A Novel Crowd Density Estimation Technique using Local Binary Pattern and Gabor Features
2017 14th IEEE International Conference on Advanced Video and Signal Based Surveillance (AVSS)(2017)
摘要
Crowd density estimation is an effective automated video surveillance technique to ensure crowd safety. In spite of various efforts being taken to estimate crowd density, it remains a challenging task. This paper proposes a new texture feature-based approach for the estimation of crowd density where two efficient texture features namely Local Binary Pattern (LBP) and Gabor Filter are used. The LBP features are computed using an extended version which reduces the dimension of conventional LBP and the Gabor features are extracted after convolving the original image with a bank of Log-Gabor filters computed at different scales and orientations. Finally, the LBP and Gabor features are concatenated to yield the final feature vector which is used to train a multi-class Support Vector Machine (SVM) classifier. The proposed technique is evaluated on the benchmarked PETS 2009 dataset, and a maximum accuracy of 90.3% is obtained for the proposed texture combination. The experimental results show the better performance of the proposed approach as compared to other conventional techniques.
更多查看译文
关键词
crowd density estimation technique,local binary pattern,Gabor features,effective automated video surveillance technique,crowd safety,texture feature,efficient texture features,Gabor Filter,LBP features,conventional LBP,Log-Gabor,final feature vector,multiclass Support Vector Machine classifier
AI 理解论文
溯源树
样例
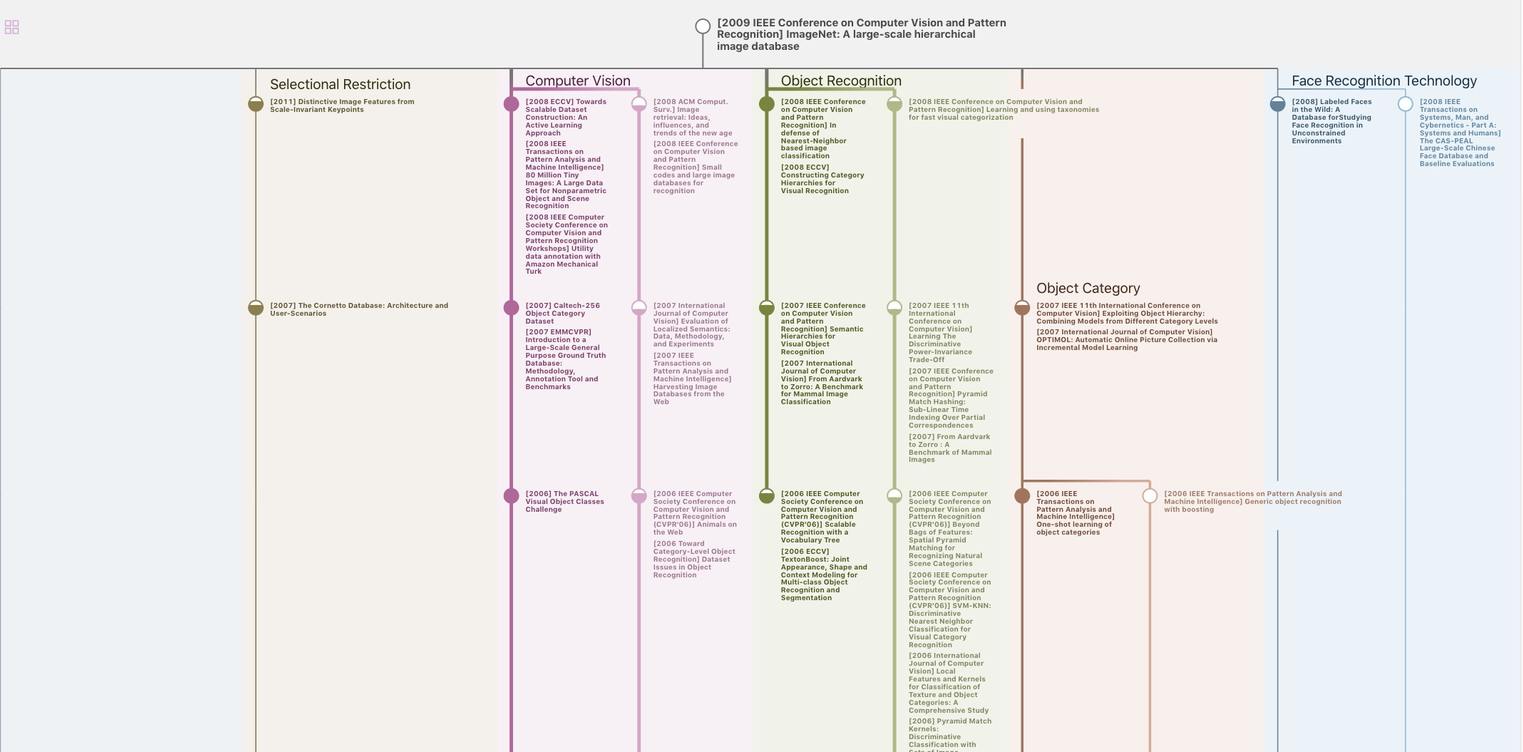
生成溯源树,研究论文发展脉络
Chat Paper
正在生成论文摘要