Switching Linear Inverse-Regression Model For Tracking Head Pose
2017 IEEE WINTER CONFERENCE ON APPLICATIONS OF COMPUTER VISION (WACV 2017)(2017)
摘要
We propose to estimate the head-pose angles (pitch, yaw, and roll) by simultaneously predicting the pose parameters from observed high-dimensional feature vectors, and tracking these parameters over time. This is achieved by embedding a Gaussian mixture of linear inverse-regression model into a dynamic Bayesian model. The use of a switching Kalman filter (SKF) enables a principled way of carrying out this embedding. The SKF governs the temporal predictive distribution of the pose parameters (modeled as continuous latent variables) conditioned by the discrete variables associated with the mixture of linear inverse-regression formulation. We formally derive the equations of the proposed switching linear regression model, we propose an approximation that is both identifiable and computationally tractable, we design an EM procedure to estimate the SKF parameters in closed-form, and we carry out experiments and comparisons with other methods using recently released datasets.
更多查看译文
关键词
linear inverse-regression model,head pose tracking,pose estimation,high-dimensional feature vectors,Gaussian mixture,dynamic Bayesian model,switching Kalman filter,SKF,switching linear regression model
AI 理解论文
溯源树
样例
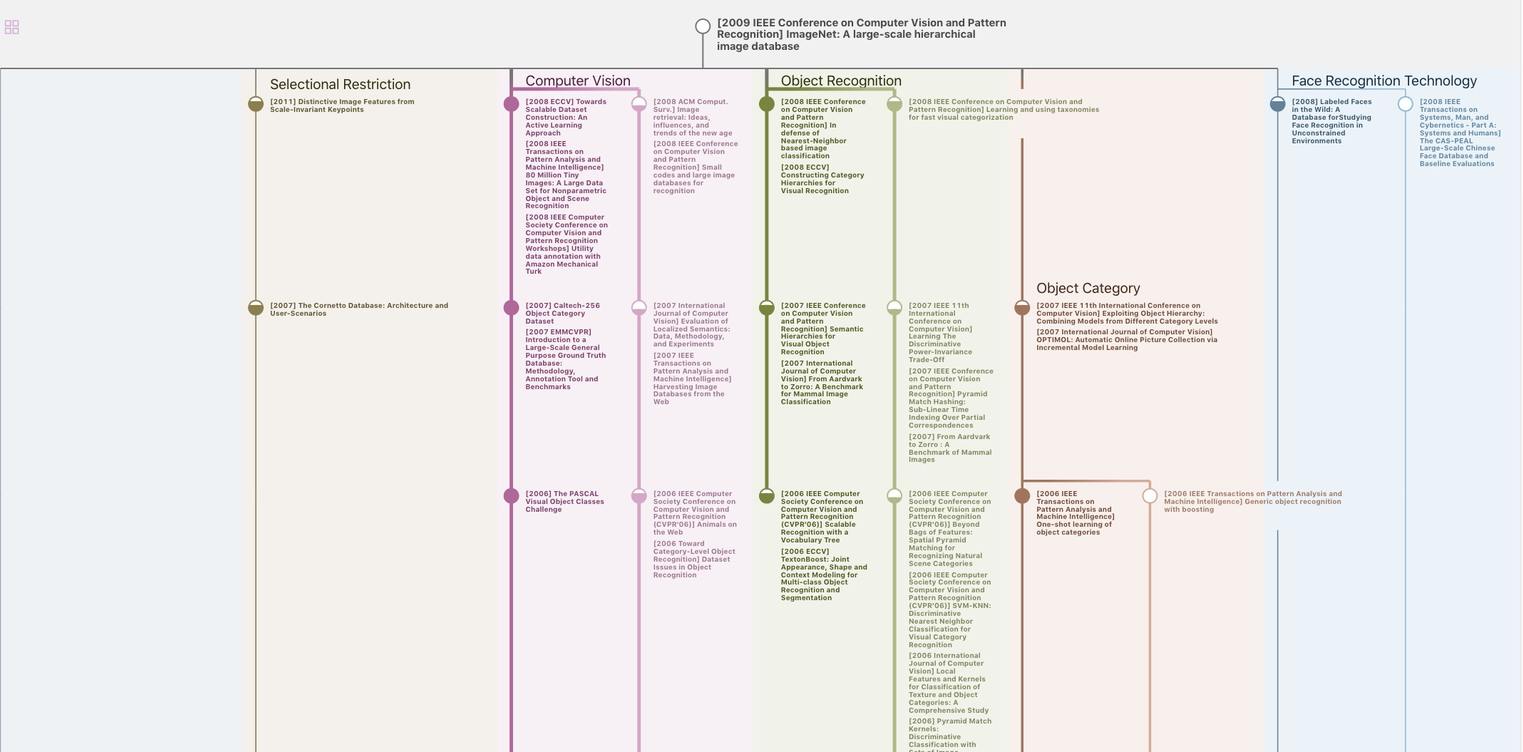
生成溯源树,研究论文发展脉络
Chat Paper
正在生成论文摘要