Residual Vs. Inception Vs. Classical Networks For Low-Resolution Face Recognition
IMAGE ANALYSIS, SCIA 2017, PT II(2017)
摘要
When analyzing surveillance footage, low-resolution face recognition is still a challenging task. While high-resolution face recognition experienced impressive improvements by Convolutional Neural Network (CNN) approaches, the benefit to low-resolution face recognition remains unclear as only few work has been done in this area. This paper adapts three popular high-resolution CNN designs to the low-resolution (LR) domain to find the most suitable architecture. Namely, the classical AlexNet/VGG architecture, Google's inception architecture and Microsoft's residual architecture are considered. While the inception and residual concept have been proven to be useful for very deep networks, it is shown in our case that shallower networks than for high-resolution recognition are sufficient. This leads to an advantage of the classical network architecture. Final evaluation on a downscaled version of the public YouTube Faces Database indicates a comparable performance to the high-resolution domain. Results with faces extracted from the SoBiS surveillance dataset indicate a superior performance of the trained networks in the LR domain.
更多查看译文
AI 理解论文
溯源树
样例
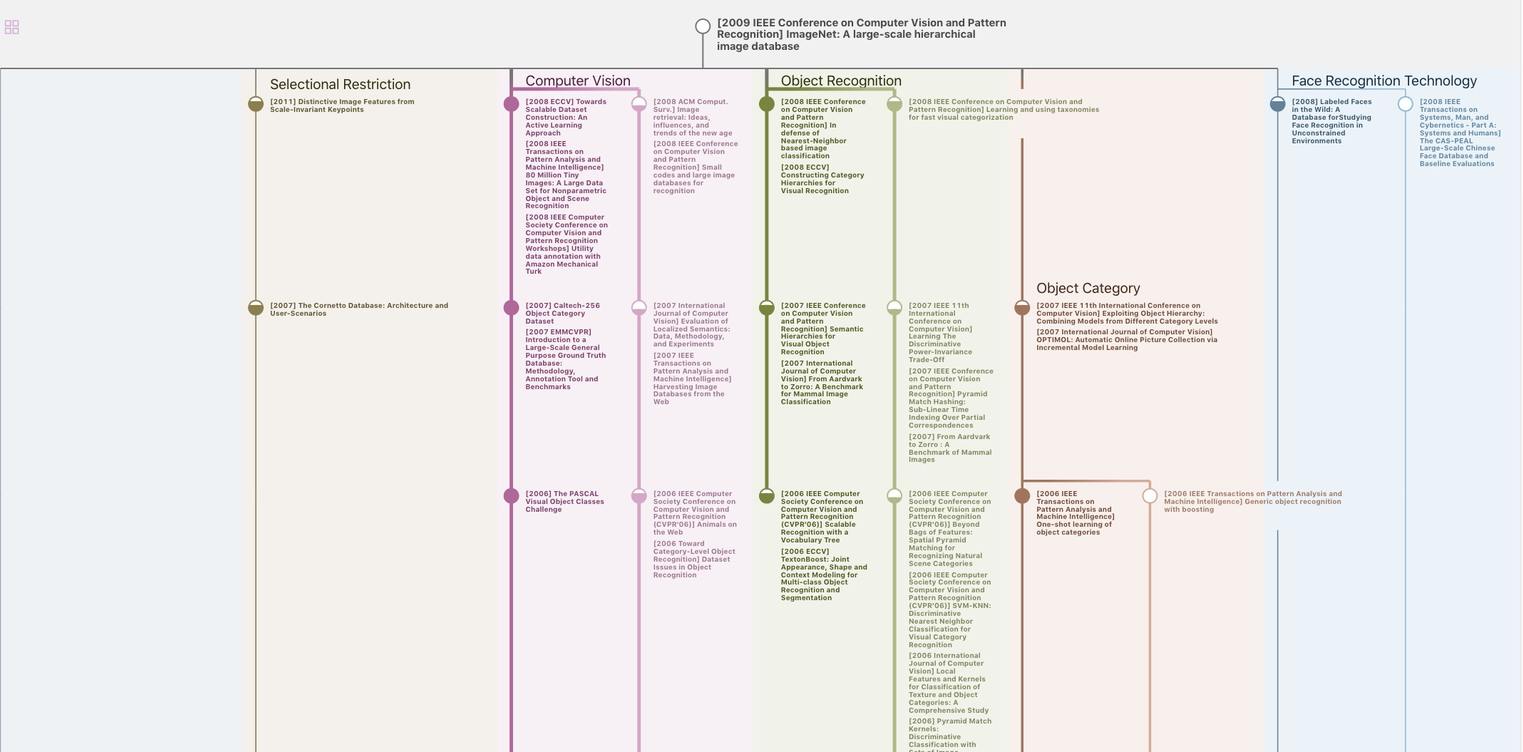
生成溯源树,研究论文发展脉络
Chat Paper
正在生成论文摘要