Share a pie?: Privacy-Preserving Knowledge Base Export through Count-min Sketches.
CODASPY(2017)
摘要
Knowledge base (KB) sharing among parties has been proven to be beneficial in several scenarios. However such sharing can arise considerable privacy concerns depending on the sensitivity of the information stored in each party's KB. In this paper, we focus on the problem of exporting a (part of a) KB of a party towards a receiving one. We introduce a novel solution that enables parties to export data in a privacy-preserving fashion, based on a probabilistic data structure, namely the \\emph{count-min sketch}. With this data structure, KBs can be exported in the form of key-value stores and inserted into a set of count-min sketches, where keys can be sensitive and values are counters. Count-min sketches can be tuned to achieve a given key collision probability, which enables a party to deny having certain keys in its own KB, and thus to preserve its privacy. We also introduce a metric, the γ-deniability (novel for count-min sketches), to measure the privacy level obtainable with a count-min sketch. Furthermore, since the value associated to a key can expose to linkage attacks, noise can be added to a count-min sketch to ensure controlled error on retrieved values. Key collisions and noise alter the values contained in the exported KB, and can affect negatively the accuracy of a computation performed on the exported KB. We explore the tradeoff between privacy preservation and computation accuracy by experimental evaluations in two scenarios related to malware detection.
更多查看译文
关键词
Information sharing, knowledge base export, privacy metric, count-min sketches
AI 理解论文
溯源树
样例
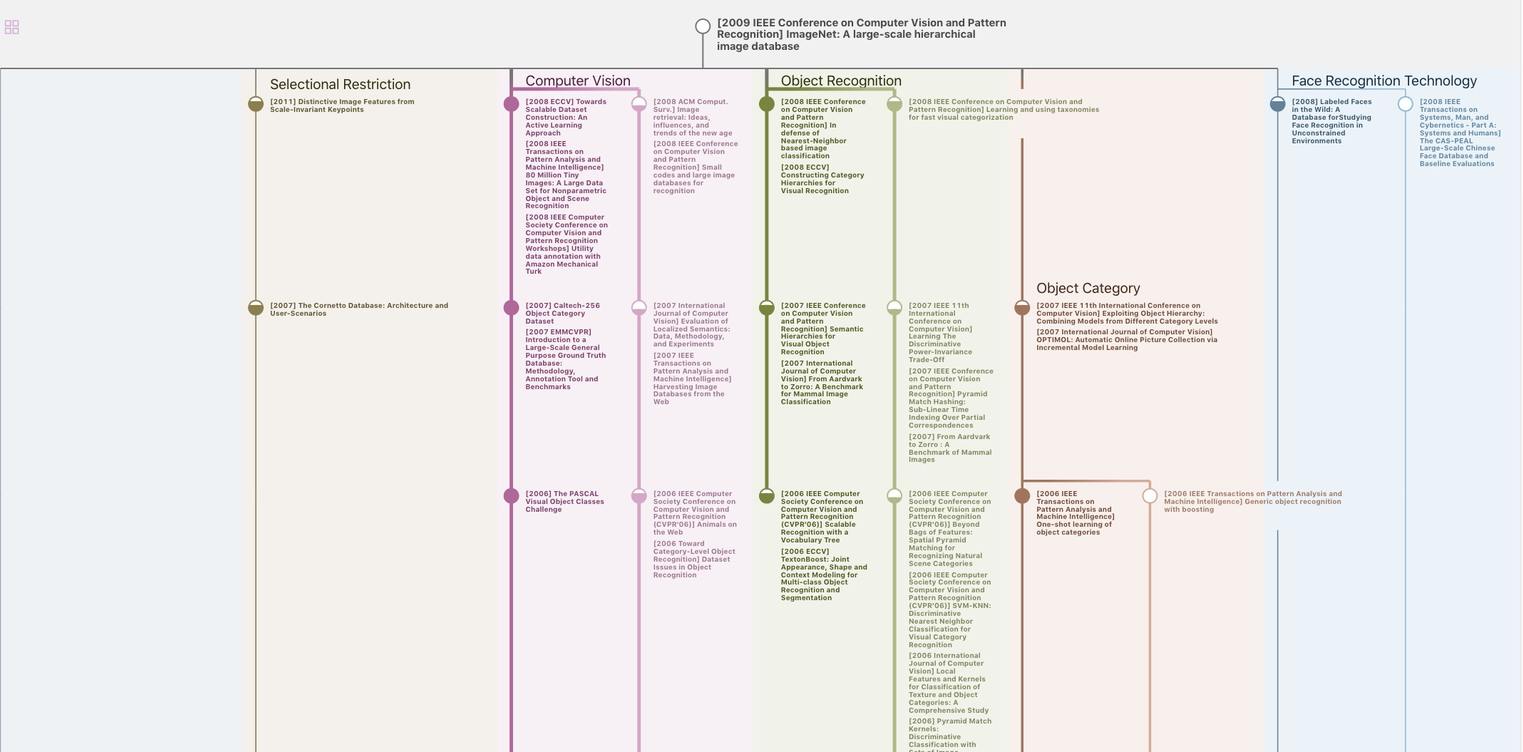
生成溯源树,研究论文发展脉络
Chat Paper
正在生成论文摘要