Variable Kernel Density Estimation In High-Dimensional Feature Spaces
THIRTY-FIRST AAAI CONFERENCE ON ARTIFICIAL INTELLIGENCE(2017)
摘要
Estimating the joint probability density function of a dataset is a central task in many machine learning applications. In this work we address the fundamental problem of kernel bandwidth estimation for variable kernel density estimation in high-dimensional feature spaces. We derive a variable kernel bandwidth estimator by minimizing the leave-one-out entropy objective function and show that this estimator is capable of performing estimation in high-dimensional feature spaces with great success. We compare the performance of this estimator to state-of-the art maximum likelihood estimators on a number of representative high-dimensional machine learning tasks and show that the newly introduced minimum leave-one-out entropy estimator performs optimally on a number of high dimensional datasets considered.
更多查看译文
AI 理解论文
溯源树
样例
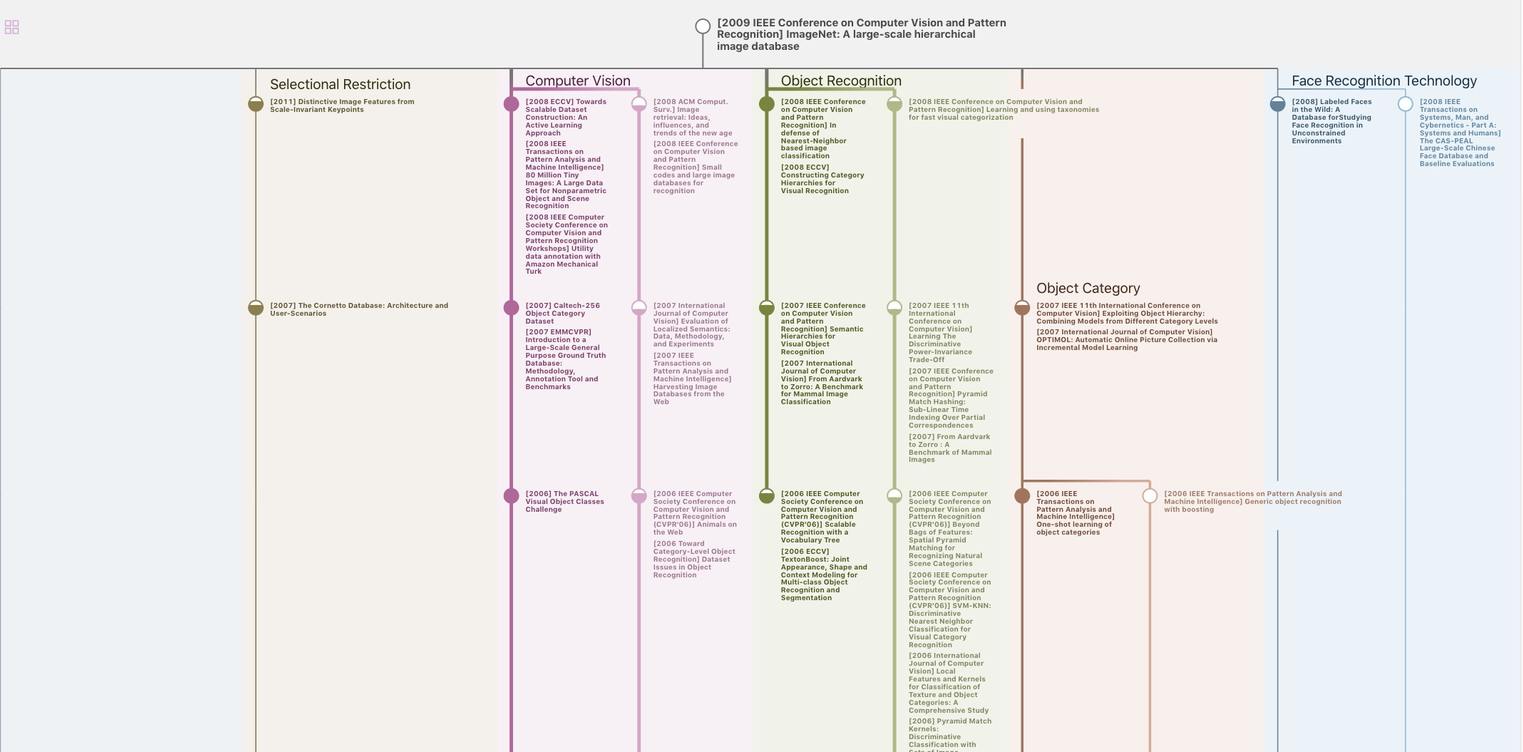
生成溯源树,研究论文发展脉络
Chat Paper
正在生成论文摘要