Deep Learning Through Evolution: A Hybrid Approach To Scheduling In A Dynamic Environment
2017 INTERNATIONAL JOINT CONFERENCE ON NEURAL NETWORKS (IJCNN)(2017)
摘要
Genetic Algorithms (GAs) have been shown to be a very effective optimisation tool on a wide variety of problems. However, they are not without their drawbacks. GAs require time to run, and evolve a bespoke solution to the desired problem in real time. This requirement can prove to be prohibitive in a high-frequency dynamic environment where on-line training time is limited. Neural Networks (NNs) on the other hand can be trained at length off-line, before being deployed on-line, allowing for fast generation of solutions on demand. This study presents a hybrid approach to time-frame scheduling in a high frequency domain. A GA approach is used to generate a dataset of optimised human-competitive solutions. Deep Learning is then deployed to extract the underlying model within the GA, enabling fast optimisation on unseen data. This hybrid approach allows for NNs to generate GA-quality schedules on-line, almost 100 times faster than running the GA.
更多查看译文
关键词
deep learning,dynamic environment,Genetic Algorithms,GA,optimisation tool,online training time,Neural Networks,NN,time-frame scheduling,high frequency domain,optimised human-competitive solutions
AI 理解论文
溯源树
样例
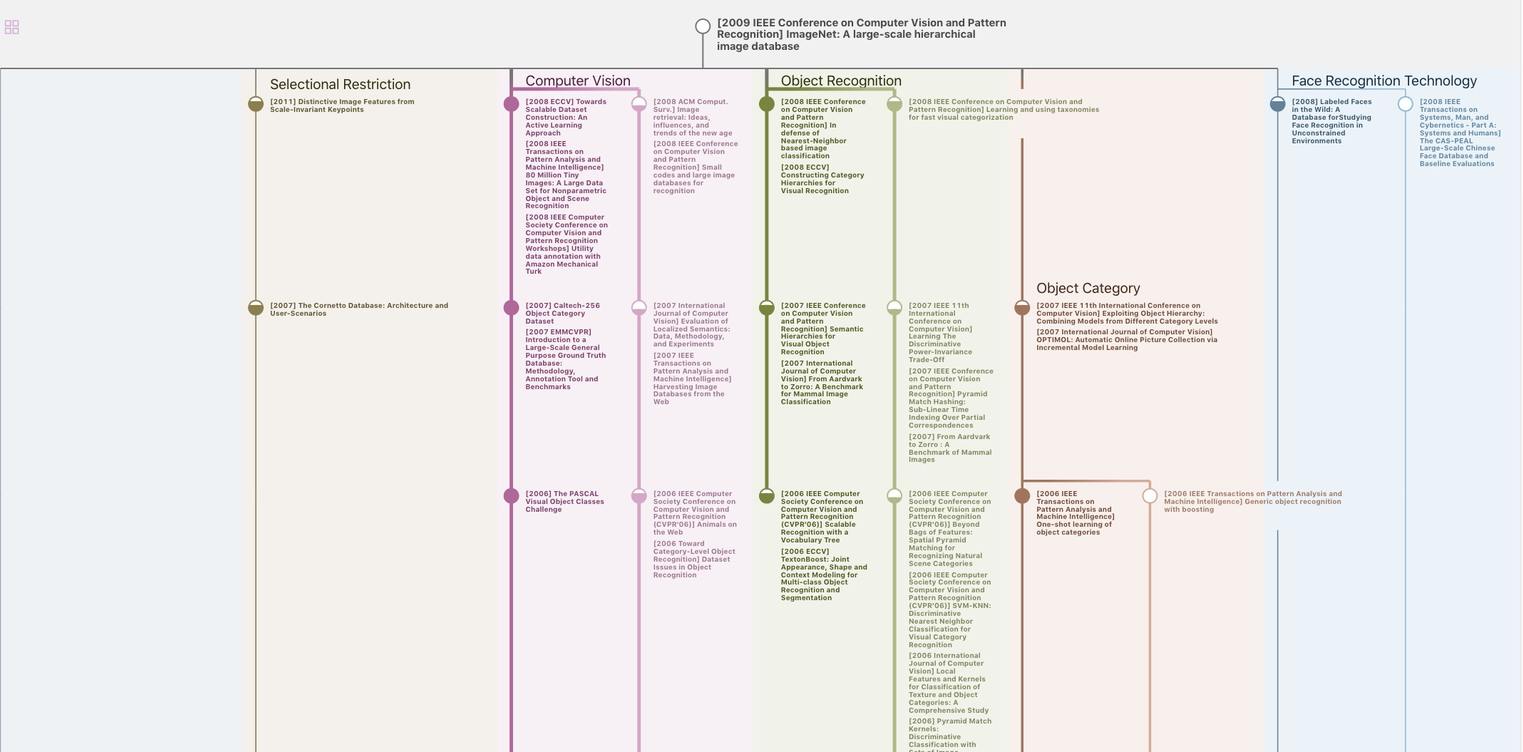
生成溯源树,研究论文发展脉络
Chat Paper
正在生成论文摘要