Learning from Semantically Dependent Multi-Tasks
2017 INTERNATIONAL JOINT CONFERENCE ON NEURAL NETWORKS (IJCNN)(2017)
摘要
We consider a different setting from regular multitask learning, where data from different tasks share no common instances and no common feature dictionary, while the features can be semantically correlated and all tasks share the same class space. For example, in the two tasks of identifying terrorism information from English news and Arabic news respectively, one associated dataset could be news from Cable News Network (CNN), and the other be news crawled from websites of Arabic countries. Intuitively, these two tasks could help each other, although they share no common feature space. This new setting has brought obstacles to traditional multi-task learning algorithms and multi-view learning algorithms. We argue that these different data sources can be co-trained together by exploring the latent semantics among them. To this end, we propose a new graphical model based on sparse Gaussian Conditional Random Fields (GCRF) and Hilbert-Schmidt Independence Criterion (HSIC). In additional to output the prediction accuracy for each single task, it can also model (1) the dependency between the latent feature spaces of different tasks, (2) the dependency of the category spaces, and (3) the dependency between the latent feature space and the category space in each task. To make the model inference effective, we have provided an efficient variational EM algorithm. Experiments on both synthetic data sets and real-world data sets have indicated the feasibility and effectiveness of the proposed framework.
更多查看译文
关键词
semantically dependent multitasks,multitask learning,multiview learning,latent semantics,graphical model,sparse Gaussian conditional random fields,GCRF,Hilbert-Schmidt independence criterion,HSIC,latent feature spaces,category spaces dependency,variational EM algorithm
AI 理解论文
溯源树
样例
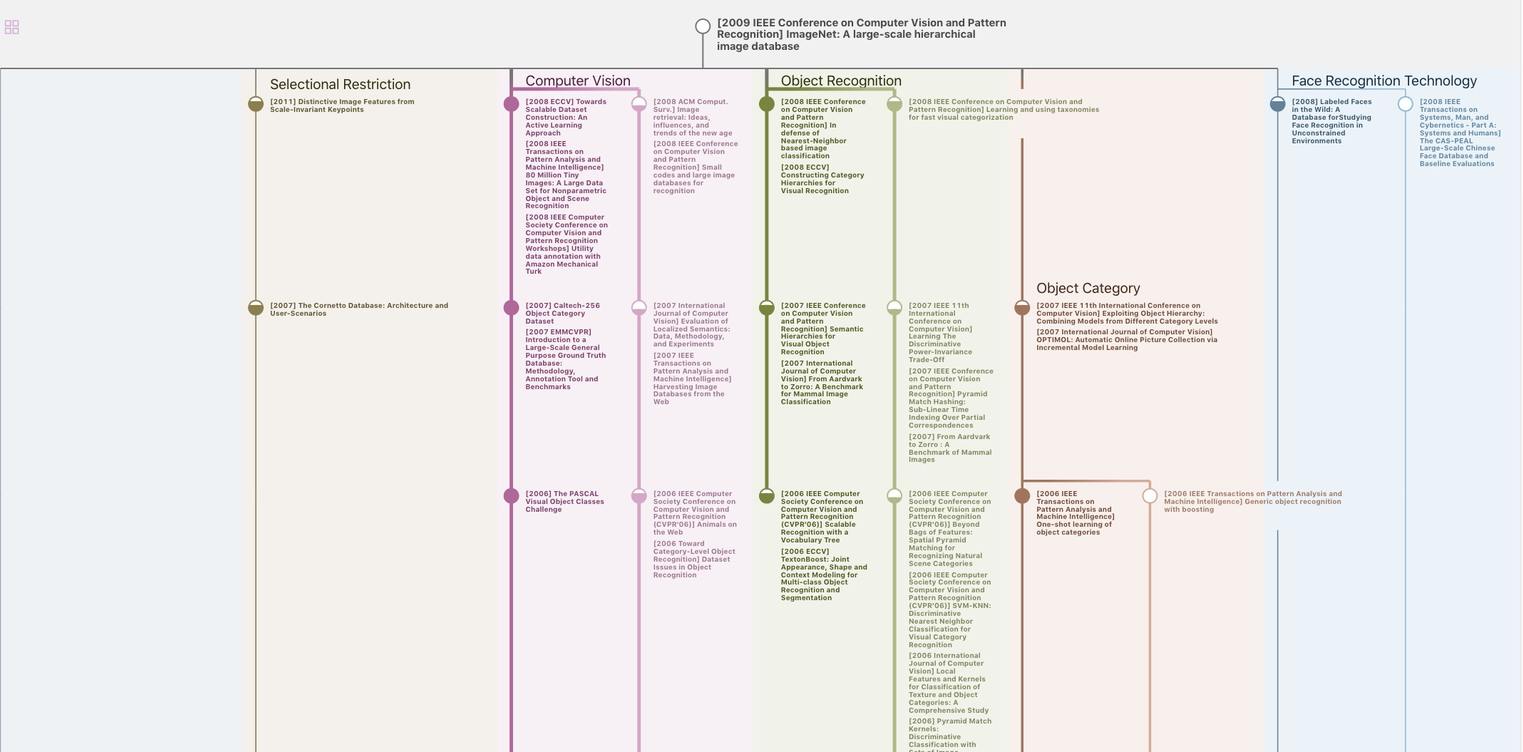
生成溯源树,研究论文发展脉络
Chat Paper
正在生成论文摘要