The effects of environmental structure on the evolution of modularity in a pattern classifier.
FOURTEENTH EUROPEAN CONFERENCE ON ARTIFICIAL LIFE (ECAL 2017)(2017)
摘要
We examine hierarchical modularity - modularity on multiple levels, in which the modules at a lower level of abstraction can serve as nodes in a network at a higher level of abstraction that also has positive modularity - as well as degree of modularity on a single level of abstraction, in evolved neural networks in single-task, parallel-subtask environments, and sequential-subtask environments, using a common benchmark problem. We determine that top-performing networks evolved in the sequential-subtask environment have both more levels of hierarchical modularity, and a higher degree of modularity within levels, than those involved in either the single-task or parallel-subtask environment. In the single-task environment, both single-level and hierarchical modularity tend to rise initially before stagnating and even declining, while in the sequential-subtask environment, both single-level and hierarchical modularity tend to rise throughout the period of evolution.
更多查看译文
AI 理解论文
溯源树
样例
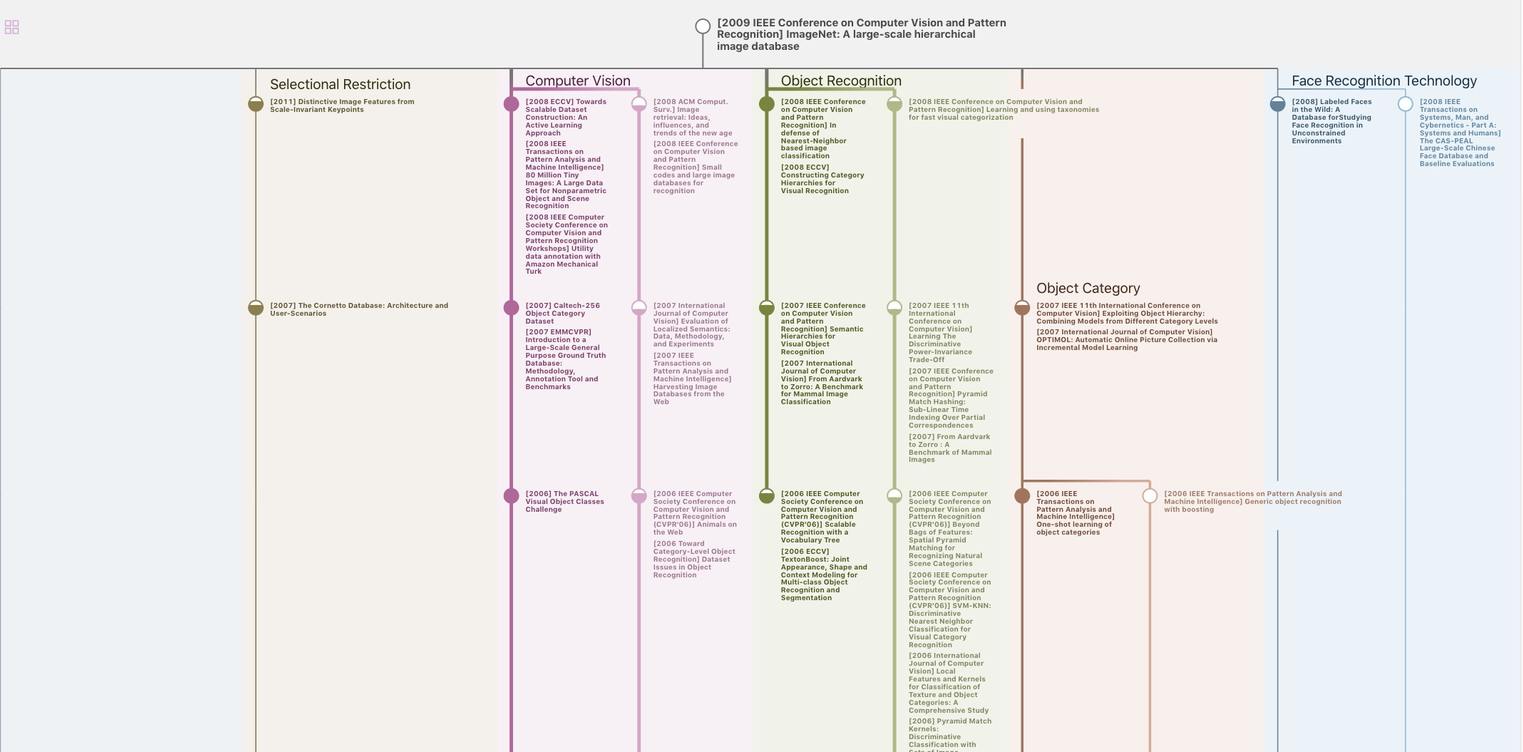
生成溯源树,研究论文发展脉络
Chat Paper
正在生成论文摘要