Cascaded Fully Convolutional Networks For Automatic Prenatal Ultrasound Image Segmentation
2017 IEEE 14TH INTERNATIONAL SYMPOSIUM ON BIOMEDICAL IMAGING (ISBI 2017)(2017)
摘要
Computerized prenatal ultrasound (US) image segmentation methods can greatly improve the efficiency and objectiveness of the biometry interpretation. However, the boundary incompleteness and ambiguity in US images hinder the automatic solutions severely. In this paper, we propose a cascaded framework for fully automatic US image segmentation. A customized Fully Convolutional Network (FCN) was utilized to exploit feature extractions from multiple visual scales and distinguish the anatomy with a dense prediction map. To enhance the local spatial consistency of the prediction map, we further implant the FCN core into an Auto-Context scheme. By modifying the join operator in traditional Auto-Context scheme from parallelization to summation, our framework gains extra considerable improvement. We demonstrate the efficacy of our method on two challenging datasets: fetal head and abdomen US images. Extensive experimental results show that our method can bridge severe boundary incompleteness and achieves the best segmentation accuracy when compared with state-of-the-art methods.
更多查看译文
关键词
Prenatal ultrasound, image segmentation, fully convolutional networks, auto-context, join operator
AI 理解论文
溯源树
样例
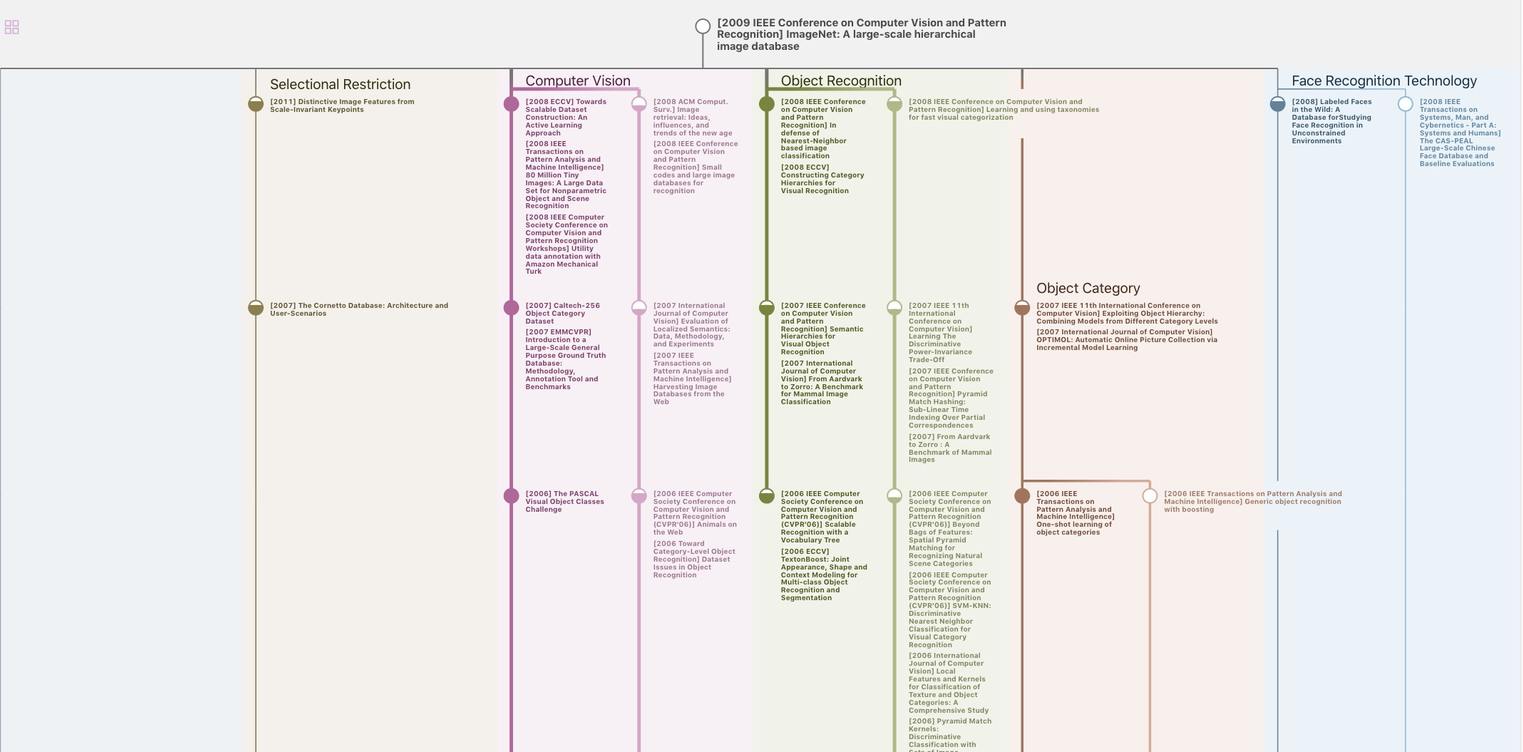
生成溯源树,研究论文发展脉络
Chat Paper
正在生成论文摘要