A Weighted-Resampling Based Transfer Learning Algorithm
2017 INTERNATIONAL JOINT CONFERENCE ON NEURAL NETWORKS (IJCNN)(2017)
摘要
Transfer learning has attracted more and more attention, and many scholars proposed some useful strategies. Boosting is the main strategy for transfer learning. In boosting, resampling is preferred over reweighting, and it can be applied to any base learner. In this paper, we propose a weighted-resampling method for transfer learning, called TrResampling. Firstly, resampling is applied to the data with heaven weight in the source domain, and the resampled data is used with the target data as the training data to build a classifier. Then the TrAdaBoost algorithm is used to adjust the weights of source data and target data. We discuss Decision Tree, Naive Bayes, and SVM as the base learner in TrResampling, and choose the suitable for TrResampling. In order to illustrate the performance of the proposed algorithm, we compare TrResampling with the state-of-the-art algorithm TrAdaBoost and the base learner Decision Tree, experimental results on UCI data sets indicate that TrResampling is superior to TrAdaBoost and Decision Tree on many data sets.
更多查看译文
关键词
weighted-resampling based transfer learning algorithm,TrResampling,TrAdaBoost algorithm,decision tree,naive Bayes,SVM,UCI data sets
AI 理解论文
溯源树
样例
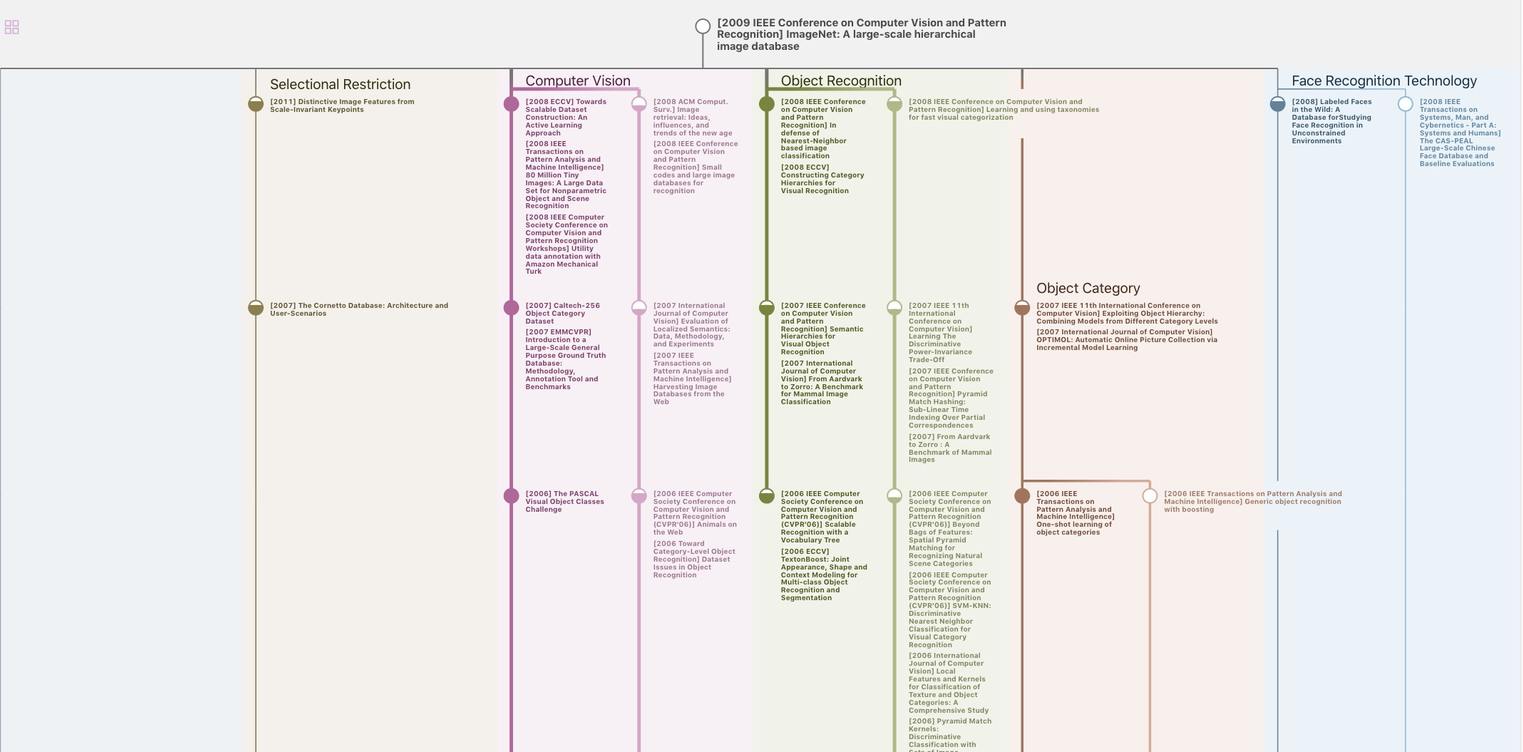
生成溯源树,研究论文发展脉络
Chat Paper
正在生成论文摘要