Learning to Explain Entity Relationships by Pairwise Ranking with Convolutional Neural Networks.
IJCAI(2017)
Abstract
Providing a plausible explanation for the relationship between two related entities is an important task in some applications of knowledge graphs, such as in search engines. However, most existing methods require a large number of manually labeled training data, which cannot be applied in large-scale knowledge graphs due to the expensive data annotation. In addition, these methods typically rely on costly handcrafted features. In this paper, we propose an effective pairwise ranking model by leveraging clickthrough data of a Web search engine to address these two problems. We first construct large-scale training data by leveraging the query-title pairs derived from clickthrough data of a Web search engine. Then, we build a pairwise ranking model which employs a convolutional neural network to automatically learn relevant features. The proposed model can be easily trained with backpropagation to perform the ranking task. The experiments show that our method significantly outperforms several strong baselines.
MoreTranslated text
Key words
Relational Data Modeling,Knowledge Graph Embedding,Named Entity Recognition,Representation Learning,Topic Modeling
AI Read Science
Must-Reading Tree
Example
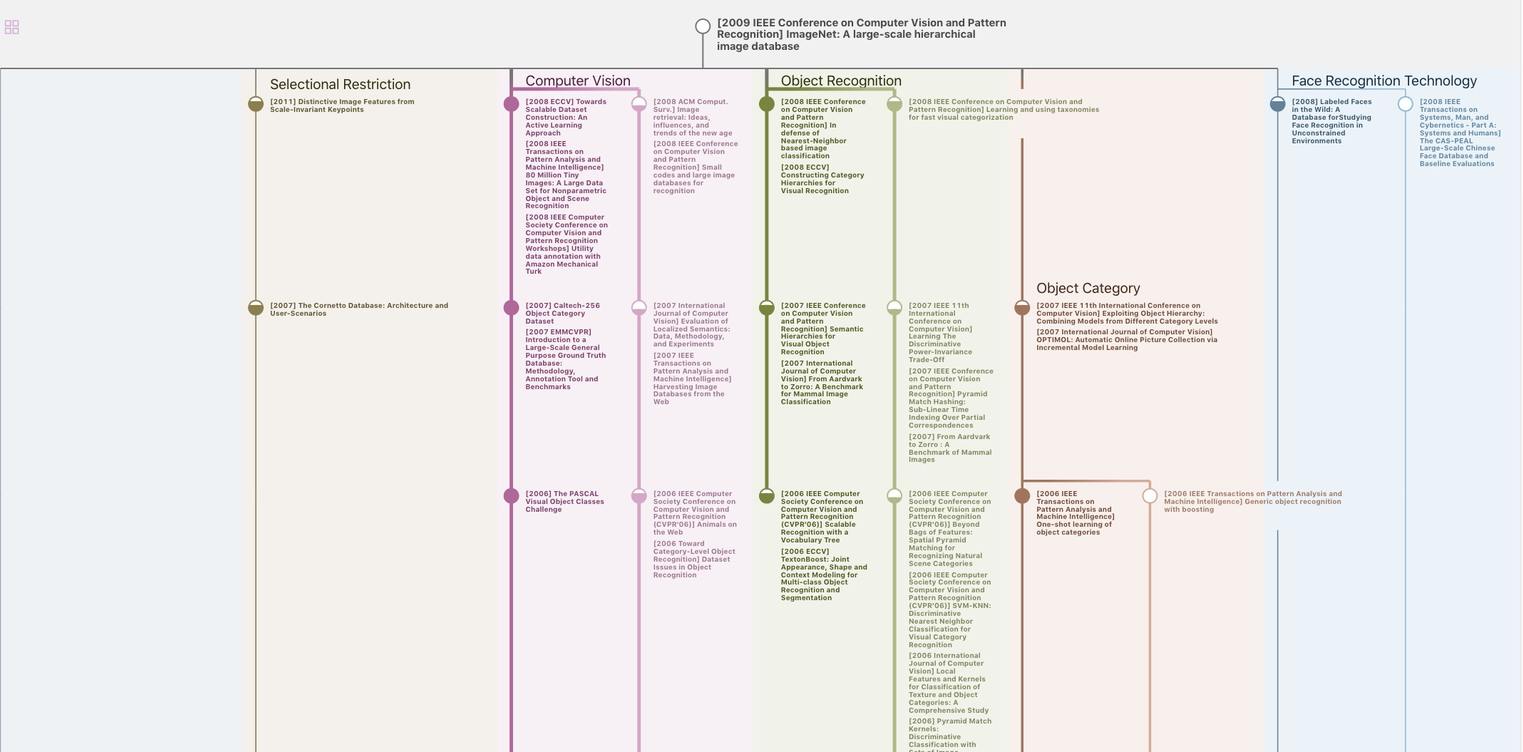
Generate MRT to find the research sequence of this paper
Chat Paper
Summary is being generated by the instructions you defined