Speech enhancement under low SNR conditions via noise estimation using sparse and low-rank NMF with Kullback–Leibler divergence
IEEE/ACM Transactions on Audio, Speech and Language Processing (TASLP)(2015)
摘要
A key stage in speech enhancement is noise estimation which usually requires prior models for speech or noise or both. However, prior models can sometimes be difficult to obtain. In this paper, without any prior knowledge of speech and noise, sparse and low-rank nonnegative matrix factorization (NMF) with Kullback-Leibler divergence is proposed to noise and speech estimation by decomposing the input noisy magnitude spectrogram into a low-rank noise part and a sparse speech-like part. This initial unsupervised speech-noise estimation allows us to set a subsequent regularized version of NMF or convolutional NMF to reconstruct the noise and speech spectrogram, either by estimating a speech dictionary on the fly (categorized as unsupervised approaches) or by using a pre-trained speech dictionary on utterances with disjoint speakers (categorized as semi-supervised approaches). Information fusion was investigated by taking the geometric mean of the outputs from multiple enhancement algorithms. The performance of the algorithms were evaluated on five metrics (PESQ, SDR, SNR, STOI and OVERALL) by making experiments on TIMIT with fifteen noise types. The geometric means of the proposed unsupervised approaches outperformed spectral subtraction (SS), minimum mean square estimation (MMSE) under low input SNR conditions. All the proposed semi-supervised approaches showed superiority over SS and MMSE and also obtained better performance than the state-of-the-art algorithms which utilized a prior noise or speech dictionary under low SNR conditions.
更多查看译文
关键词
blockwise/convolutional nonnegative matrix factorization,sparse and low-rank decomposition,speech enhancement,nonnegative matrix factorization,hidden markov models,matrix decomposition,dictionaries,spectrogram,speech,kullback leibler divergence,matrix factorization,noise
AI 理解论文
溯源树
样例
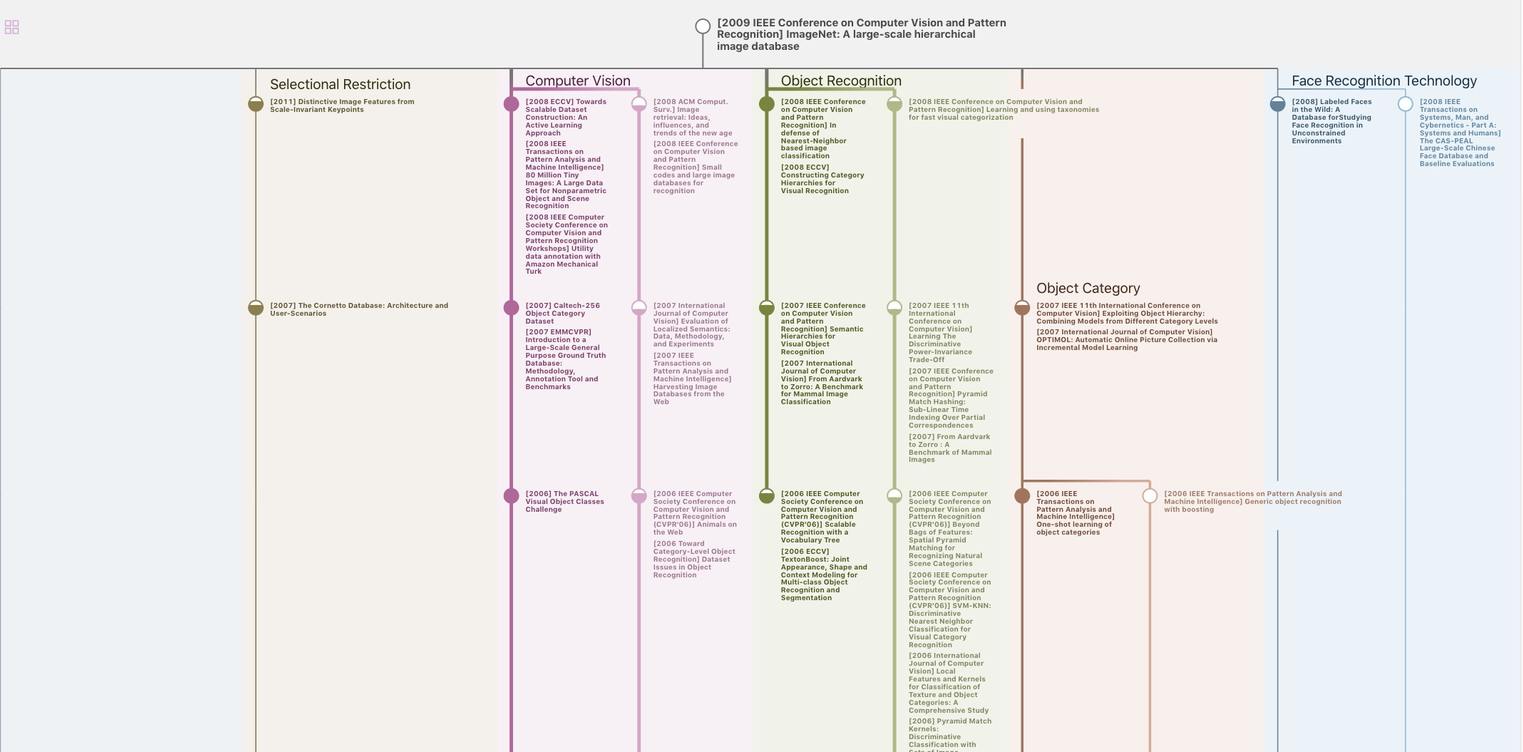
生成溯源树,研究论文发展脉络
Chat Paper
正在生成论文摘要