Steering approaches to Pareto-optimal multiobjective reinforcement learning.
Neurocomputing(2017)
摘要
For reinforcement learning tasks with multiple objectives, it may be advantageous to learn stochastic or non-stationary policies. This paper investigates two novel algorithms for learning non-stationary policies which produce Pareto-optimal behaviour (w-steering and Q-steering), by extending prior work based on the concept of geometric steering. Empirical results demonstrate that both new algorithms offer substantial performance improvements over stationary deterministic policies, while Q-steering significantly outperforms w-steering when the agent has no information about recurrent states within the environment. It is further demonstrated that Q-steering can be used interactively by providing a human decision-maker with a visualisation of the Pareto front and allowing them to adjust the agent’s target point during learning. To demonstrate broader applicability, the use of Q-steering in combination with function approximation is also illustrated on a task involving control of local battery storage for a residential solar power system.
更多查看译文
关键词
Multiobjective reinforcement learning,Non-stationary policies,Geometric steering,Interactive reinforcement learning,Pareto optimality
AI 理解论文
溯源树
样例
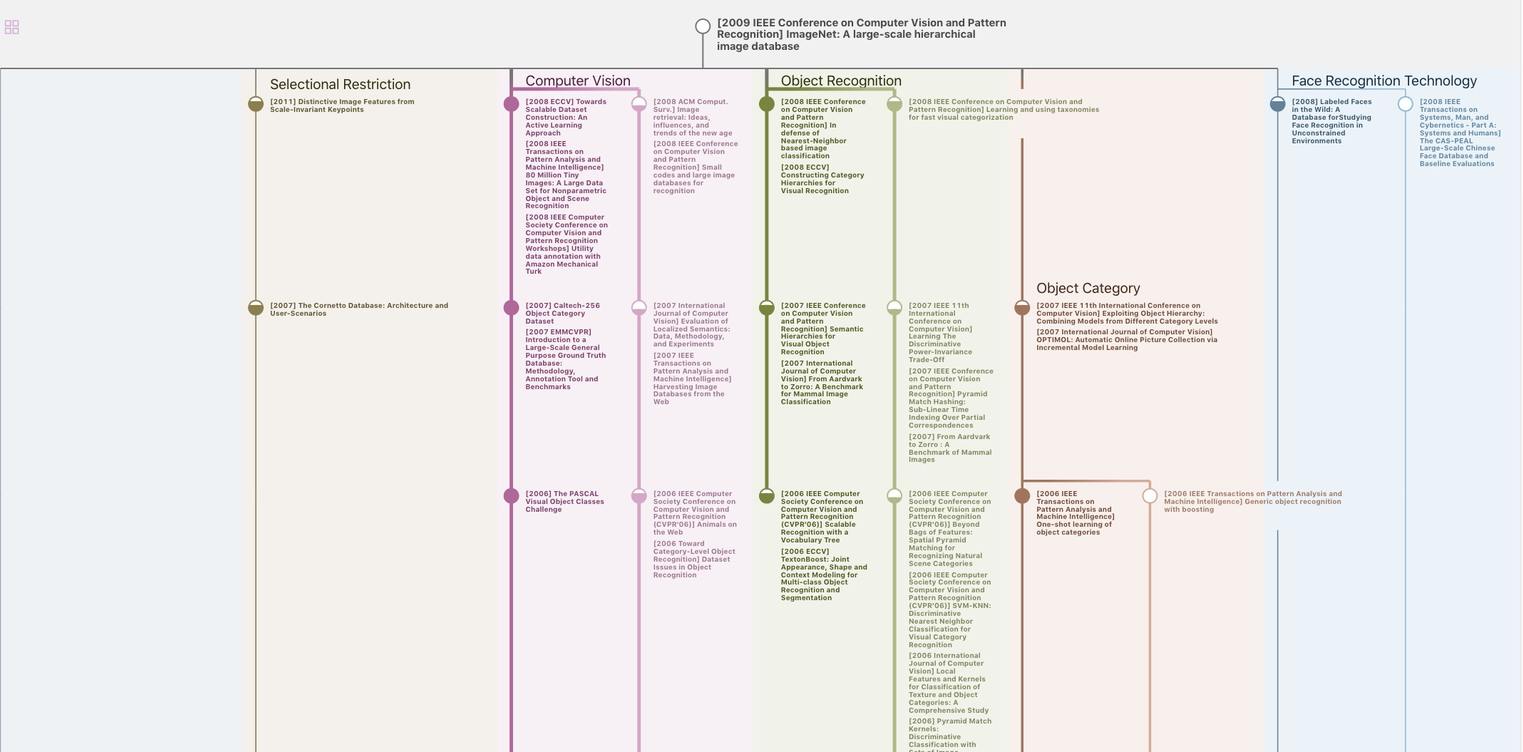
生成溯源树,研究论文发展脉络
Chat Paper
正在生成论文摘要