Folding: Why Good Models Sometimes Make Spurious Recommendations
RecSys(2017)
摘要
In recommender systems based on low-rank factorization of a partially observed user-item matrix, a common phenomenon that plagues many otherwise effective models is the interleaving of good and spurious recommendations in the top-K results. A single spurious recommendation can dramatically impact the perceived quality of a recommender system. Spurious recommendations do not result in serendipitous discoveries but rather cognitive dissonance. In this work, we investigate folding, a major contributing factor to spurious recommendations. Folding refers to the unintentional overlap of disparate groups of users and items in the low-rank embedding vector space, induced by improper handling of missing data. We formally define a metric that quantifies the severity of folding in a trained system, to assist in diagnosing its potential to make inappropriate recommendations. The folding metric complements existing information retrieval metrics that focus on the number of good recommendations and their ranks but ignore the impact of undesired recommendations. We motivate the folding metric definition on synthetic data and evaluate its effectiveness on both synthetic and real world datasets. In studying the relationship between the folding metric and other characteristics of recommender systems, we observe that optimizing for goodness metrics can lead to high folding and thus more spurious recommendations.
更多查看译文
关键词
collaborative filtering, matrix factorization, folding, evaluation metric, MNAR
AI 理解论文
溯源树
样例
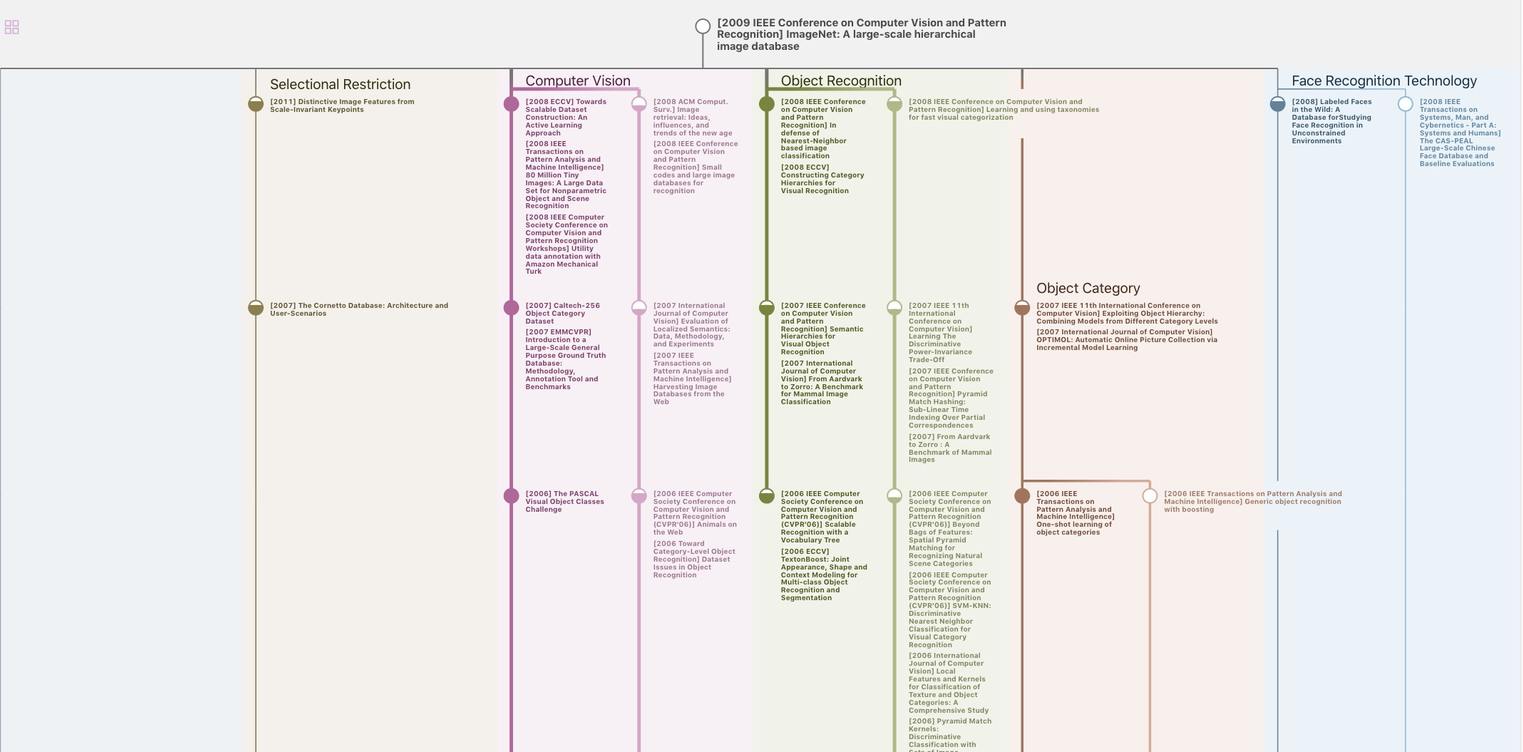
生成溯源树,研究论文发展脉络
Chat Paper
正在生成论文摘要