A Low Power, Fully Event-Based Gesture Recognition System
30TH IEEE CONFERENCE ON COMPUTER VISION AND PATTERN RECOGNITION (CVPR 2017)(2017)
摘要
We present the first gesture recognition system implemented end-to-end on event-based hardware, using a TrueNorth neurosynaptic processor to recognize hand gestures in real-time at low power from events streamed live by a Dynamic Vision Sensor (DVS). The biologically inspired DVS transmits data only when a pixel detects a change, unlike traditional frame-based cameras which sample every pixel at a fixed frame rate. This sparse, asynchronous data representation lets event-based cameras operate at much lower power than frame-based cameras. However, much of the energy efficiency is lost if, as in previous work, the event stream is interpreted by conventional synchronous processors. Here, for the first time, we process a live DVS event stream using TrueNorth, a natively event-based processor with 1 million spiking neurons. Configured here as a convolutional neural network (CNN), the TrueNorth chip identifies the onset of a gesture with a latency of 105 ms while consuming less than 200mW. The CNN achieves 96.5% out-of-sample accuracy on a newly collected DVS dataset (DvsGesture) comprising 11 hand gesture categories from 29 subjects under 3 illumination conditions.
更多查看译文
关键词
asynchronous data representation,cameras,live DVS event stream,TrueNorth chip,TrueNorth neurosynaptic processor,Dynamic Vision Sensor,biologically inspired DVS,fixed frame rate,sparse data representation,hand gesture recognition,DVS dataset,low power fully event-based gesture recognition system,natively event-based processor,spiking neurons,convolutional neural network,CNN,illumination conditions,synchronous processors,time 105.0 ms,power 200.0 mW
AI 理解论文
溯源树
样例
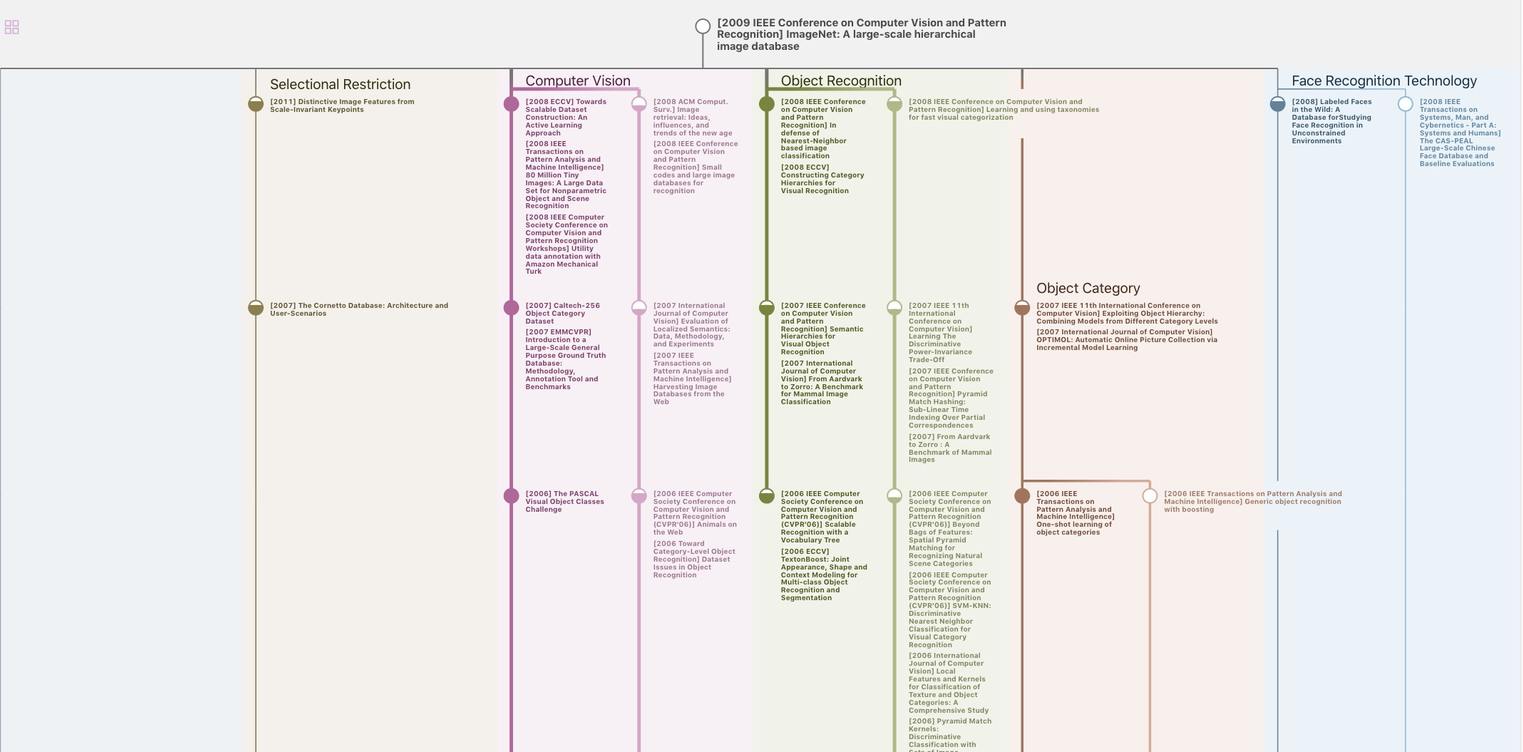
生成溯源树,研究论文发展脉络
Chat Paper
正在生成论文摘要