Prior Knowledge Integration For Neural Machine Translation Using Posterior Regularization
PROCEEDINGS OF THE 55TH ANNUAL MEETING OF THE ASSOCIATION FOR COMPUTATIONAL LINGUISTICS (ACL 2017), VOL 1(2018)
摘要
Although neural machine translation has made significant progress recently, how to integrate multiple overlapping, arbitrary prior knowledge sources remains a challenge. In this work, we propose to use posterior regularization to provide a general framework for integrating prior knowledge into neural machine translation. We represent prior knowledge sources as features in a log-linear model, which guides the learning process of the neural translation model. Experiments on Chinese-English translation show that our approach leads to significant improvements.
更多查看译文
AI 理解论文
溯源树
样例
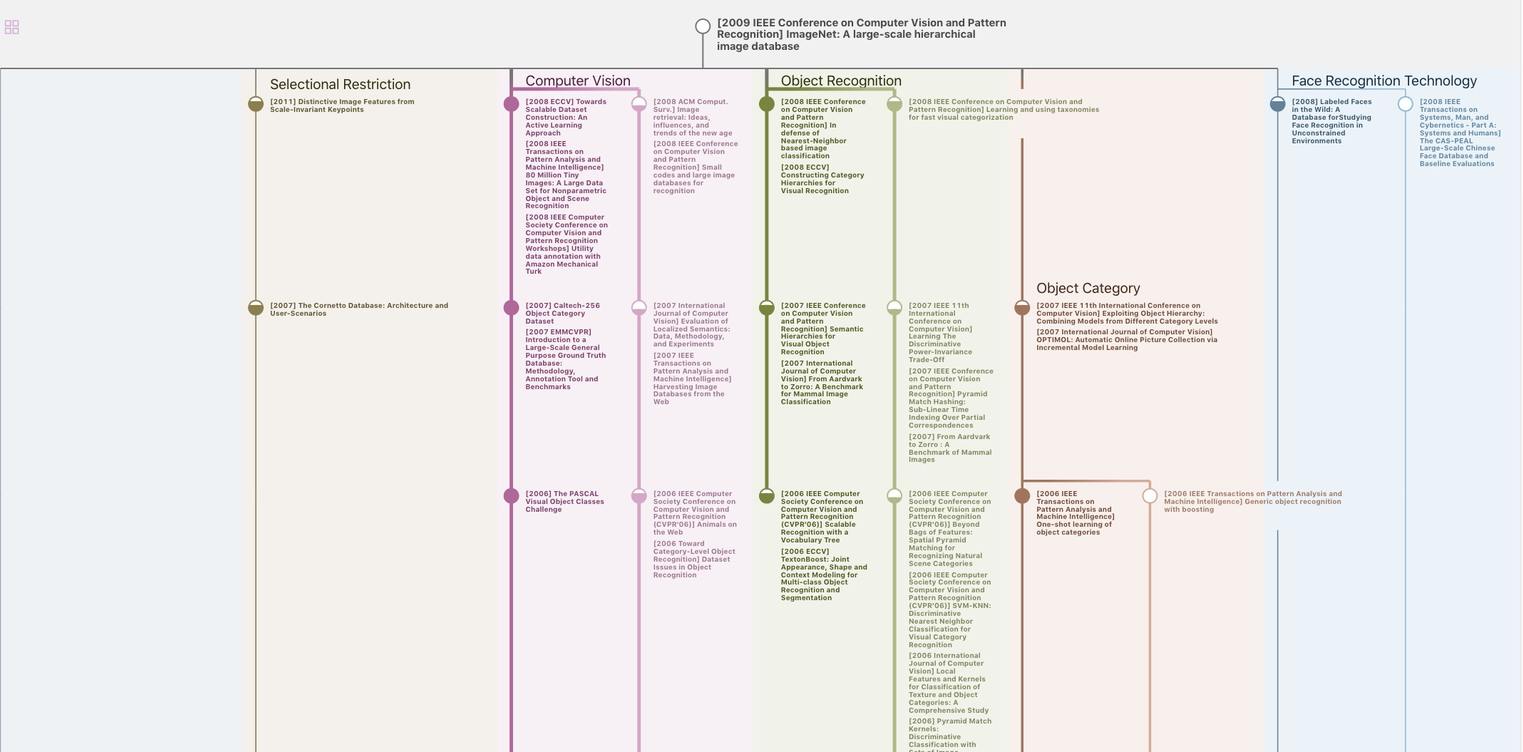
生成溯源树,研究论文发展脉络
Chat Paper
正在生成论文摘要