TransNet: Translation-Based Network Representation Learning for Social Relation Extraction.
IJCAI(2017)
摘要
Conventional network representation learning (NRL) models learn low-dimensional vertex representations by simply regarding each edge as a binary or continuous value. However, there exists rich semantic information on edges and the interactions between vertices usually preserve distinct meanings, which are largely neglected by most existing NRL models. In this work, we present a novel Translation-based NRL model, TransNet, by regarding the interactions between vertices as a translation operation. Moreover, we formalize the task of Social Relation Extraction (SRE) to evaluate the capability of NRL methods on modeling the relations between vertices. Experimental results on SRE demonstrate that TransNet significantly outperforms other baseline methods by 10% to 20% on hits@ 1. The source code and datasets can be obtained from https://github.com/thunlp/TransNet.
更多查看译文
关键词
social relation extraction,network,translation-based
AI 理解论文
溯源树
样例
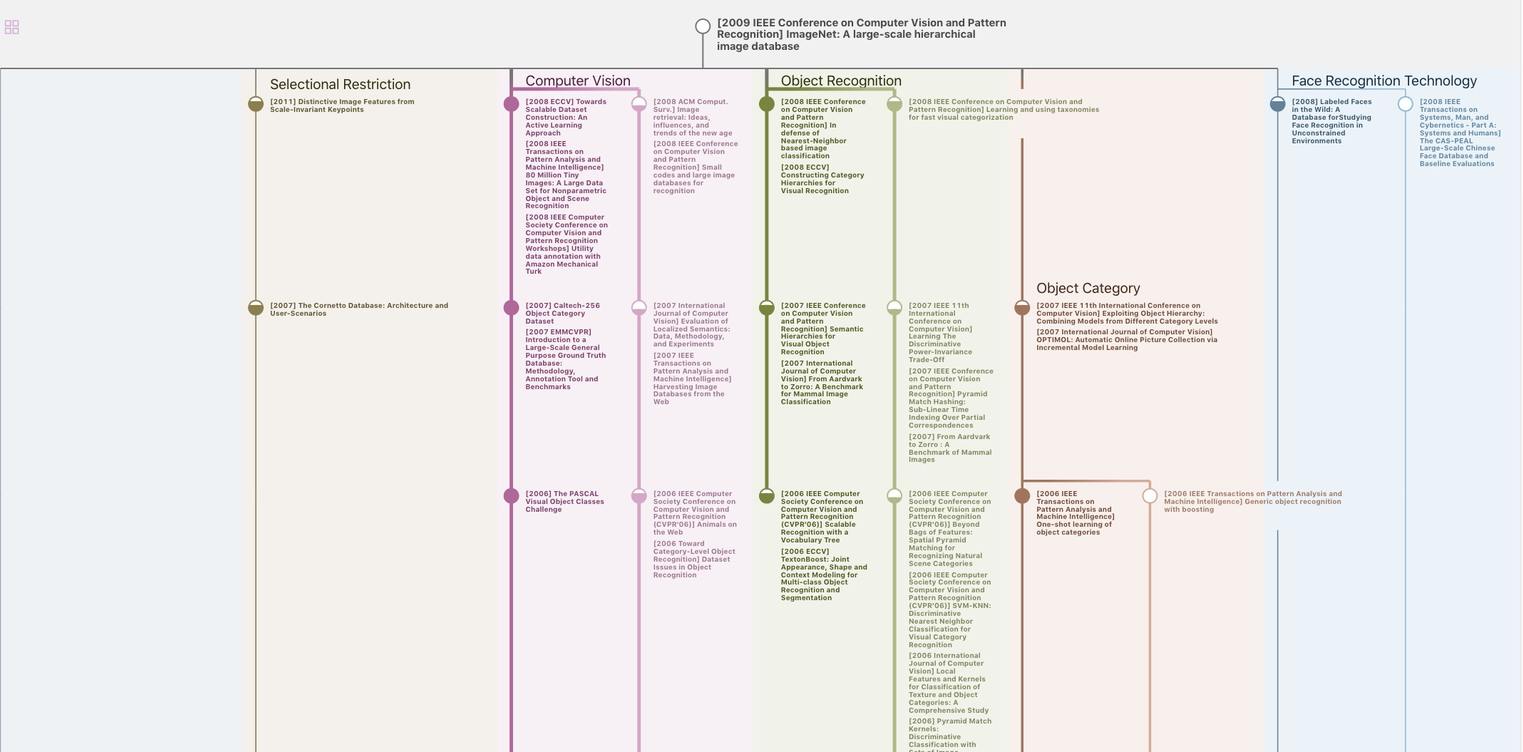
生成溯源树,研究论文发展脉络
Chat Paper
正在生成论文摘要