T P -Compilation for inference in probabilistic logic programs
International Journal of Approximate Reasoning(2016)
摘要
We propose T P -compilation, a new inference technique for probabilistic logic programs that is based on forward reasoning. T P -compilation proceeds incrementally in that it interleaves the knowledge compilation step for weighted model counting with forward reasoning on the logic program. This leads to a novel anytime algorithm that provides hard bounds on the inferred probabilities. The main difference with existing inference techniques for probabilistic logic programs is that these are a sequence of isolated transformations. Typically, these transformations include conversion of the ground program into an equivalent propositional formula and compilation of this formula into a more tractable target representation for weighted model counting. An empirical evaluation shows that T P -compilation effectively handles larger instances of complex or cyclic real-world problems than current sequential approaches, both for exact and anytime approximate inference. Furthermore, we show that T P -compilation is conducive to inference in dynamic domains as it supports efficient updates to the compiled model. A new inference technique for probabilistic logic programs.We interleave knowledge compilation with forward reasoning.Exact as well as anytime approximate inference.Our approach is conducive to inference in dynamic models.Empirical evaluation on various domains.
更多查看译文
关键词
Probabilistic inference,Knowledge compilation,Probabilistic logic programs,Dynamic relational models
AI 理解论文
溯源树
样例
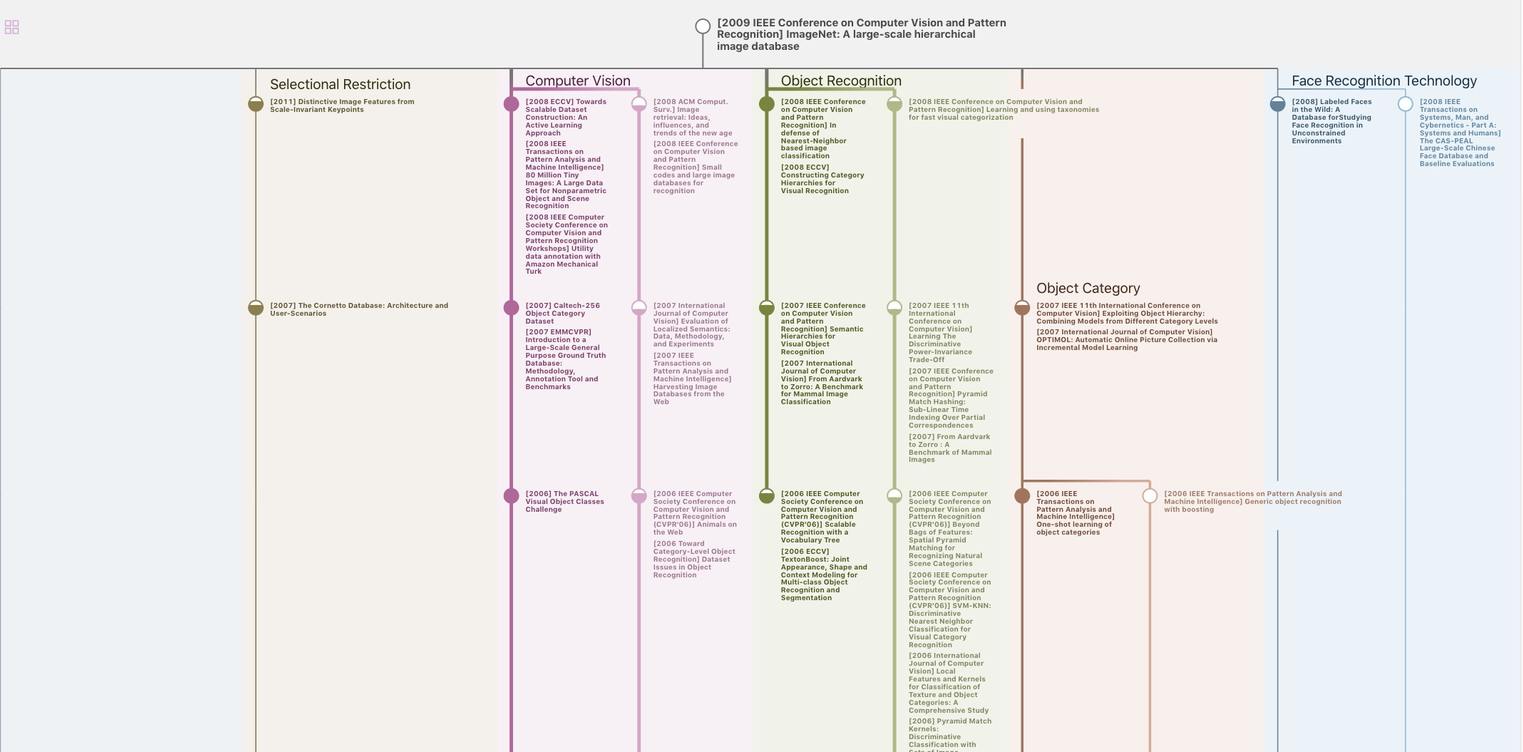
生成溯源树,研究论文发展脉络
Chat Paper
正在生成论文摘要