Learning To Weight Color And Depth For Rgb-D Visual Search
IMAGE ANALYSIS AND PROCESSING,(ICIAP 2017), PT I(2017)
摘要
Both color and depth information may be deployed to seek by content through RGB-D imagery. Previous works dealing with global descriptors for RGB-D images advocate a decision level fusion whereby independently computed color and depth representations are juxtaposed to pursue similarity search. Differently, in this paper we propose a learning-to-rank paradigm aimed at weighting the two information channels according to the specific traits of the task and data at hand, thereby effortlessly addressing the potential diversity across applications. In particular, we propose a novel method, referred to as kNN-rank, which can learn the regularities among the outputs yielded by similarity-based queries. A further novel contribution of this paper concerns the Hyper-RGBD framework, a set of tools conceived to enable seamless aggregation of existing RGB-D datasets in order to obtain new data featuring desired peculiarities and cardinality.
更多查看译文
关键词
RGB-D image search, Compact descriptors, Learning-to-rank
AI 理解论文
溯源树
样例
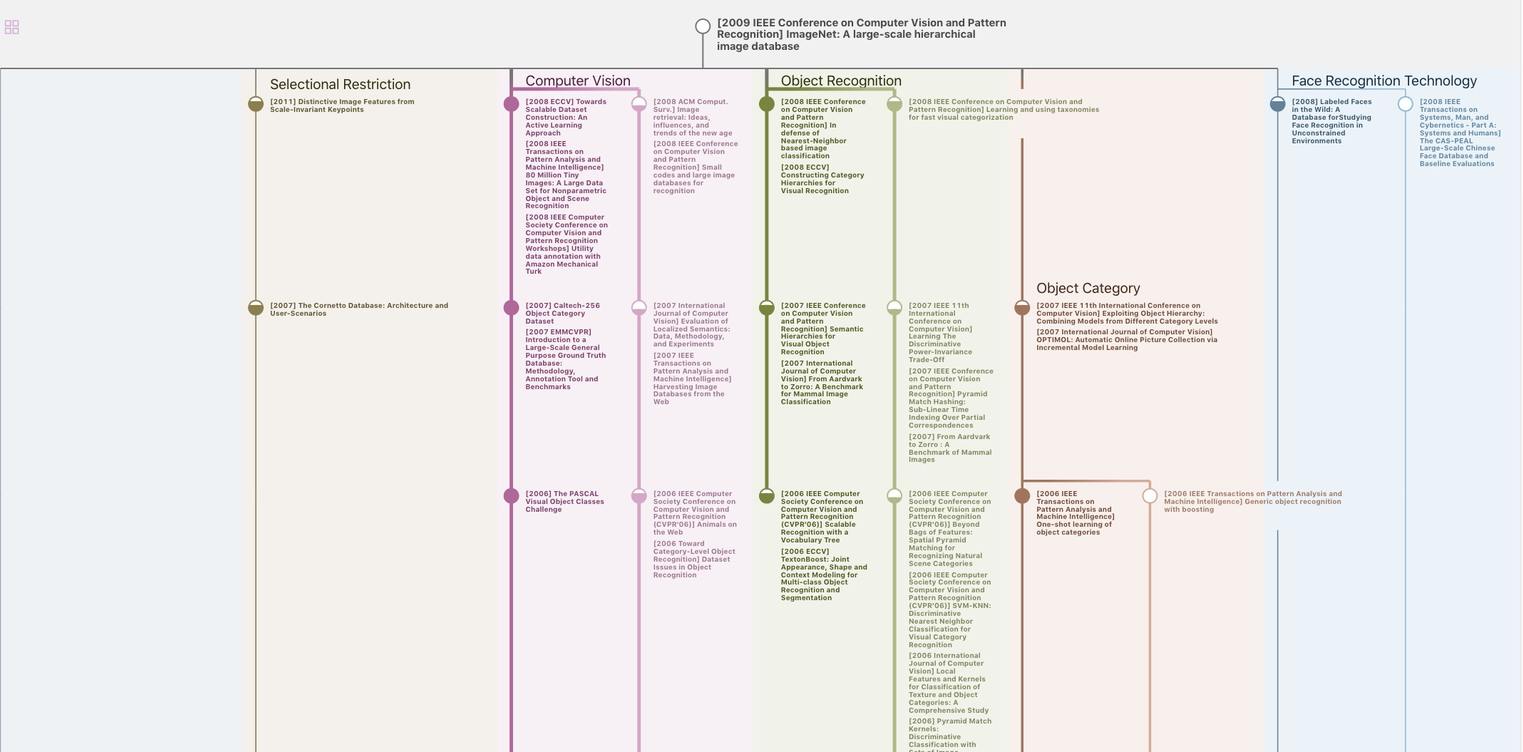
生成溯源树,研究论文发展脉络
Chat Paper
正在生成论文摘要