Exploiting the Tradeoff Between Program Accuracy and Soft-error Resiliency Overhead for Machine Learning Workloads.
CoRR(2017)
Key words
Soft Errors,Robustness,Fault Tolerance,Error Detection,Transient Faults
AI Read Science
Must-Reading Tree
Example
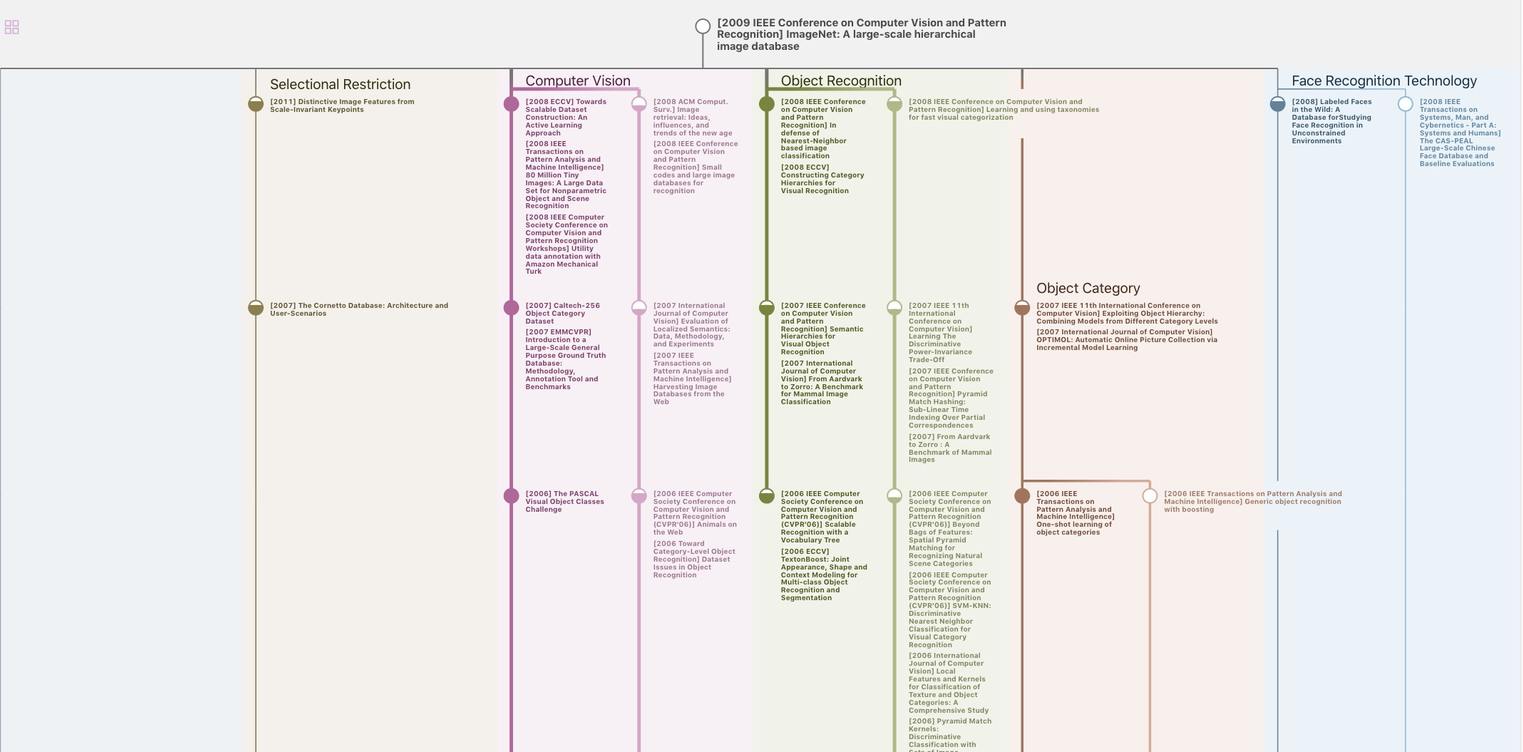
Generate MRT to find the research sequence of this paper
Chat Paper
Summary is being generated by the instructions you defined