Hybrid spiking-based multi-layered self-learning neuromorphic system based on memristor crossbar arrays.
DATE(2017)
摘要
Neuromorphic computing systems are under heavy investigation as a potential substitute for the traditional von Neumann systems in high-speed low-power applications. Recently, memristor crossbar arrays were utilized in realizing spiking-based neuromorphic system, where memristor conductance values correspond to synaptic weights. Most of these systems are composed of a single crossbar layer, in which system training is done off-chip, using computer based simulations, then the trained weights are pre-programmed to the memristor crossbar array. However, multi-layered, on-chip trained systems become crucial for handling massive amount of data and to overcome the resistance shift that occurs to memristors overtime. In this work, we propose a spiking-based multi-layered neuromorphic computing system capable of online training. The system performance is evaluated using three different datasets showing improved results versus previous work. In addition, studying the system accuracy versus memristor resistance shift shows promising results.
更多查看译文
关键词
hybrid spiking-based multilayered self-learning neuromorphic system,memristor crossbar arrays,von Neumann systems,high-speed low-power applications,memristor conductance values,synaptic weights,single crossbar layer,computer based simulations,multilayered on-chip trained systems,spiking-based multilayered neuromorphic computing system,online training
AI 理解论文
溯源树
样例
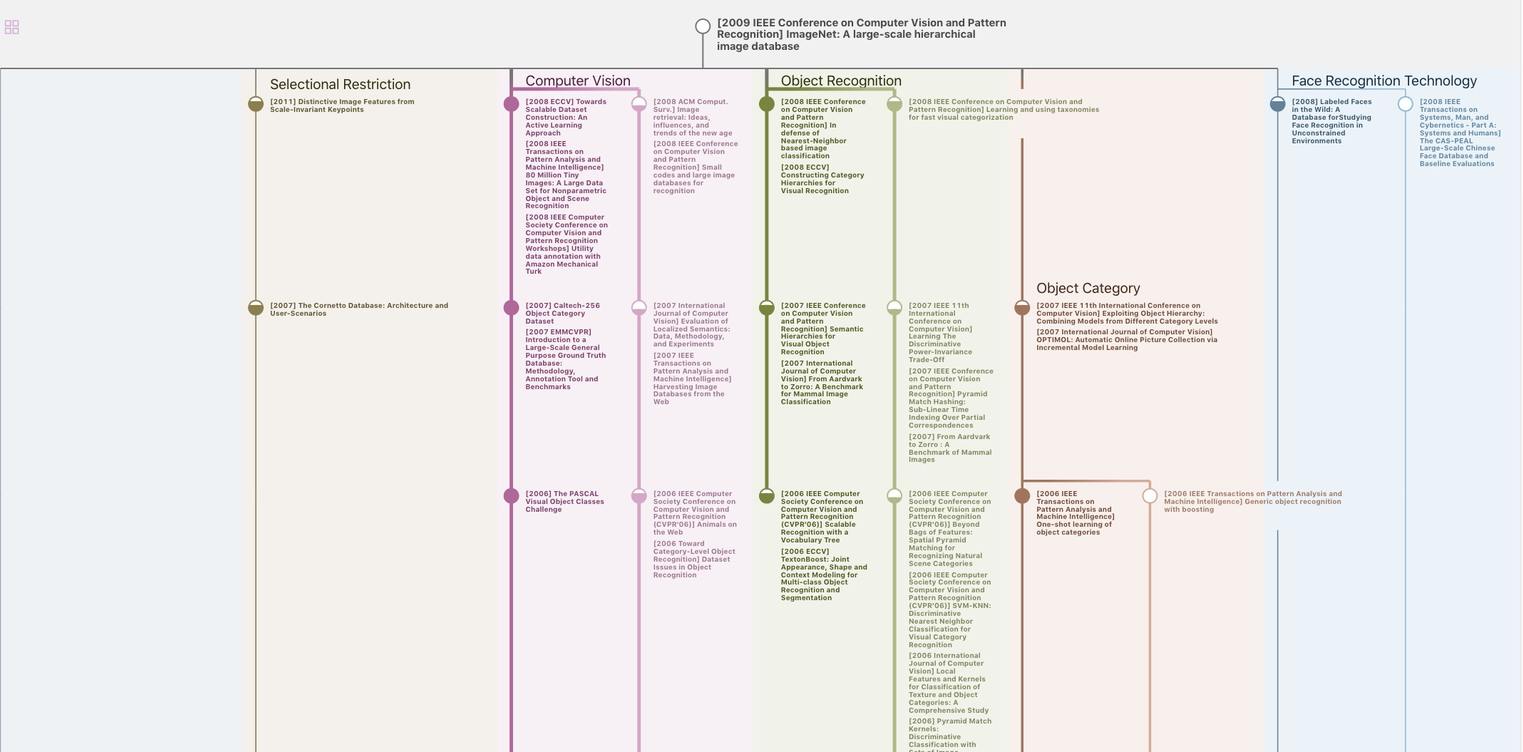
生成溯源树,研究论文发展脉络
Chat Paper
正在生成论文摘要