Classification Models To Predict Vasopressor Administration For Septic Shock In The Emergency Department
2017 39TH ANNUAL INTERNATIONAL CONFERENCE OF THE IEEE ENGINEERING IN MEDICINE AND BIOLOGY SOCIETY (EMBC)(2017)
摘要
Optimal management of sepsis and septic shock in the emergency department (ED) involves timely decisions related to intravenous fluid resuscitation and initiation of vasoactive medication support. A decision-support tool trained on electronic health record data, can help improve this complex decision. We retrospectively extracted vital signs, lab measurements, and fluid administration information from 807 patient visits over a two-year period to a major ED. Patients selected for inclusion had a high likelihood of septic shock. We trained binary classifiers to discriminate between patients administered vasopressors in the ED and those not administered vasopressors at any point. Using features extracted from the entire ED visit record yielded a maximum area under the receiver-operating characteristic curve (AUC) of 0.798 (95% CI 0.725-0.849) in a hold-out test set. In a separate task, we used individual vital signs observations with lab results to predict vasopressor administration, yielding a maximum AUC of 0.762 (95% CI 0.748-0.777). Lastly, we trained separate classifiers for different subgroups of vital signs observations. These subgroups were defined by the cumulative number of fluid boluses delivered at the time of the observation. The maximum AUC achieved by any of these classifiers was 0.815 (95% CI 0.784-0.853), occurring for vital signs observations made after 2 bolus administrations. Classifiers in all tasks significantly outperformed existing clinical tools for assessing prognosis in ED sepsis. This work shows how relatively few features can provide instantaneous and accurate prediction of need for an intervention that is typically a complex clinical decision.
更多查看译文
关键词
Emergency Service, Hospital,Fluid Therapy,Humans,Resuscitation,Shock, Septic
AI 理解论文
溯源树
样例
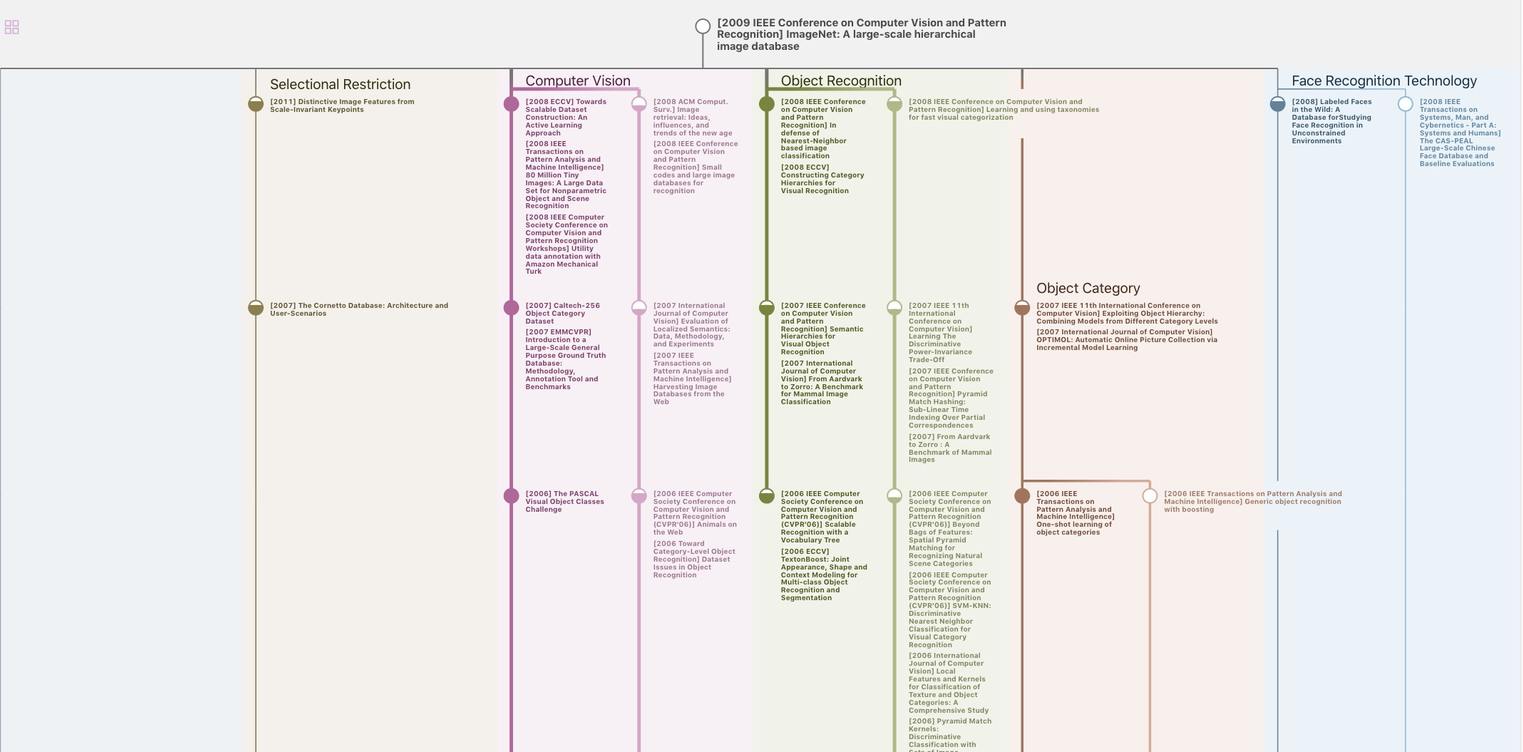
生成溯源树,研究论文发展脉络
Chat Paper
正在生成论文摘要