Online Adaptive Hidden Markov Model for Multi-Tracker Fusion.
Computer Vision and Image Understanding(2016)
摘要
We introduce a novel method for fusion of multiple trackers based on Hidden Markov Models.We propose a simple, and so far unused, unsupervised method for HMMs training in the context of tracking using a tunable feature-based detector with very low false positive rate-state-of-the-art performance on multiple public benchmarks and, additionally, on extensive collection of publicly available sequences. In this paper, we propose a novel method for visual object tracking called HMMTxD. The method fuses observations from complementary out-of-the box trackers and a detector by utilizing a hidden Markov model whose latent states correspond to a binary vector expressing the failure of individual trackers. The Markov model is trained in an unsupervised way, relying on an online learned detector to provide a source of tracker-independent information for a modified Baum- Welch algorithm that updates the model w.r.t. the partially annotated data. We show the effectiveness of the proposed method on combination of two and three tracking algorithms. The performance of HMMTxD is evaluated on two standard benchmarks (CVPR2013 and VOT) and on a rich collection of 77 publicly available sequences. The HMMTxD outperforms the state-of-the-art, often significantly, on all data-sets in almost all criteria.
更多查看译文
关键词
Visual tracking,On-line learning,Hidden markov model,Object detection
AI 理解论文
溯源树
样例
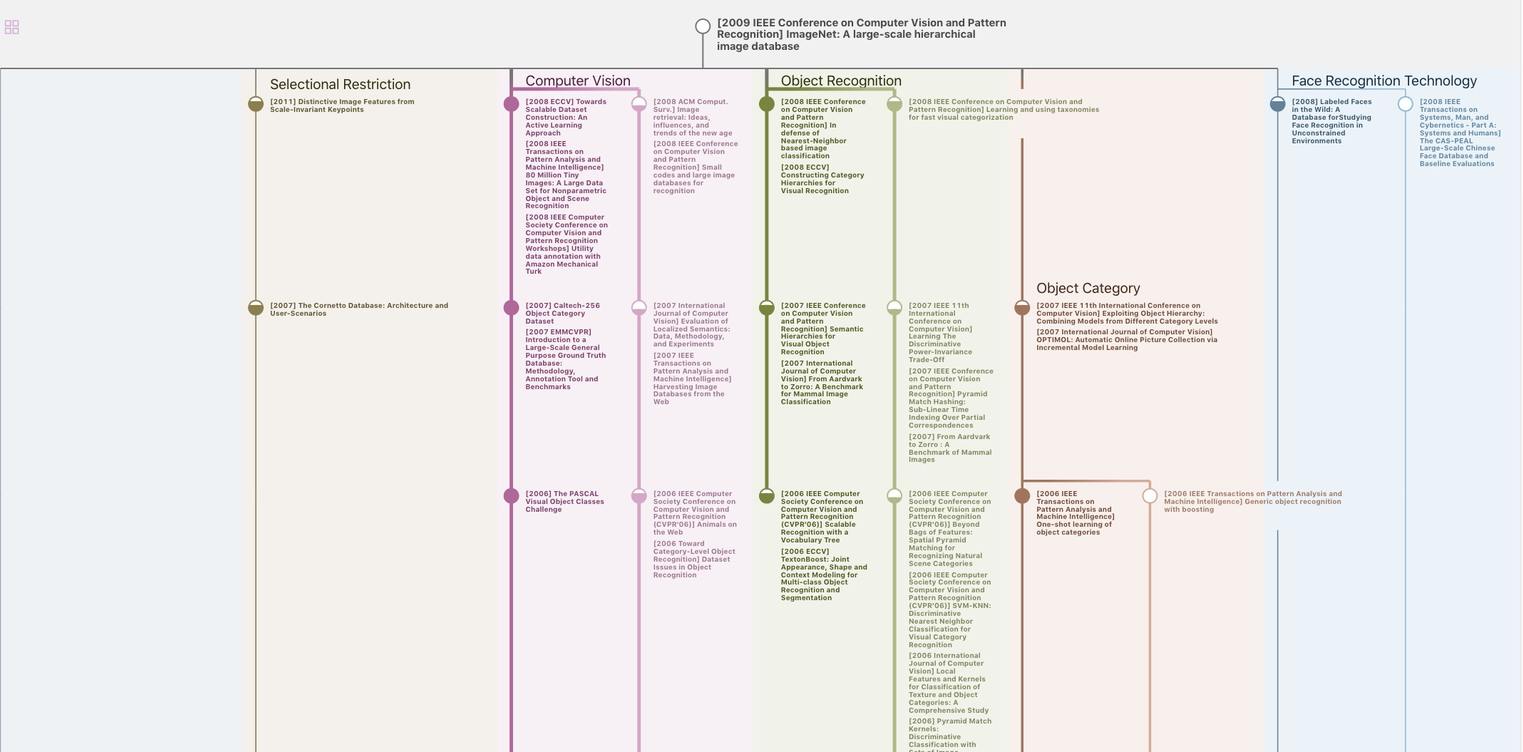
生成溯源树,研究论文发展脉络
Chat Paper
正在生成论文摘要