Prediction of amino acid side chain conformation using a deep neural network
arXiv: Biomolecules(2017)
摘要
A deep neural network based architecture was constructed to predict amino acid side chain conformation with unprecedented accuracy. Amino acid side chain conformation prediction is essential for protein homology modeling and protein design. Current widely-adopted methods use physics-based energy functions to evaluate side chain conformation. Here, using a deep neural network architecture without physics-based assumptions, we have demonstrated that side chain conformation prediction accuracy can be improved by more than 25 especially for aromatic residues compared with current standard methods. More strikingly, the prediction method presented here is robust enough to identify individual conformational outliers from high resolution structures in a protein data bank without providing its structural factors. We envisage that our amino acid side chain predictor could be used as a quality check step for future protein structure model validation and many other potential applications such as side chain assignment in Cryo-electron microscopy, crystallography model auto-building, protein folding and small molecule ligand docking.
更多查看译文
关键词
conformation,deep neural
AI 理解论文
溯源树
样例
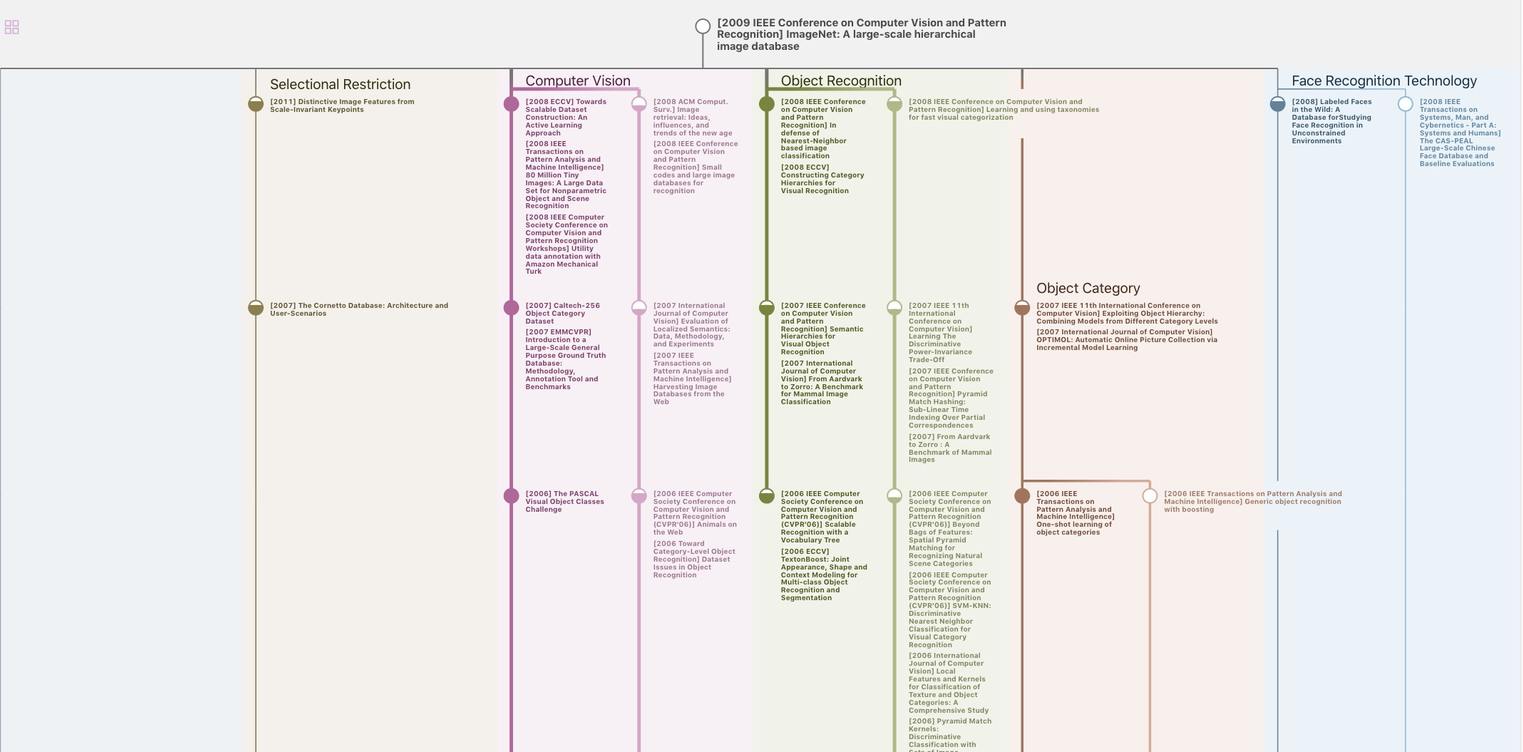
生成溯源树,研究论文发展脉络
Chat Paper
正在生成论文摘要