Motor Imagery Eeg Classification With Optimal Subset Of Wavelet Based Common Spatial Pattern And Kernel Extreme Learning Machine
2017 39TH ANNUAL INTERNATIONAL CONFERENCE OF THE IEEE ENGINEERING IN MEDICINE AND BIOLOGY SOCIETY (EMBC)(2017)
Abstract
Performance of motor imagery based brain-computer interfaces (MI BCIs) greatly depends on how to extract the features. Various versions of filter-bank based common spatial pattern have been proposed and used in MI BCIs. Filter-bank based common spatial pattern has more number of features compared with original common spatial pattern. As the number of features increases, the MI BCIs using filter-bank based common spatial pattern can face overfitting problems. In this study, we used eigenvector centrality feature selection method, wavelet packet decomposition common spatial pattern, and kernel extreme learning machine to improve the performance of MI BCIs and avoid overfitting problems. Furthermore, the computational speed was improved by using kernel extreme learning machine.
MoreTranslated text
Key words
motor imagery based brain-computer interfaces (MI BCIs), wavelet packet decomposition common spatial pattern (WPD-CSP), feature selection, extreme learning machine (ELM)
AI Read Science
Must-Reading Tree
Example
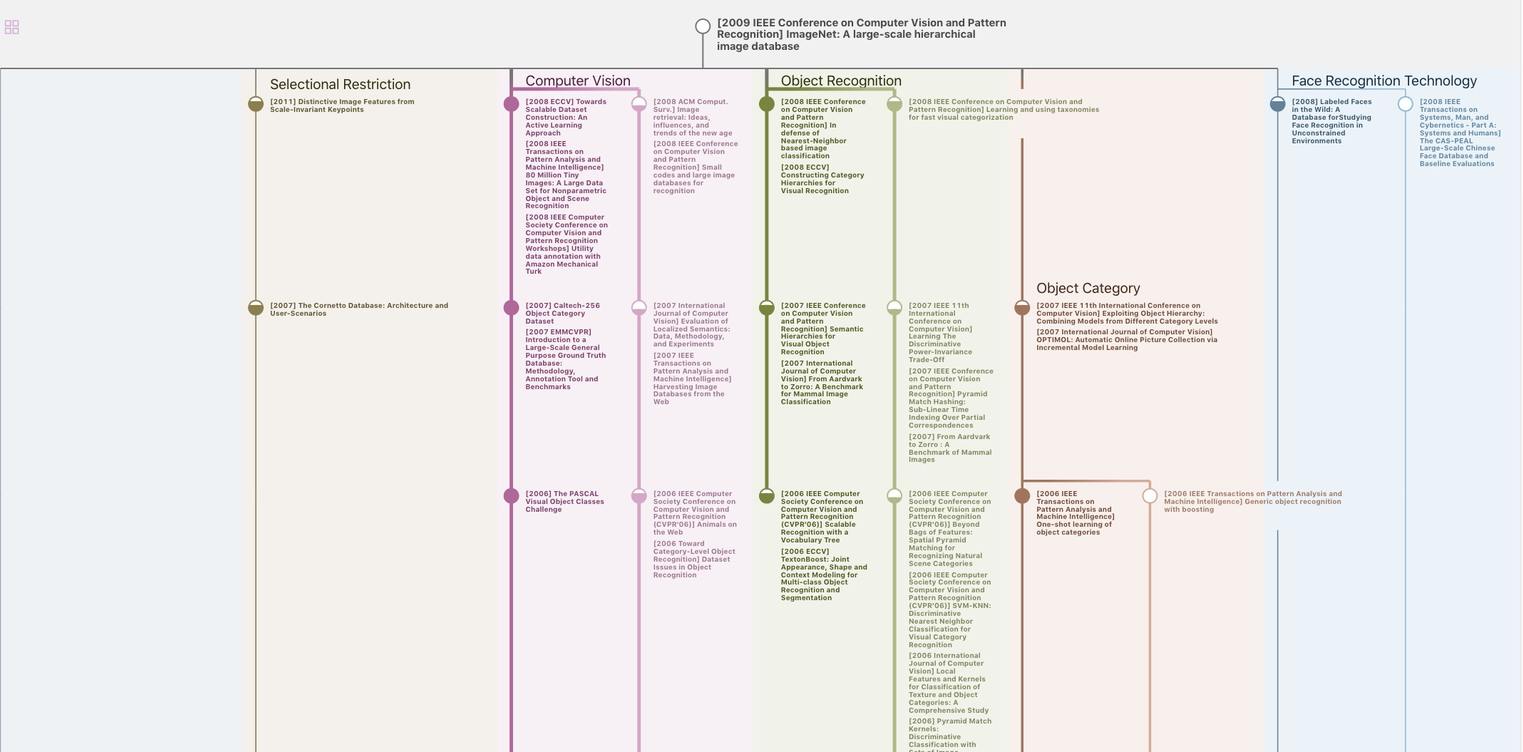
Generate MRT to find the research sequence of this paper
Chat Paper
Summary is being generated by the instructions you defined