What Are You Known For?: Learning User Topical Profiles with Implicit and Explicit Footprints
SIGIR(2017)
摘要
User interests and expertise are valuable but often hidden resources on social media. For example, Twitter Lists and LinkedIn's Skill Tags provide a partial perspective on what users are known for (by aggregating crowd tagging knowledge), but the vast majority of users are untagged; their interests and expertise are essentially hidden from important applications such as personalized recommendation, community detection, and expert mining. A natural approach to overcome these limitations is to intelligently learn user topical profiles by exploiting information from multiple, heterogeneous footprints: for instance, Twitter users who post similar hashtags may have similar interests, and YouTube users who upvote the same videos may have similar preferences. And yet identifying \"similar\" users by exploiting similarity in such a footprint space often provides conflicting evidence, leading to poor-quality user profiles. In this paper, we propose a unified model for learning user topical profiles that simultaneously considers multiple footprints. We show how these footprints can be embedded in a generalized optimization framework that takes into account pairwise relations among all footprints for robustly learning user profiles. Through extensive experiments, we find the proposed model is capable of learning high-quality user topical profiles, and leads to a 10-15% improvement in precision and mean average error versus a cross-triadic factorization state-of-the-art baseline.
更多查看译文
关键词
Social Media, User Profile, User Behavior
AI 理解论文
溯源树
样例
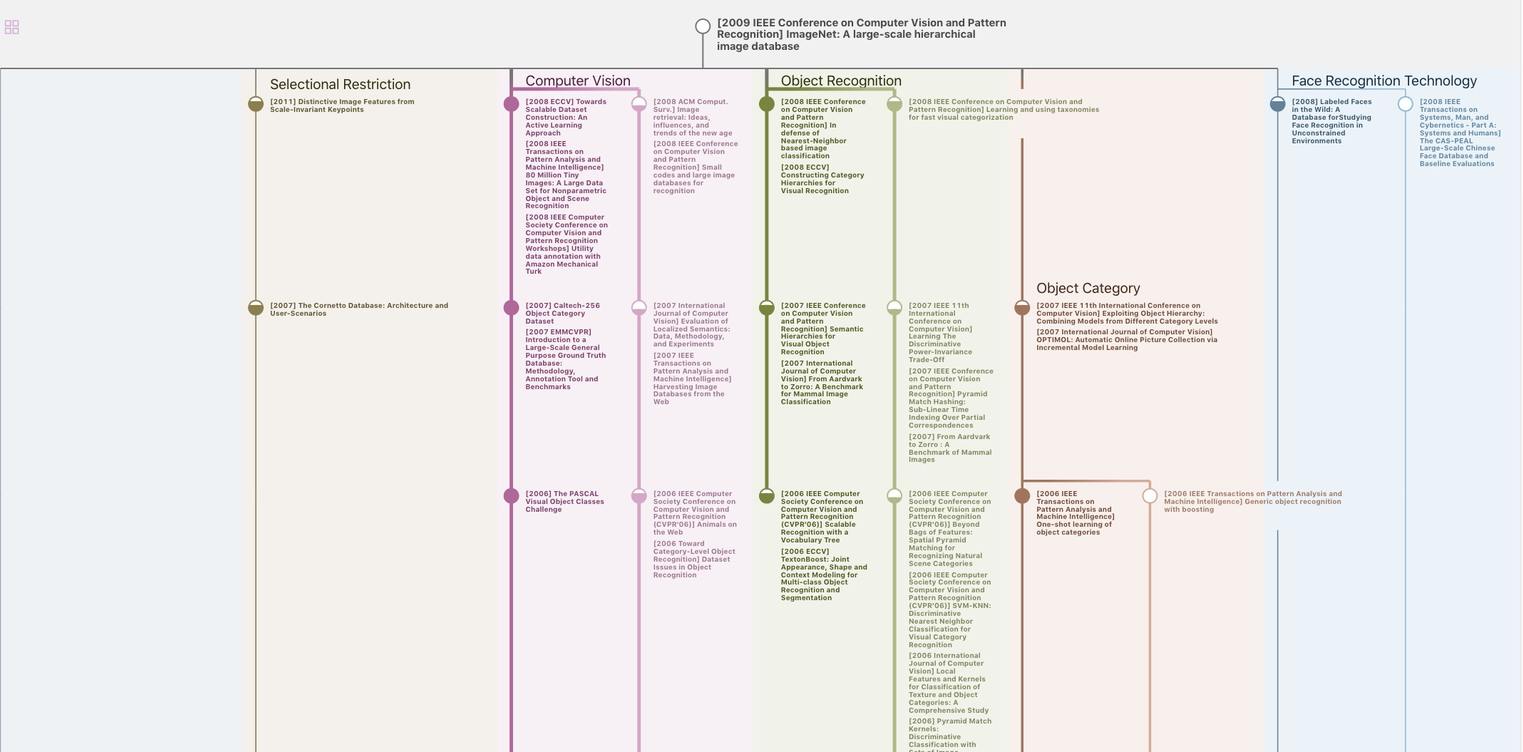
生成溯源树,研究论文发展脉络
Chat Paper
正在生成论文摘要