Webly Supervised Semantic Segmentation
Computer Vision and Pattern Recognition(2017)
关键词
webly supervised semantic segmentation,weakly supervised semantic segmentation algorithm,image tags,web images,clean foregrounds,common backgrounds,realistic scenes,three-stage training pipeline,semantic segmentation models,class-specific shallow neural network,segmentation masks,deep convolutional neural network,end-to-end training,semantic segmentation approaches,PASCAL VOC 2012 segmentation benchmark,VOC
AI 理解论文
溯源树
样例
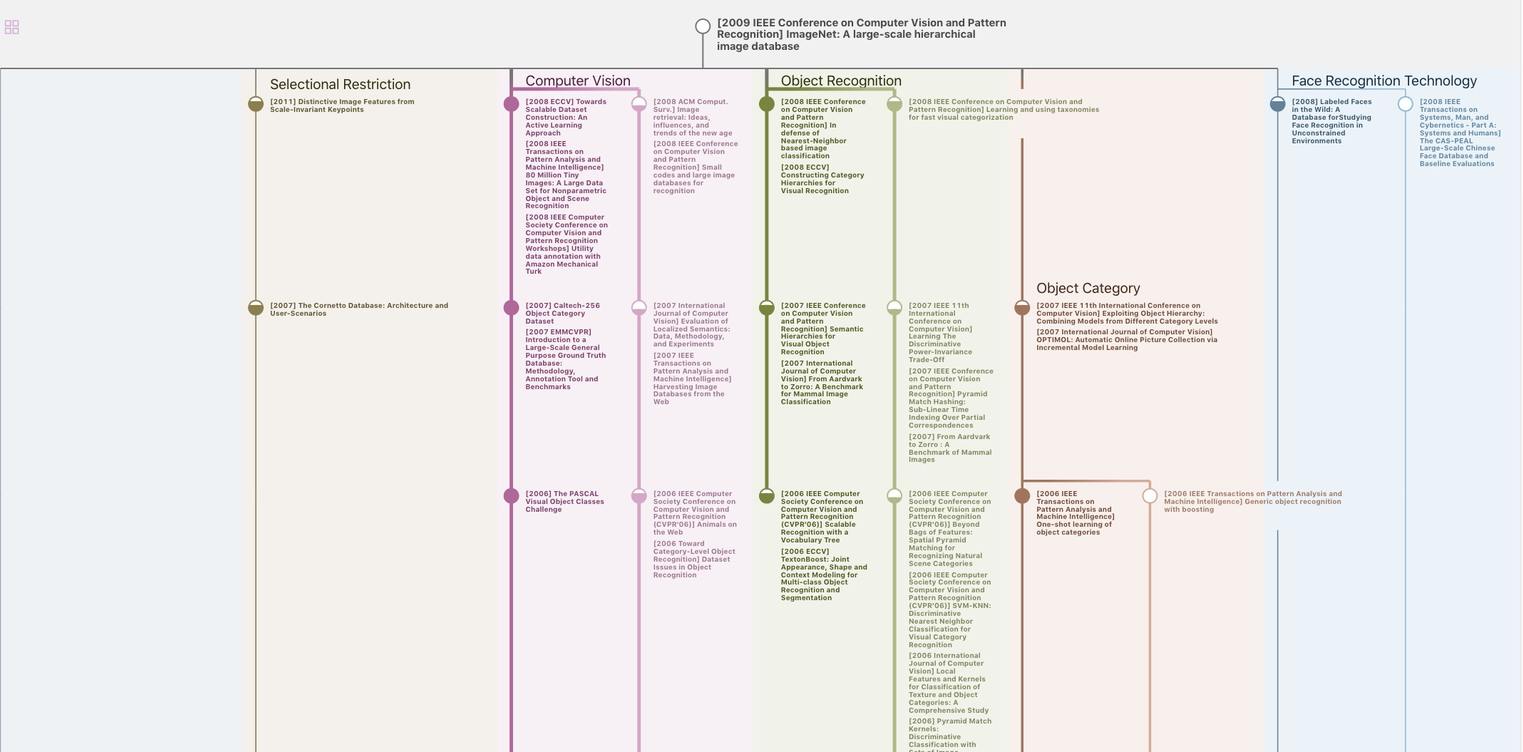
生成溯源树,研究论文发展脉络
Chat Paper
正在生成论文摘要