Deep Multi-Modal Vehicle Detection In Aerial Isr Imagery
2017 IEEE WINTER CONFERENCE ON APPLICATIONS OF COMPUTER VISION (WACV 2017)(2017)
摘要
Since the introduction of deep convolutional neural networks (CNNs), object detection in imagery has witnessed substantial breakthroughs in state-of-the-art performance. The defense community utilizes overhead image sensors that acquire large field-of-view aerial imagery in various bands of the electromagnetic spectrum, which is then exploited for various applications, including the detection and localization of man-made objects. In this work, we utilize a recent state-of-the art object detection algorithm, faster R-CNN, to train a deep CNN for vehicle detection in multimodal imagery. We utilize the vehicle detection in aerial imagery (VEDAI) dataset, which contains overhead imagery that is representative of an ISR setting. Our contribution includes modification of key parameters in the faster R-CNN algorithm for this setting where the objects of interest are spatially small, occupying less than 1.5 x 10(-3) of the total image pixels. Our experiments show that (1) an appropriately trained deep CNN leads to average precision rates above 93% on vehicle detection, and (2) transfer learning between imagery modalities is possible, yielding average precision rates above 90% in the absence of fine-tuning.
更多查看译文
关键词
deep multimodal vehicle detection,aerial ISR Imagery,deep convolutional neural networks,CNN,defense community,image sensors,electromagnetic spectrum,object detection algorithm,vehicle detection in aerial imagery,VEDAI dataset,R-CNN algorithm,image pixels
AI 理解论文
溯源树
样例
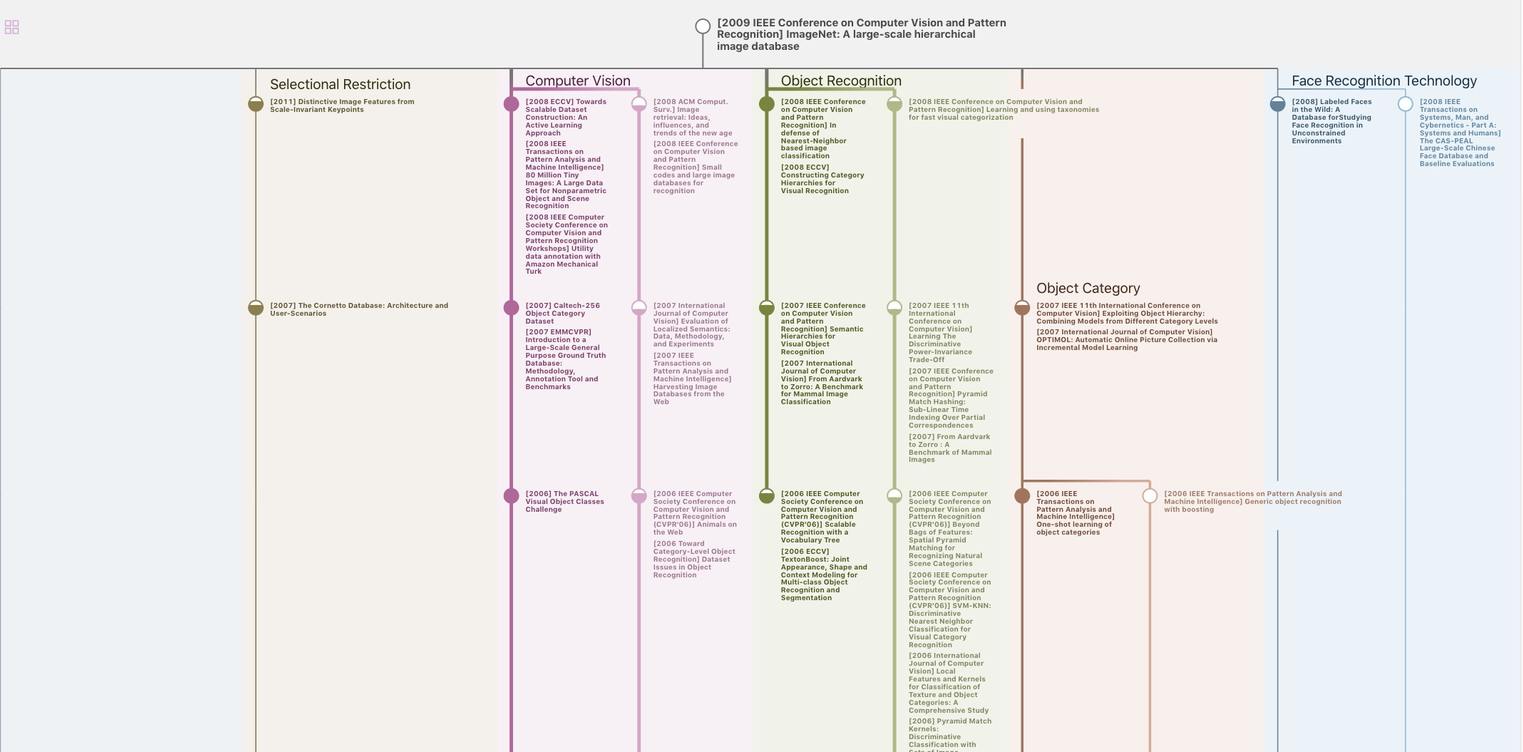
生成溯源树,研究论文发展脉络
Chat Paper
正在生成论文摘要