OFSF-BC: Online feature selection framework for binary classification
2016 IEEE/ACS 13th International Conference of Computer Systems and Applications (AICCSA)(2016)
摘要
Feature selection is a very important technique in machine learning and pattern classification. Feature selection studies using batch learning methods are inefficient when handling big data in real world, especially when data arrives sequentially. Online Feature Selection is a new paradigm which is more efficient than batch feature selection methods but it still very challenging in large-scale ultra-high dimensional sparse domains. In this paper, we propose a framework of online feature selection for binary classification exploiting first-order and second-order information. This framework is designed to assume an efficient and scalable online feature selection process. In the experimental studies, we adopt first-order and second-order online learning based online feature selection methods FOOL-OFS and SOOL-OFS. We conduct extensive experiments to evaluate the learning accuracy and time cost of different algorithms on several benchmarks and some real-world datasets.
更多查看译文
关键词
feature selection,first-order online learning,second-order online learning,binary classification
AI 理解论文
溯源树
样例
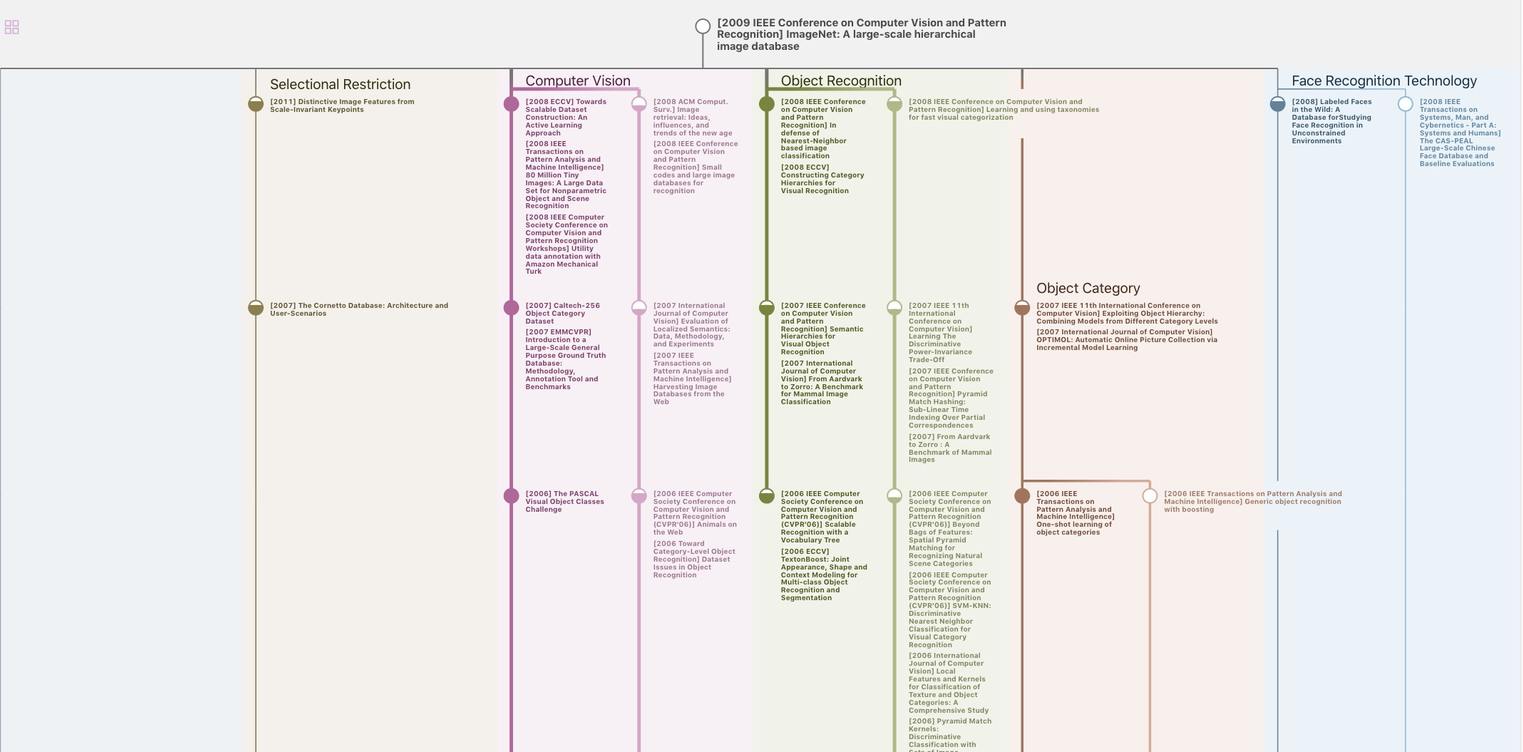
生成溯源树,研究论文发展脉络
Chat Paper
正在生成论文摘要