Multimodal Fusion with Recurrent Neural Networks for Rumor Detection on Microblogs.
MM '17: ACM Multimedia Conference Mountain View California USA October, 2017(2017)
摘要
Microblogs have become popular media for news propagation in recent years. Meanwhile, numerous rumors and fake news also bloom and spread wildly on the open social media platforms. Without verification, they could seriously jeopardize the credibility of microblogs. We observe that an increasing number of users are using images and videos to post news in addition to texts. Tweets or microblogs are commonly composed of text, image and social context. In this paper, we propose a novel Recurrent Neural Network with an attention mechanism (att-RNN) to fuse multimodal features for effective rumor detection. In this end-to-end network, image features are incorporated into the joint features of text and social context, which are obtained with an LSTM (Long-Short Term Memory) network, to produce a reliable fused classification. The neural attention from the outputs of the LSTM is utilized when fusing with the visual features. Extensive experiments are conducted on two multimedia rumor datasets collected from Weibo and Twitter. The results demonstrate the effectiveness of the proposed end-to-end att-RNN in detecting rumors with multimodal contents.
更多查看译文
关键词
Rumor detection, multimodal fusion, LSTM, attention mechanism, microblog
AI 理解论文
溯源树
样例
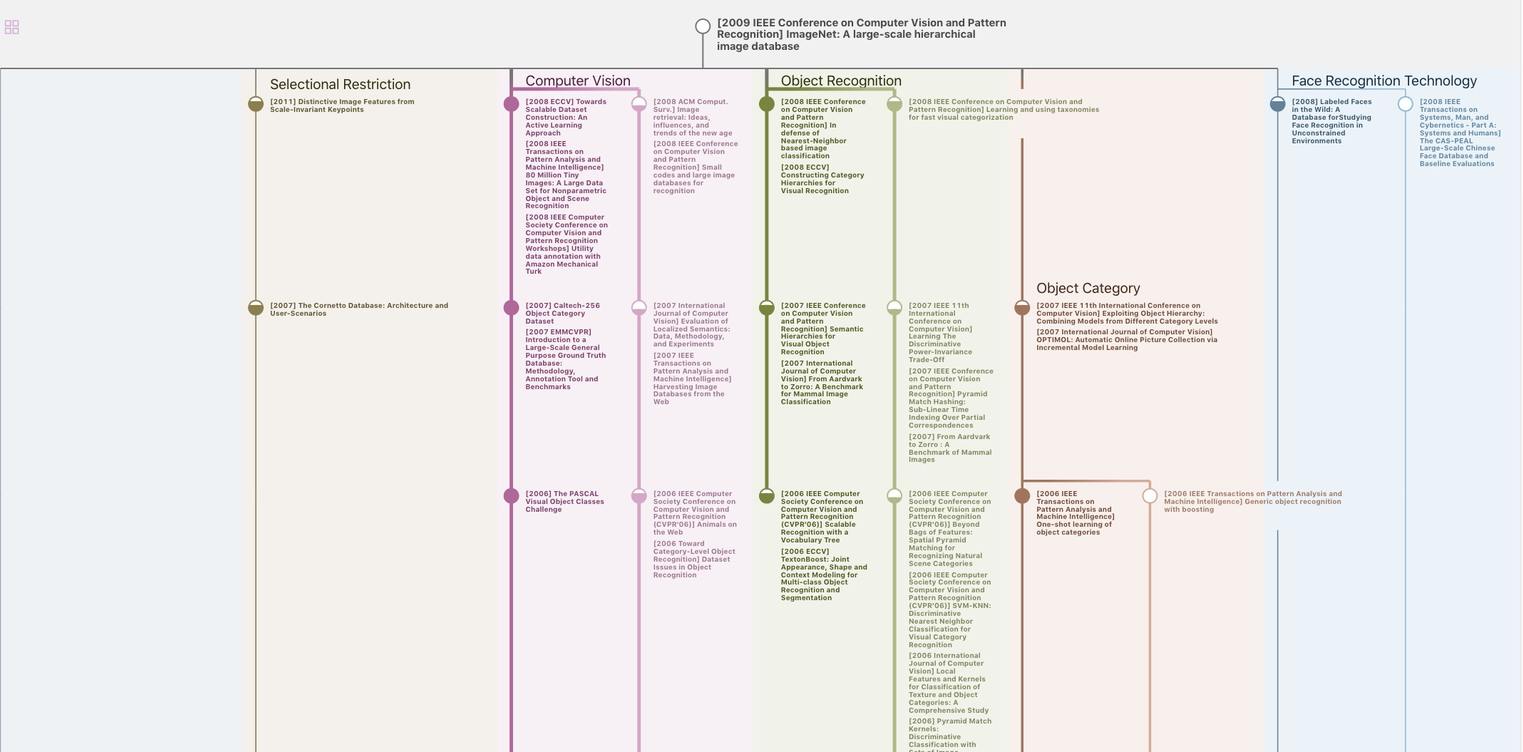
生成溯源树,研究论文发展脉络
Chat Paper
正在生成论文摘要