Bayesian Optimization For Conditional Hyperparameter Spaces
2017 INTERNATIONAL JOINT CONFERENCE ON NEURAL NETWORKS (IJCNN)(2017)
摘要
Hyperparameter optimization is now widely applied to tune the hyperparameters of learning algorithms. The hyperparameters can have structure, resulting in hyperparameters depending on conditions, or on the values of other hyperparameters. We target the problem of combined algorithm selection and hyperparameter optimization, which includes at least one conditional hyperparameter: the choice of the learning algorithm. In this work, we show that Bayesian optimization with Gaussian processes can be used for the optimization of conditional spaces with the injection of knowledge concerning conditions in the kernel. We propose and examine the behavior of two kernels, a conditional kernel which forces the similarity of two samples from different condition branches to be zero, and the Laplace kernel, based on similarities with Mondrian processes and random forests. We show the benefit of using such kernels, as well as proper imputation of inactive hyperparameters, on a benchmark of scikit-learn models.
更多查看译文
关键词
Bayesian optimization,conditional hyperparameter spaces,hyperparameter optimization,learning algorithms,Gaussian process,Laplace kernel,Mondrian process,random forests,scikit-learn models
AI 理解论文
溯源树
样例
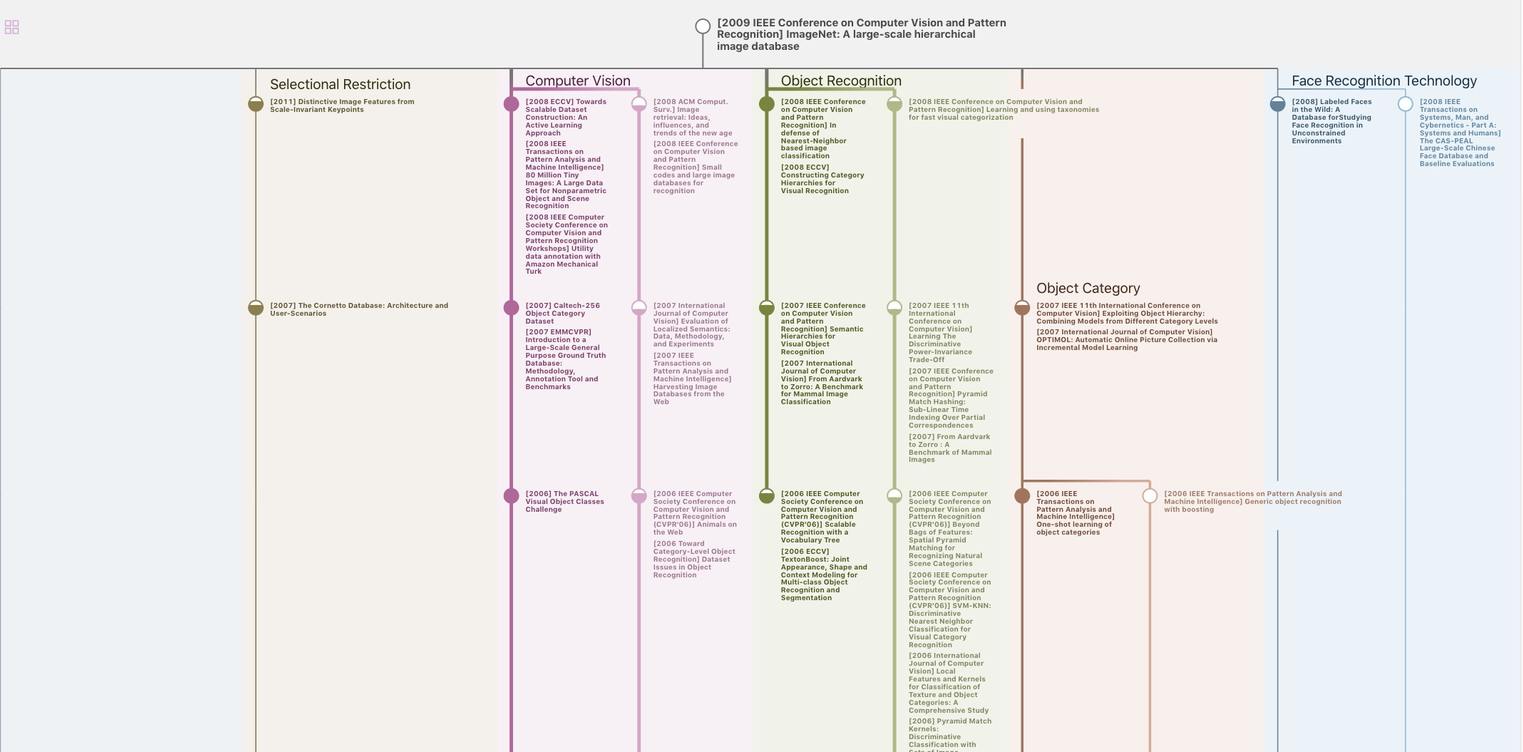
生成溯源树,研究论文发展脉络
Chat Paper
正在生成论文摘要