Ensemble-learning Approaches for Network Security and Anomaly Detection
Big-DAMA@SIGCOMM(2017)
摘要
The application of machine learning models to network security and anomaly detection problems has largely increased in the last decade; however, there is still no clear best-practice or silver bullet approach to address these problems in a general context. While deep-learning is today a major breakthrough in other domains, it is difficult to say which is the best model or category of models to address the detection of anomalous events in operational networks. We present a potential solution to fill this gap, exploring the application of ensemble learning models to network security and anomaly detection. We investigate different ensemble-learning approaches to enhance the detection of attacks and anomalies in network measurements, following a particularly promising model known as the Super Learner. The Super Learner performs asymptotically as well as the best possible weighted combination of the base learners, providing a very powerful approach to tackle multiple problems with the same technique. We test the proposed solution for two different problems, using the well-known MAWILab dataset for detection of network attacks, and a semi-synthetic dataset for detection of traffic anomalies in operational cellular networks. Results confirm that the Super Learner provides better results than any of the single models, opening the door for a generalization of a best-practice technique for these specific domains.
更多查看译文
关键词
Network Attacks,App Anomalies,Machine Learning,Ensemble Learning,Super Learner,High-Dimensional Data
AI 理解论文
溯源树
样例
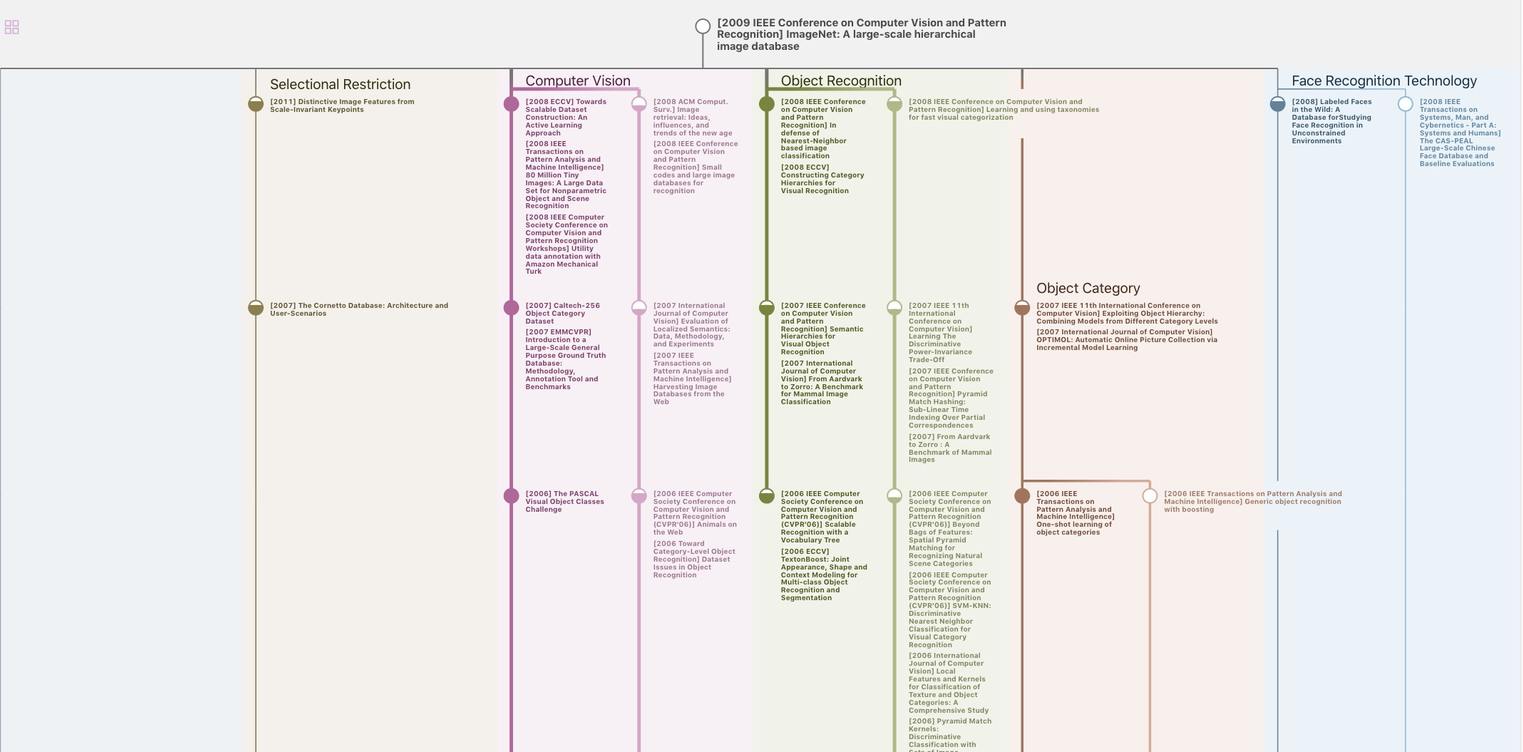
生成溯源树,研究论文发展脉络
Chat Paper
正在生成论文摘要