SLAQ: quality-driven scheduling for distributed machine learning.
SoCC '17: ACM Symposium on Cloud Computing Santa Clara California September, 2017(2018)
摘要
Training machine learning (ML) models with large datasets can incur significant resource contention on shared clusters. This training typically involves many iterations that continually improve the quality of the model. Yet in exploratory settings, better models can be obtained faster by directing resources to jobs with the most potential for improvement. We describe SLAQ, a cluster scheduling system for approximate ML training jobs that aims to maximize the overall job quality.
When allocating cluster resources, SLAQ explores the quality-runtime trade-offs across multiple jobs to maximize system-wide quality improvement. To do so, SLAQ leverages the iterative nature of ML training algorithms, by collecting quality and resource usage information from concurrent jobs, and then generating highly-tailored quality-improvement predictions for future iterations. Experiments show that SLAQ achieves an average quality improvement of up to 73% and an average delay reduction of up to 44% on a large set of ML training jobs, compared to resource fairness schedulers.
更多查看译文
关键词
Scheduling, Machine Learning, Approximate Computing, Resource Management, Quality
AI 理解论文
溯源树
样例
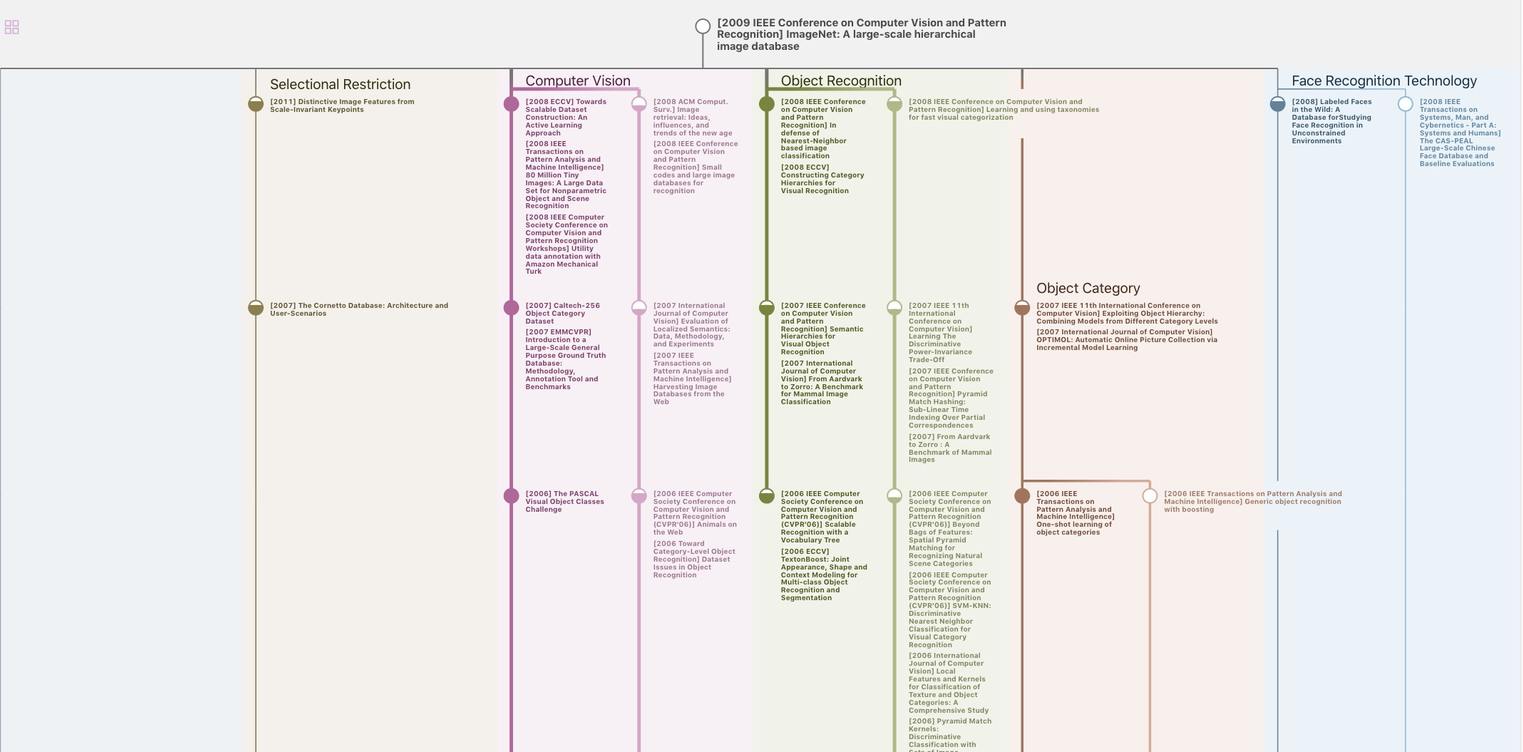
生成溯源树,研究论文发展脉络
Chat Paper
正在生成论文摘要