Block matching sparsity regularization-based image reconstruction for incomplete projection data in computed tomography.
PHYSICS IN MEDICINE AND BIOLOGY(2018)
摘要
In medical imaging many conventional regularization methods, such as total variation or total generalized variation, impose strong prior assumptions which can only account for very limited classes of images. A more reasonable sparse representation frame for images is still badly needed. Visually understandable images contain meaningful patterns, and combinations or collections of these patterns can be utilized to form some sparse and redundant representations which promise to facilitate image reconstructions. In this work, we propose and study block matching sparsity regularization (BMSR) and devise an optimization program using BMSR for computed tomography (CT) image reconstruction for an incomplete projection set. The program is built as a constrained optimization, minimizing the L1-norm of the coefficients of the image in the transformed domain subject to data observation and positivity of the image itself. To solve the program efficiently, a practical method based on the proximal point algorithm is developed and analyzed. In order to accelerate the convergence rate, a practical strategy for tuning the BMSR parameter is proposed and applied. The experimental results for various settings, including real CT scanning, have verified the proposed reconstruction method showing promising capabilities over conventional regularization.
更多查看译文
关键词
computed tomography,image reconstruction,block matching sparsity regularization,proximal point algorithm,projection onto convex set
AI 理解论文
溯源树
样例
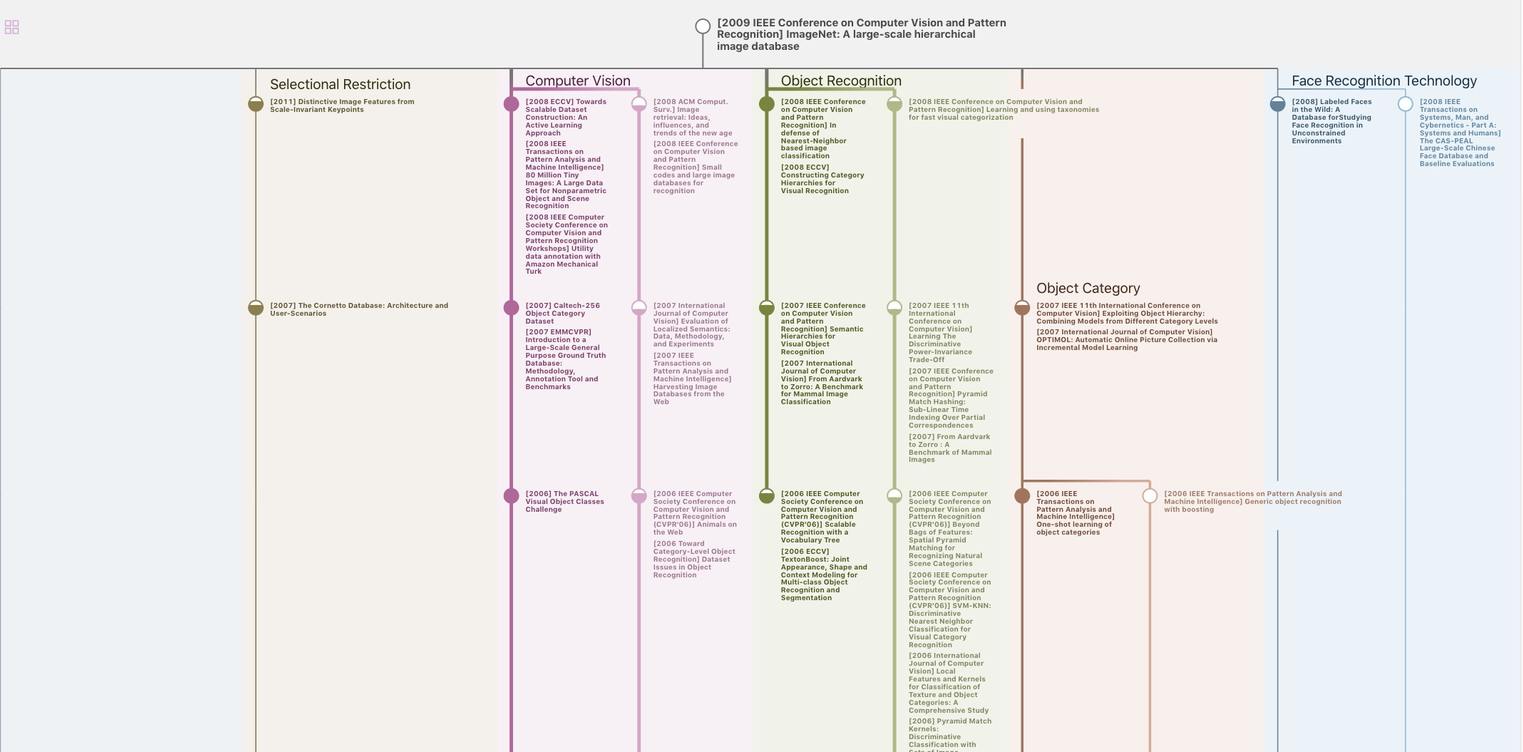
生成溯源树,研究论文发展脉络
Chat Paper
正在生成论文摘要