Multitask Spectral Learning Of Weighted Automata
ADVANCES IN NEURAL INFORMATION PROCESSING SYSTEMS 30 (NIPS 2017)(2017)
摘要
We consider the problem of estimating multiple related functions computed by weighted automata (WFA). We first present a natural notion of relatedness between WFAs by considering to which extent several WFAs can share a common underlying representation. We then introduce the novel model of vector-valued WFA which conveniently helps us formalize this notion of relatedness. Finally, we propose a spectral learning algorithm for vector-valued WFAs to tackle the multitask learning problem. By jointly learning multiple tasks in the form of a vector-valued WFA, our algorithm enforces the discovery of a representation space shared between tasks. The benefits of the proposed multitask approach are theoretically motivated and showcased through experiments on both synthetic and real world datasets.
更多查看译文
AI 理解论文
溯源树
样例
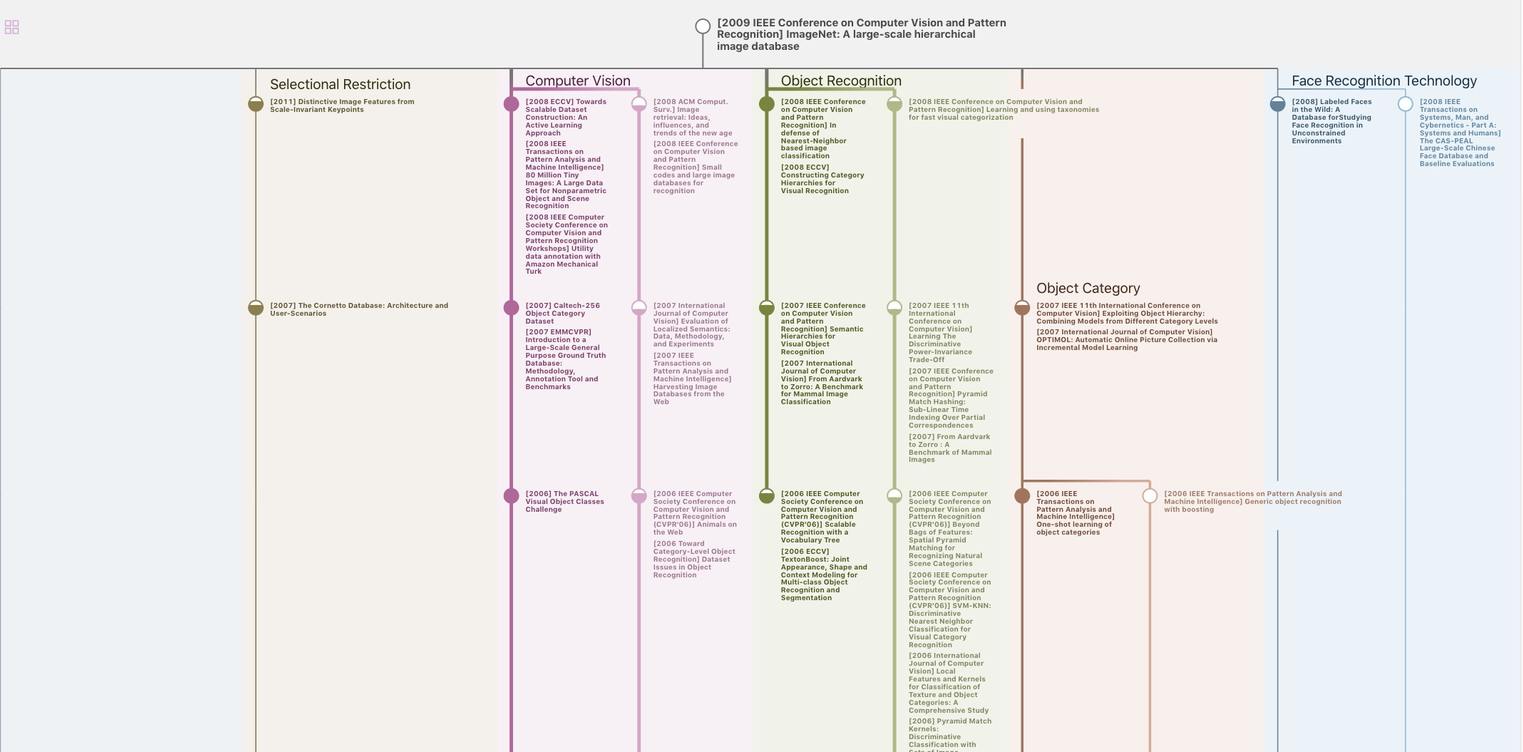
生成溯源树,研究论文发展脉络
Chat Paper
正在生成论文摘要