Structured Embedding Via Pairwise Relations And Long-Range Interactions In Knowledge Base
PROCEEDINGS OF THE TWENTY-NINTH AAAI CONFERENCE ON ARTIFICIAL INTELLIGENCE(2015)
摘要
We consider the problem of embedding entities and relations of knowledge bases into low-dimensional continuous vector spaces (distributed representations). Unlike most existing approaches, which are primarily efficient for modelling pairwise relations between entities, we attempt to explicitly model both pairwise relations and long-range interactions between entities, by interpreting them as linear operators on the low-dimensional embeddings of the entities. Therefore, in this paper we introduces path ranking to capture the long-range interactions of knowledge graph and at the same time preserve the pairwise relations of knowledge graph; we call it structured embedding via pairwise relation and long-range interactions (referred to as SePLi). Comparing with the-state-of-the-art models, SePLi achieves better performances of embeddings.
更多查看译文
AI 理解论文
溯源树
样例
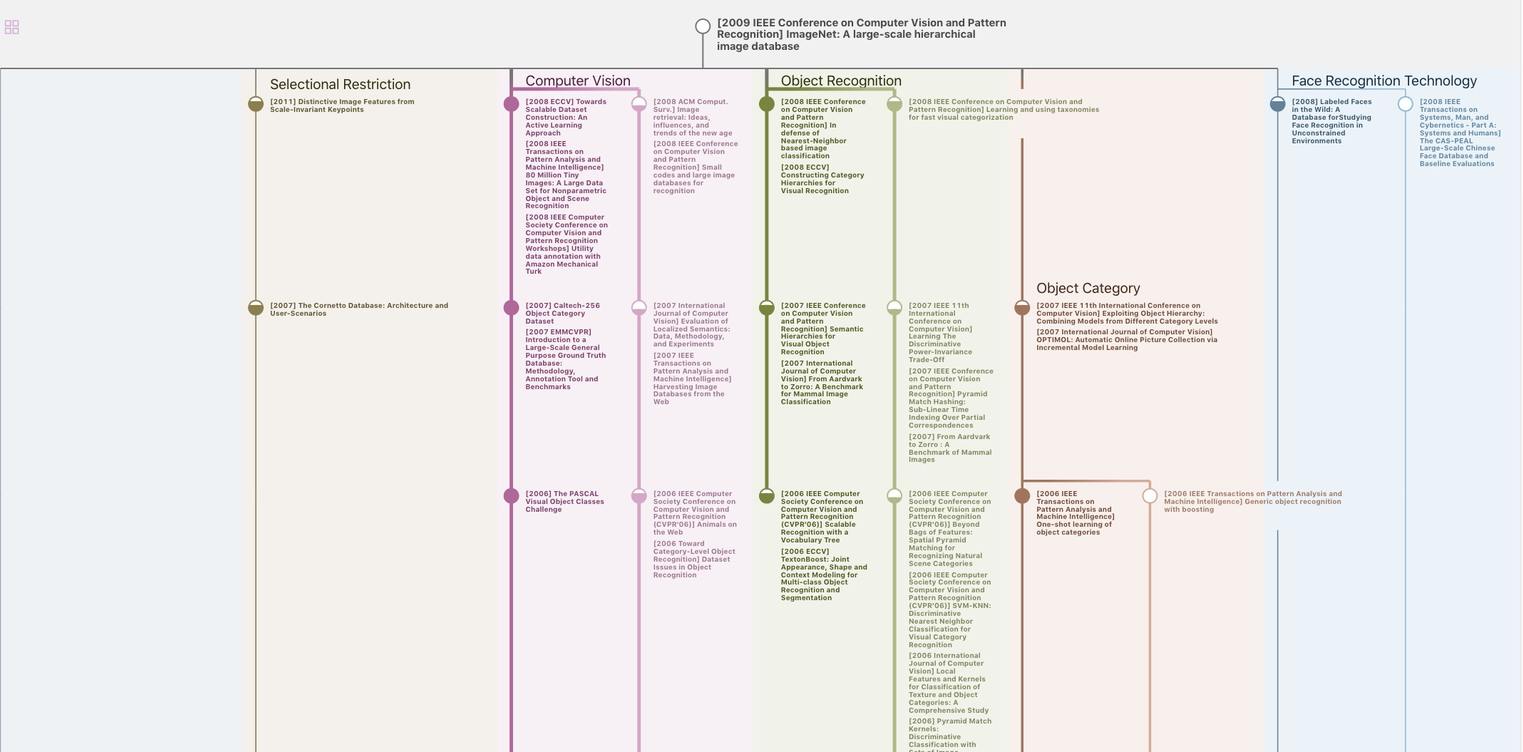
生成溯源树,研究论文发展脉络
Chat Paper
正在生成论文摘要