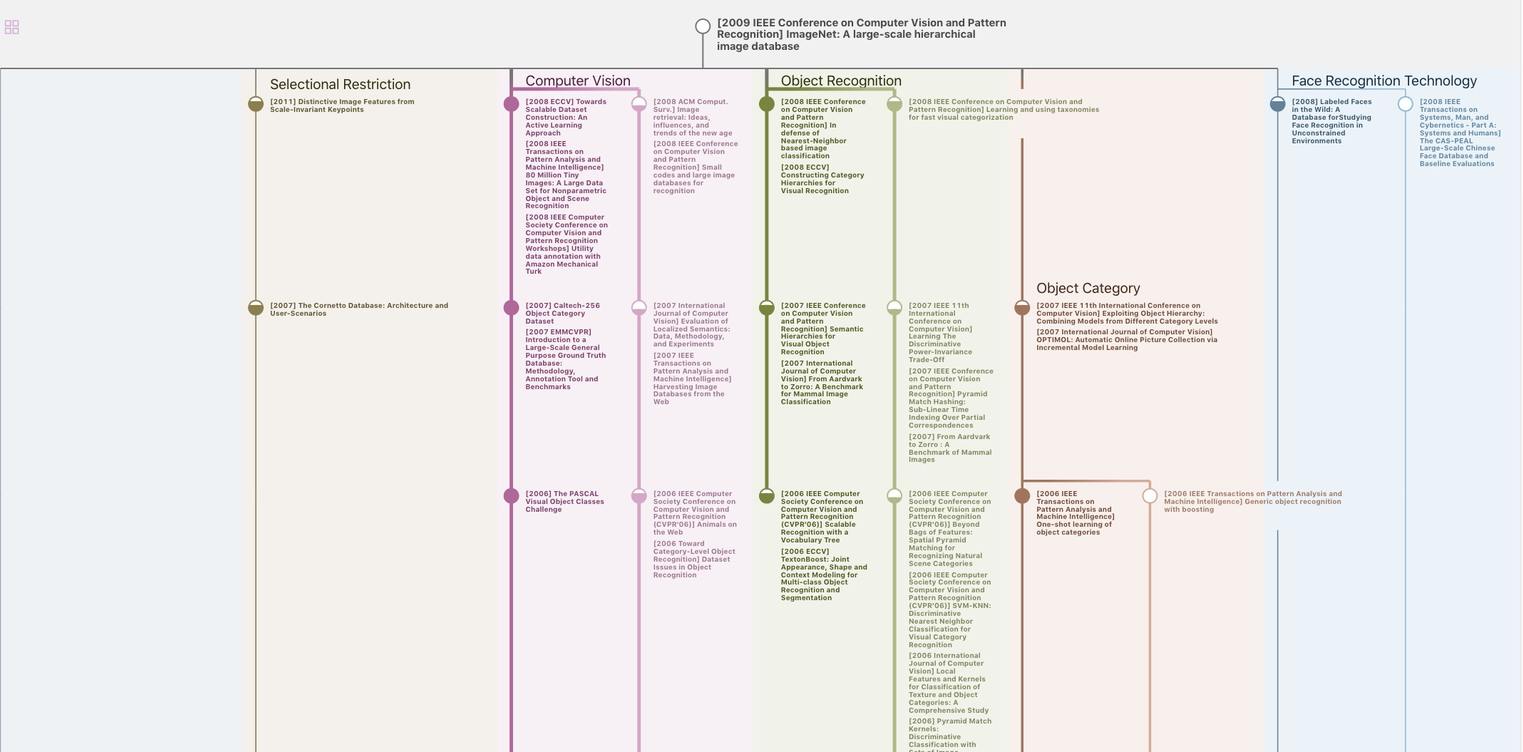
生成溯源树,研究论文发展脉络
Chat Paper
正在生成论文摘要