Fast Boosting Based Detection Using Scale Invariant Multimodal Multiresolution Filtered Features
30TH IEEE CONFERENCE ON COMPUTER VISION AND PATTERN RECOGNITION (CVPR 2017)(2017)
Abstract
In this paper we propose a novel boosting-based sliding window solution for object detection which can keep up with the precision of the state-of-the art deep learning approaches, while being 10 to 100 times faster. The solution takes advantage of multisensorial perception and exploits information from color, motion and depth. We introduce multimodal multiresolution filtering of signal intensity, gradient magnitude and orientation channels, in order to capture structure at multiple scales and orientations. To achieve scale invariant classification features, we analyze the effect of scale change on features for different filter types and propose a correction scheme. To improve recognition we incorporate 2D and 3D context by generating spatial, geometric and symmetrical channels. Finally, we evaluate the proposed solution on multiple benchmarks for the detection of pedestrians, cars and bicyclists. We achieve competitive results at over 25 frames per second.
MoreTranslated text
Key words
image motion,image depth,filter types,fast boosting-based detection,scale invariant multimodal multiresolution filtered features,image color,scale change,scale invariant classification features,orientation channels,gradient magnitude,signal intensity,multimodal multiresolution filtering,multisensorial perception,object detection,window solution,multiple benchmarks,symmetrical channels,geometric channels,spatial channels
AI Read Science
Must-Reading Tree
Example
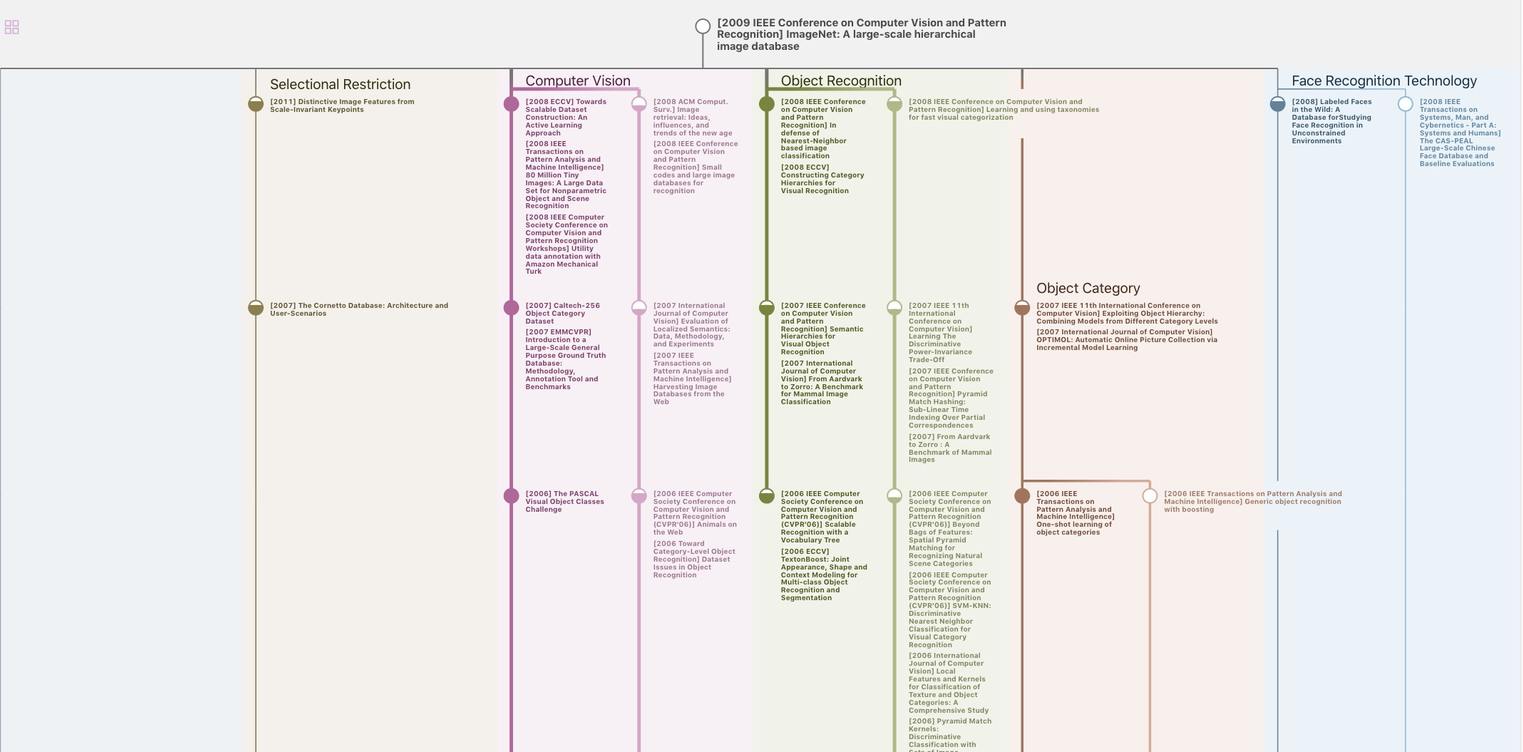
Generate MRT to find the research sequence of this paper
Chat Paper
Summary is being generated by the instructions you defined